Weakly Supervised Deep Learning for Breast Cancer Segmentation with Coarse Annotations
medical image computing and computer-assisted intervention(2020)
摘要
Cancer lesion segmentation plays a vital role in breast cancer diagnosis and treatment planning. As creating labels for large medical image datasets can be time-consuming, laborious and error prone, a framework is proposed in this paper by using coarse annotations generated from boundary scribbles for training deep convolutional neural networks. These coarse annotations include locations of lesions but are lack of accurate information about boundaries. To mitigate the negative impact of annotation errors, we propose an adaptive weighted constrained loss that can change the weight of the task-specific penalty term according to the learning process. To impose further supervision about the boundaries, uncertainty-based boundary maps are generated, which can provide better descriptions for the blurry boundaries. Validation on a dataset containing 154 MRI scans has shown an average Dice coefficient of \(82.25\%\), which is comparable to results from fine annotations, demonstrating the efficacy of the proposed approach.
更多查看译文
关键词
weakly supervised deep learning,breast cancer segmentation,breast cancer,deep learning
AI 理解论文
溯源树
样例
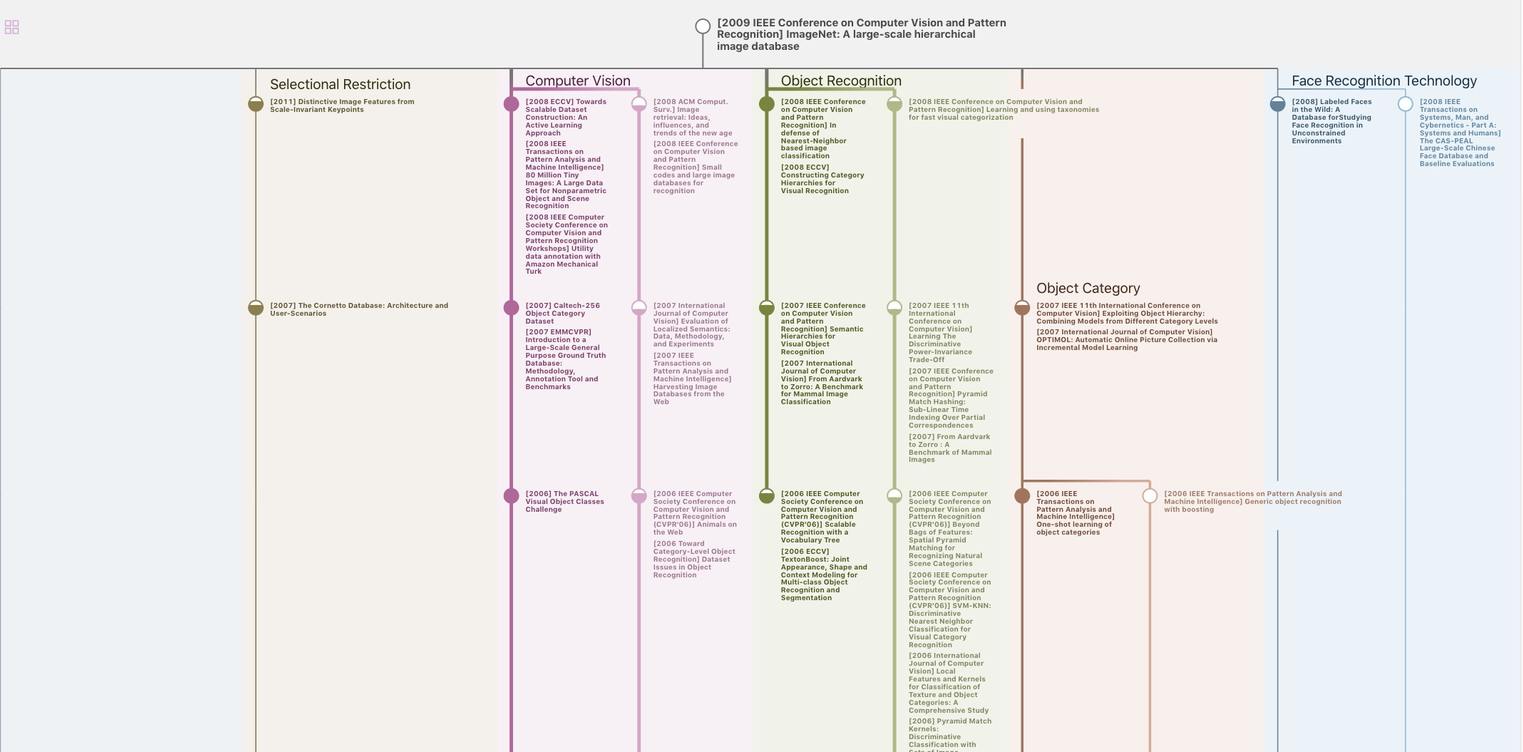
生成溯源树,研究论文发展脉络
Chat Paper
正在生成论文摘要