Minimax Regret Optimisation For Robust Planning In Uncertain Markov Decision Processes
THIRTY-FIFTH AAAI CONFERENCE ON ARTIFICIAL INTELLIGENCE, THIRTY-THIRD CONFERENCE ON INNOVATIVE APPLICATIONS OF ARTIFICIAL INTELLIGENCE AND THE ELEVENTH SYMPOSIUM ON EDUCATIONAL ADVANCES IN ARTIFICIAL INTELLIGENCE(2021)
摘要
The parameters for a Markov Decision Process (MDP) often cannot be specified exactly. Uncertain MDPs (UMDPs) capture this model ambiguity by defining sets which the parameters belong to Minimax regret has been proposed as an objective for planning in UMDPs to find robust policies which are not overly conservative. In this work, we focus on planning for Stochastic Shortest Path (SSP) UMDPs with uncertain cost and transition functions. We introduce a Bellman equation to compute the regret for a policy. We propose a dynamic programming algorithm that utilises the regret Bellman equation, and show that it optimises minimax regret exactly for UMDPs with independent uncertainties. For coupled uncertainties, we extend our approach to use options to enable a trade off between computation and solution quality. We evaluate our approach on both synthetic and real-world domains, showing that it significantly outperforms existing baselines.
更多查看译文
关键词
robust planning,uncertain markov
AI 理解论文
溯源树
样例
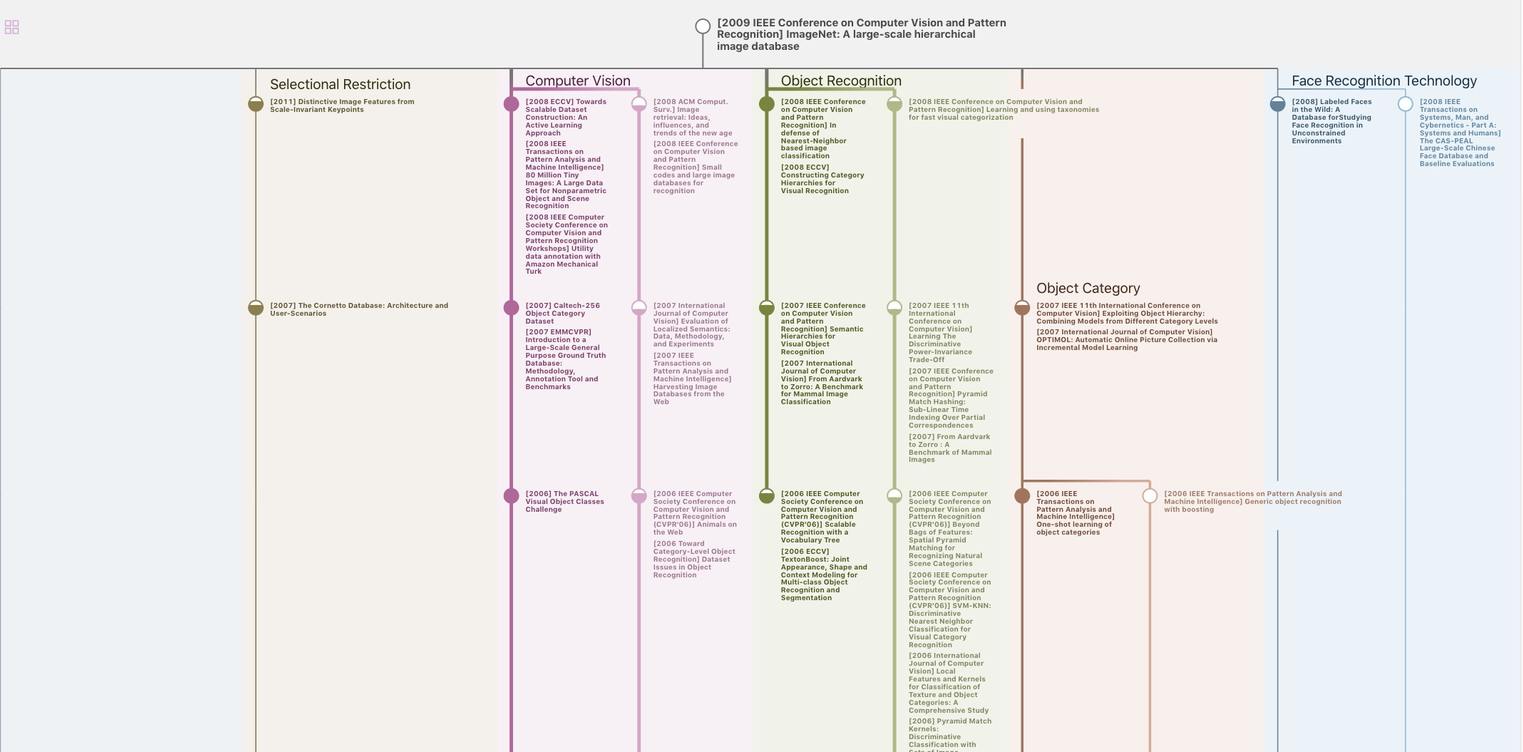
生成溯源树,研究论文发展脉络
Chat Paper
正在生成论文摘要