Neuro-Inspired-in-Memory Computing Using Charge-Trapping MemTransistor on Germanium as Synaptic Device
IEEE Transactions on Electron Devices(2020)
摘要
In this work, we fabricated charge-trapping MemTransistors (CTMTs) on a germanium (Ge) substrate with a single-charge-trapping-layer gate-stack or a double-charge-trapping-layer gate-stack. We first constructed the energy band diagram of two gate stacks using transmission electron microscope (TEM) images and by X-ray photoelectron spectroscopy analysis. We deposited Al2O3 as a tunneling layer and a barrier layer using an atomic layer deposition (ALD) system while depositing HfO2 by ALD as the charge-trapping layer whose conduction band offset with respect to Al2O3 is 1.74 eV. Next, we demonstrated the memory characteristics of the CTMTs. By implementing the double-charge-trapping-layer gate-stack on the CTMT, we were able to enlarge the memory windows by 372 mV, improve the retention by 2.7%, and reduce the read disturbance. Furthermore, we demonstrated the synaptic device characteristics of the CTMTs. With the optimization of pulse schemes, we reduced the nonlinearity of potentiation ($\alpha _{\text {p}}$ ) and depression ($\alpha _{\text {d}}$ ) from 8.62 and -6.01 to 0.71 and 0.01, respectively, enlarged the ON/OFF ratio from 10.2 to 66.2, and increased the recognition accuracy from 24.5% to 82.1% simultaneously. With the implementation of the double-charge-trapping-layer gate-stack, we could further enlarge the ON/OFF ratio to 75.3 and increase the recognition accuracy to 86.5% simultaneously.
更多查看译文
关键词
Analog memories,artificial intelligence (AI),dielectric materials,germanium (Ge),MOSFETs,multilayer perceptrons (MLPs),neural network hardware,pattern recognition,semiconductor memories
AI 理解论文
溯源树
样例
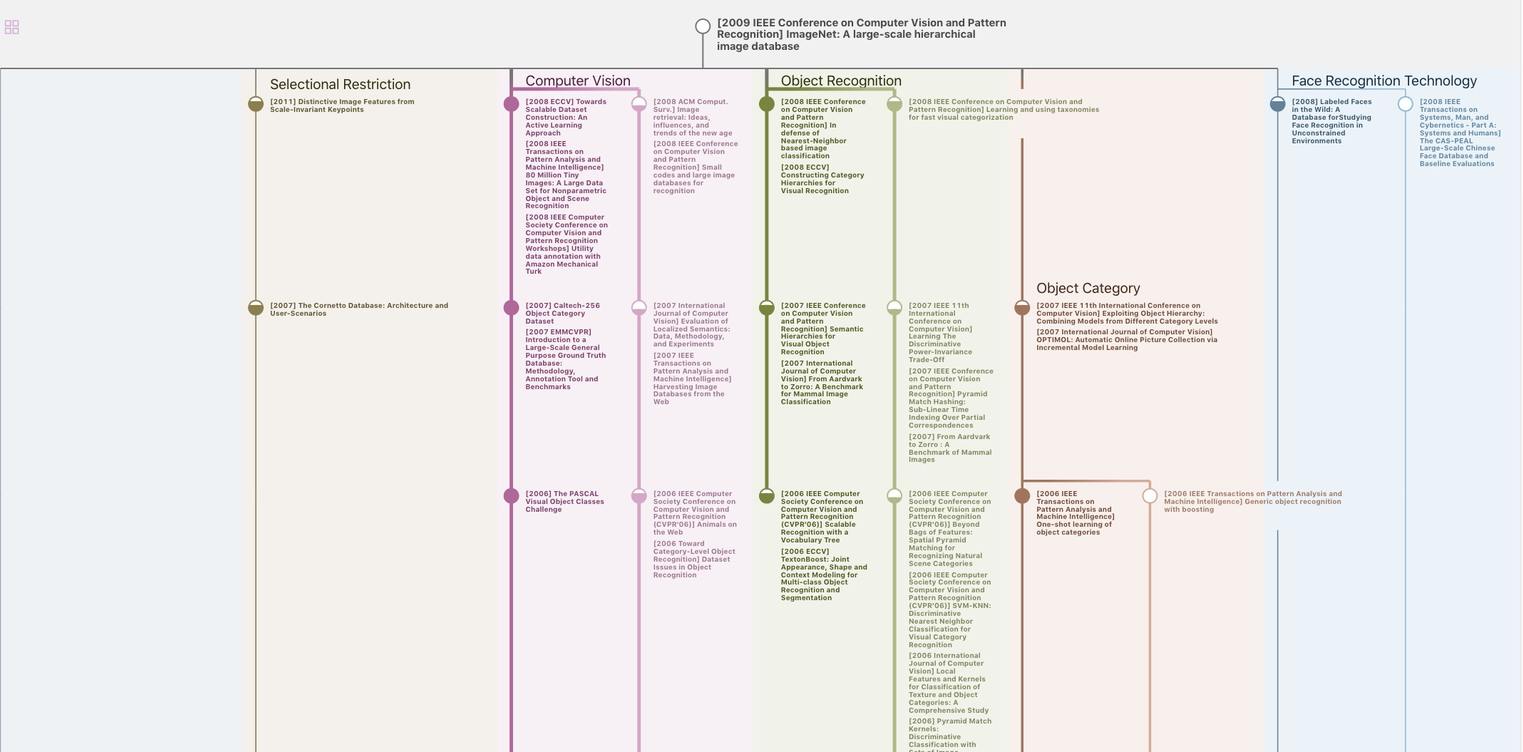
生成溯源树,研究论文发展脉络
Chat Paper
正在生成论文摘要