Counterfeit Anomaly Using Generative Adversarial Network for Anomaly Detection
IEEE ACCESS(2020)
摘要
Anomaly detection aims to detect anomaly with only normal data available for training. It attracts considerable attentions in the medical domain, as normal data is relatively easy to obtain but it is rather difficult to have abnormal data especially for some rare diseases, making training a standard classifier challenging or even impossible. Recently, generative adversarial networks (GANs) become prevalent for anomaly detection and most existing GAN-based methods detect outliers by the reconstruction error. In this paper, we propose a novel framework called adGAN for anomaly detection using GAN. Unlike existing GAN-based methods, adGAN is a discriminative model, which uses the fake data generated from GAN as an abnormal class, and then learns a boundary between normal data and simulated abnormal data. Thus it is able to output the anomaly scores directly similar as one-class SVM (OCSVM), without any reconstruction process. We explicitly design adGAN with two key elements, i.e., fake pool generation and concentration loss. The fake pool is created by incrementally collecting the fake data produced by intermediate-state GAN, which are likely surrounding the normal data distribution. The concentration loss is innovatively introduced to penalize large standard deviations of discriminator outputs for normal data, aiming to make the distribution of normal data more compact and more likely to be separated from the distribution of the potential abnormal data. The trained discriminator is finally used as an anomaly detector. We evaluated adGAN on three datasets, including ab-MNIST for synthetic anomaly detection, the ISIC'2016 for skin lesion detection, and the BraTS'2017 for brain lesion detection. The extensive experiments demonstrate that adGAN is consistently superior to its competitors on all three datasets.
更多查看译文
关键词
Anomaly detection,Gallium nitride,Generators,Training,Image reconstruction,Biomedical imaging,Generative adversarial networks,Anomaly detection,concentration loss,fake pool,GAN
AI 理解论文
溯源树
样例
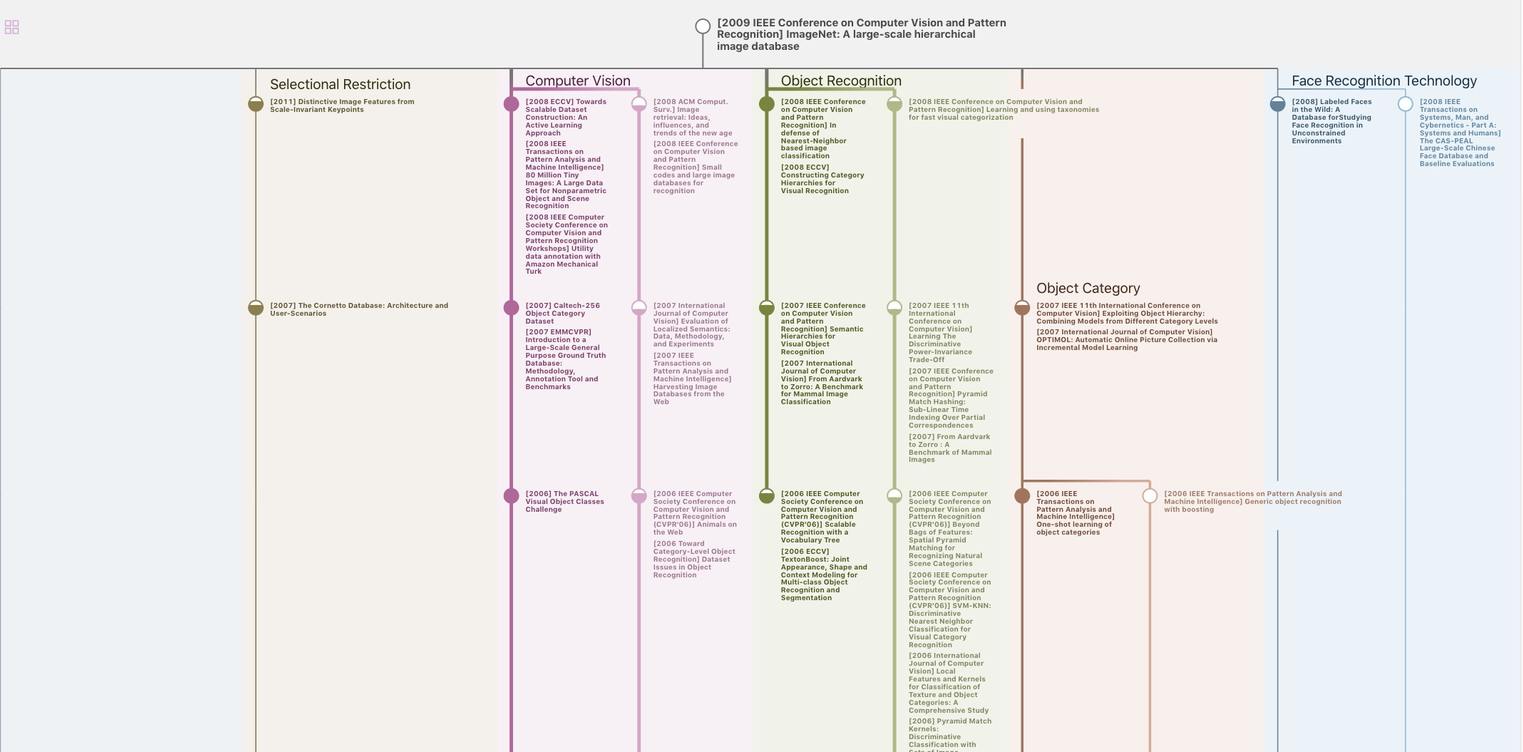
生成溯源树,研究论文发展脉络
Chat Paper
正在生成论文摘要