Multi-Decoder DPRNN: High Accuracy Source Counting and Separation
arxiv(2020)
摘要
ChampaignABSTRACTWe propose an end-to-end trainable approach to single-channel speech separation with unknown number of speakers.Our approach extends the MulCat source separation backbonewith additional output heads: a count-head to infer the num-ber of speakers, and decoder-heads for reconstructing theoriginal signals. Beyond the model, we also propose a metricon how to evaluate source separation with variable numberof speakers. Specifically, we cleared up the issue on how toevaluate the quality when the ground-truth hasmore or lessspeakersthan the ones predicted by the model. We evaluateour approach on the WSJ0-mix datasets, with mixtures upto five speakers. We demonstrate that our approach outper-forms state-of-the-art in counting the number of speakers andremains competitive in quality of reconstructed signals.
更多查看译文
关键词
high accuracy source counting,multi-decoder
AI 理解论文
溯源树
样例
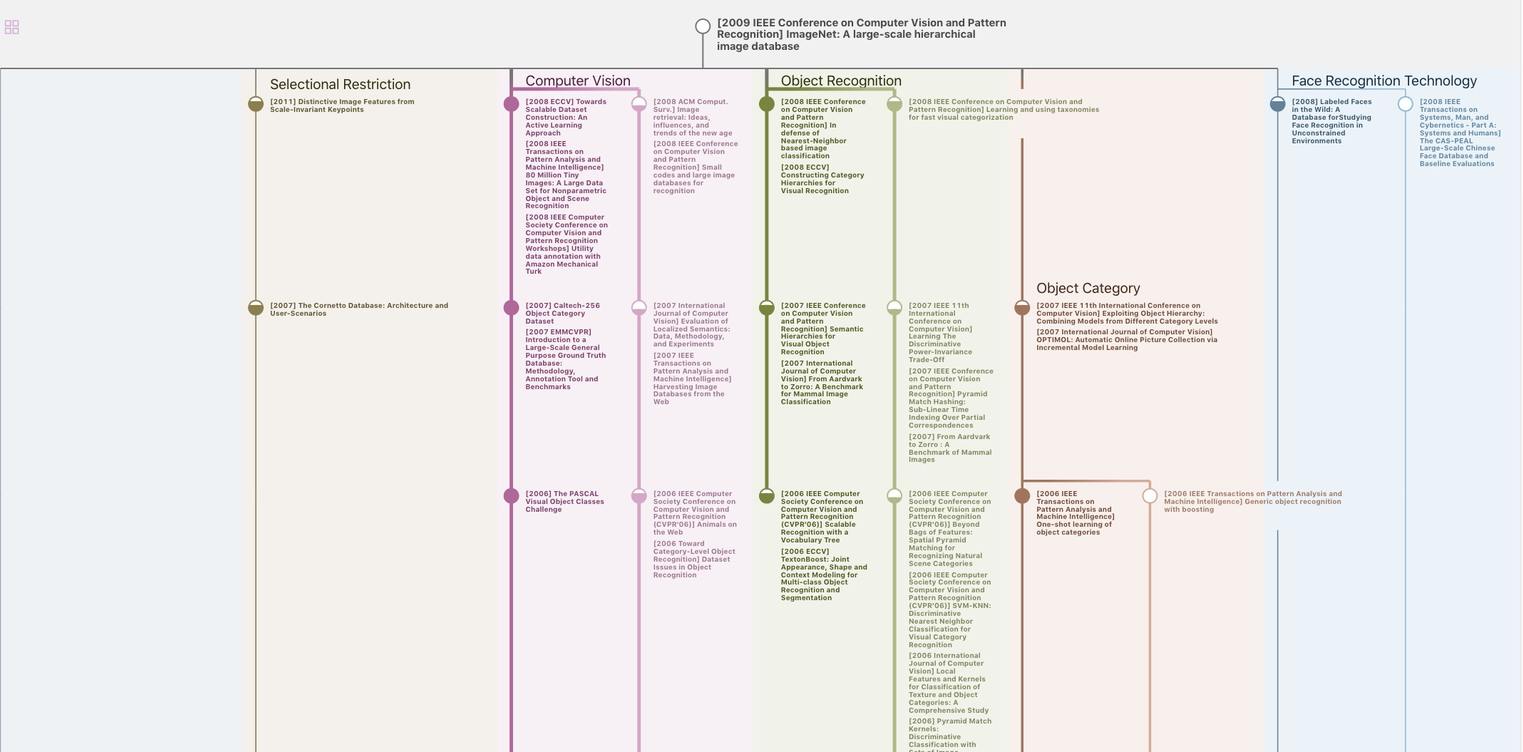
生成溯源树,研究论文发展脉络
Chat Paper
正在生成论文摘要