Upper Confidence Bounds for Combining Stochastic Bandits
arxiv(2020)
摘要
We provide a simple method to combine stochastic bandit algorithms. Our approach is based on a "meta-UCB" procedure that treats each of $N$ individual bandit algorithms as arms in a higher-level $N$-armed bandit problem that we solve with a variant of the classic UCB algorithm. Our final regret depends only on the regret of the base algorithm with the best regret in hindsight. This approach provides an easy and intuitive alternative strategy to the CORRAL algorithm for adversarial bandits, without requiring the stability conditions imposed by CORRAL on the base algorithms. Our results match lower bounds in several settings, and we provide empirical validation of our algorithm on misspecified linear bandit and model selection problems.
更多查看译文
关键词
stochastic bandits,upper confidence bounds
AI 理解论文
溯源树
样例
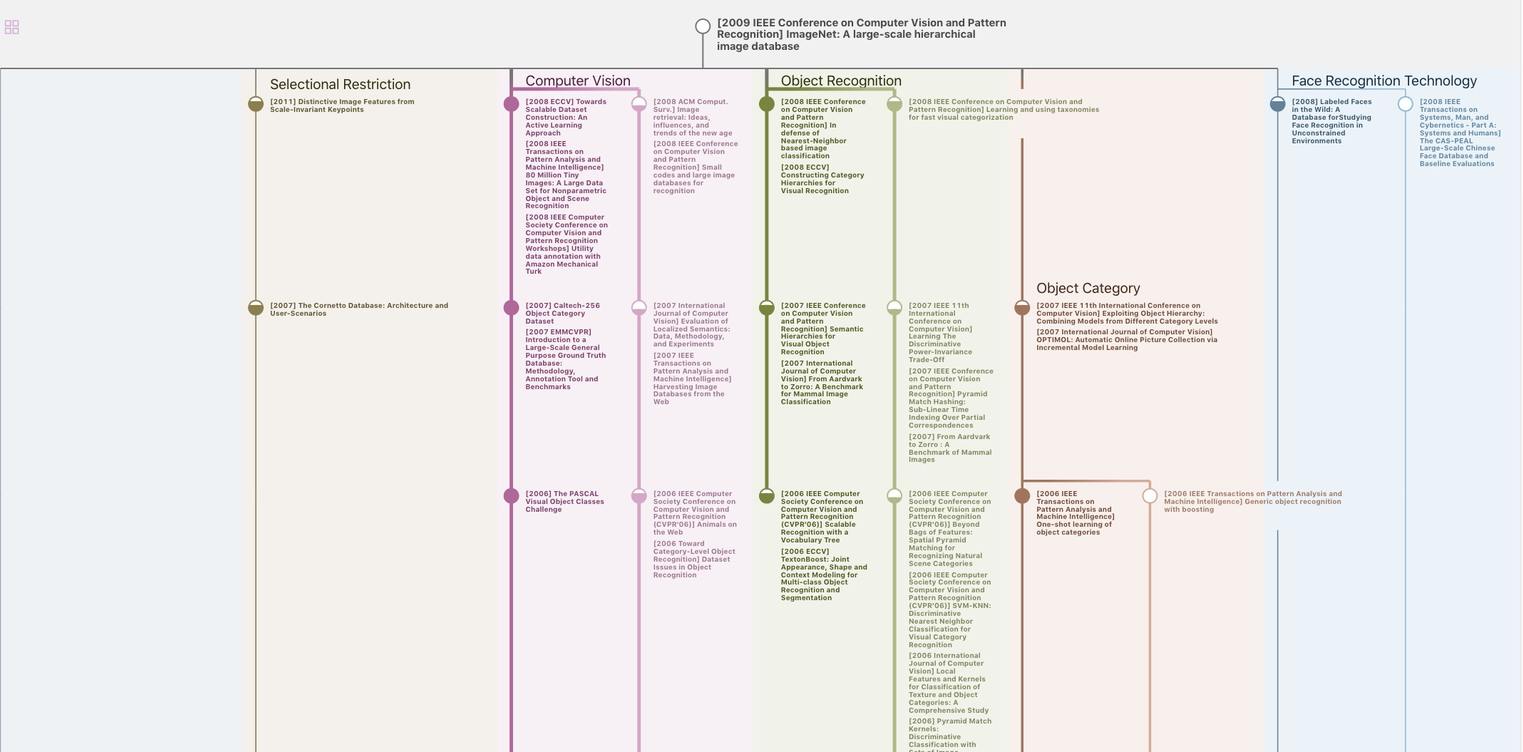
生成溯源树,研究论文发展脉络
Chat Paper
正在生成论文摘要