Cortex: Harnessing Correlations to Boost Query Performance
arxiv(2020)
摘要
Databases employ indexes to filter out irrelevant records, which reduces scan overhead and speeds up query execution. However, this optimization is only available to queries that filter on the indexed attribute. To extend these speedups to queries on other attributes, database systems have turned to secondary and multi-dimensional indexes. Unfortunately, these approaches are restrictive: secondary indexes have a large memory footprint and can only speed up queries that access a small number of records, and multi-dimensional indexes cannot scale to more than a handful of columns. We present Cortex, an approach that takes advantage of correlations to extend the reach of primary indexes to more attributes. Unlike prior work, Cortex can adapt itself to any existing primary index, whether single or multi-dimensional, to harness a broad variety of correlations, such as those that exist between more than two attributes or have a large number of outliers. We demonstrate that on real datasets exhibiting these diverse types of correlations, Cortex matches or outperforms traditional secondary indexes with $5\times$ less space, and it is $2-8\times$ faster than existing approaches to indexing correlations.
更多查看译文
关键词
query performance,harnessing correlations
AI 理解论文
溯源树
样例
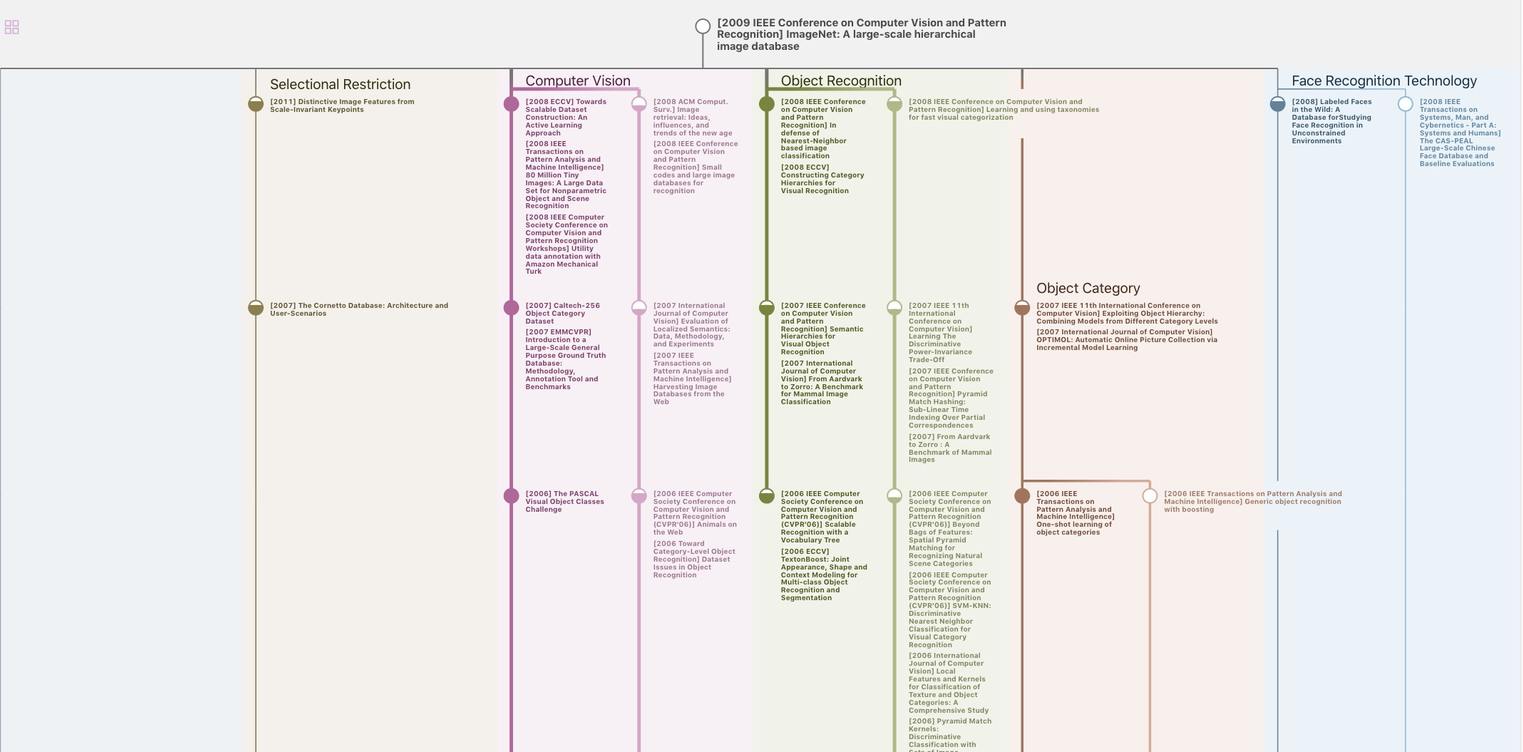
生成溯源树,研究论文发展脉络
Chat Paper
正在生成论文摘要