SemiNLL: A Framework of Noisy-Label Learning by Semi-Supervised Learning
arxiv(2020)
摘要
Deep learning with noisy labels is a challenging task. Recent prominent methods that build on a specific sample selection (SS) strategy and a specific semi-supervised learning (SSL) model achieved state-of-the-art performance. Intuitively, better performance could be achieved if stronger SS strategies and SSL models are employed. Following this intuition, one might easily derive various effective noisy-label learning methods using different combinations of SS strategies and SSL models, which is, however, reinventing the wheel in essence. To prevent this problem, we propose SemiNLL, a versatile framework that combines SS strategies and SSL models in an end-to-end manner. Our framework can absorb various SS strategies and SSL backbones, utilizing their power to achieve promising performance. We also instantiate our framework with different combinations, which set the new state of the art on benchmark-simulated and real-world datasets with noisy labels.
更多查看译文
关键词
learning,noisy-label,semi-supervised
AI 理解论文
溯源树
样例
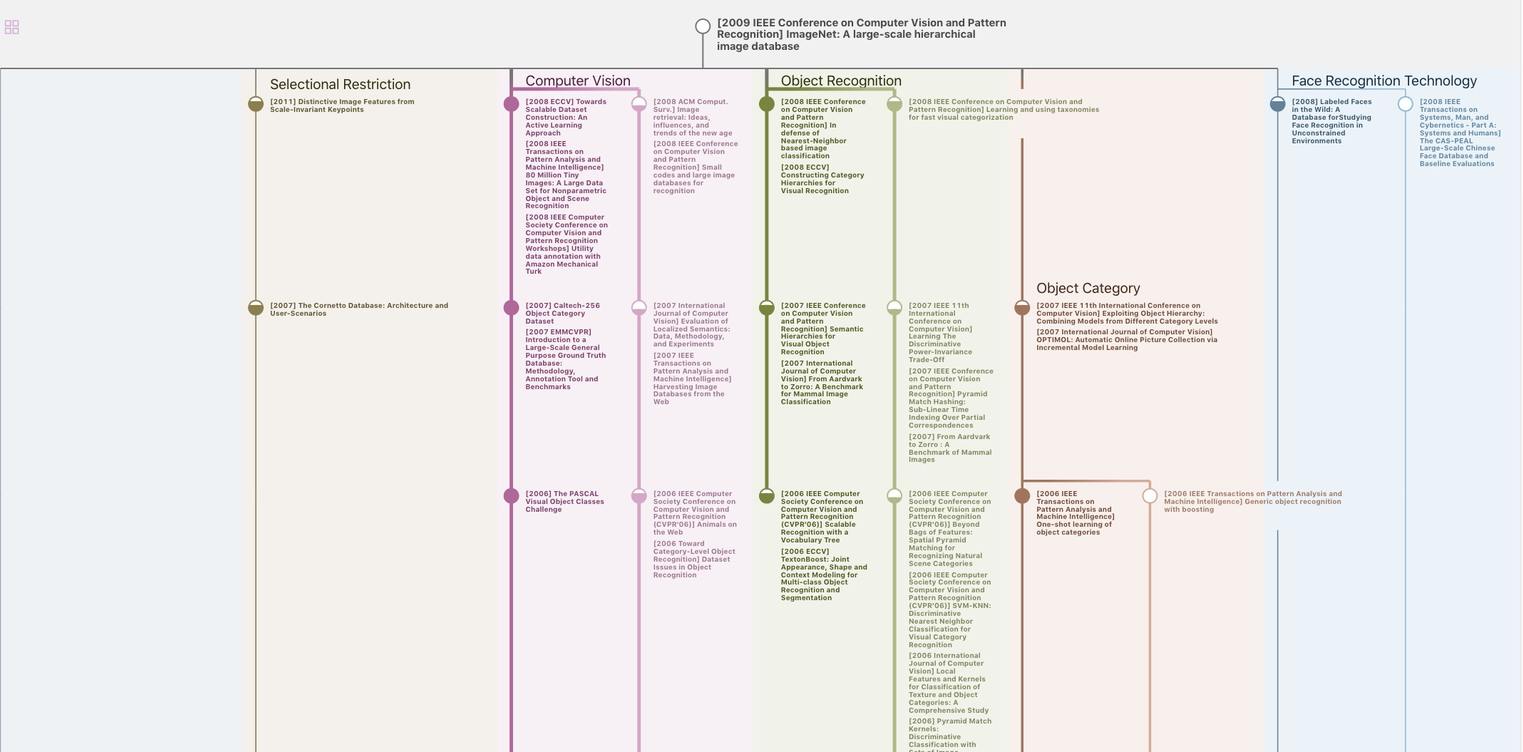
生成溯源树,研究论文发展脉络
Chat Paper
正在生成论文摘要