Optimal Emg Placement For A Robotic Prosthesis Controller With Sequential, Adaptive Functional Estimation (Safe)
ANNALS OF APPLIED STATISTICS(2020)
摘要
Robotic hand prostheses require a controller to decode muscle contraction information, such as electromyogram (EMG) signals, into the user's desired hand movement. State-of-the-art decoders demand extensive training, require data from a large number of EMG sensors and are prone to poor predictions. Biomechanical models of a single movement degree-of-freedom tell us that relatively few muscles, and, hence, fewer EMG sensors are needed to predict movement. We propose a novel decoder based on a dynamic, functional linear model with velocity or acceleration as its response and the recent past EMG signals as functional covariates. The effect of each EMG signal varies with the recent position to account for biomechanical features of hand movement, increasing the predictive capability of a single EMG signal compared to existing decoders. The effects are estimated with a multistage, adaptive estimation procedure that we call Sequential Adaptive Functional Estimation (SAFE). Starting with 16 potential EMG sensors, our method correctly identifies the few EMG signals that are known to be important for an able-bodied subject. Furthermore, the estimated effects are interpretable and can significantly improve understanding and development of robotic hand prostheses.
更多查看译文
关键词
Electromyography signal, varying functional regression, functional variable selection, adaptive group LASSO, correlated functional predictors, sequential adaptive functional estimation
AI 理解论文
溯源树
样例
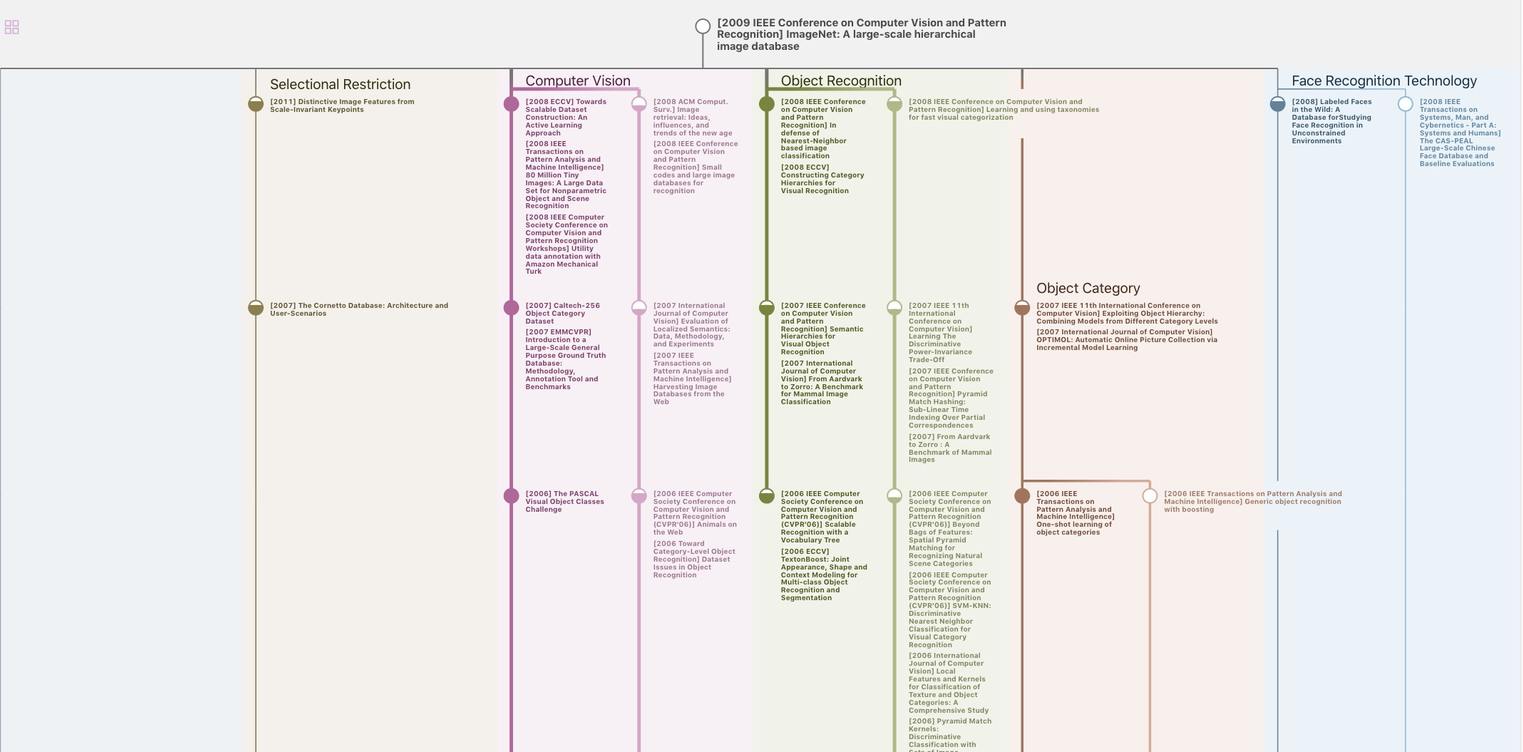
生成溯源树,研究论文发展脉络
Chat Paper
正在生成论文摘要