Learning Link Schedules in Self-Backhauled Millimeter Wave Cellular Networks
IEEE Transactions on Wireless Communications(2020)
摘要
Multihop self-backhauling is a key enabling technology for millimeter wave cellular deployments. We consider the multihop link scheduling problem with the objective of minimizing the end-to-end delay experienced by a typical packet. This is a complex problem, and so we model the system as a network of queues and formulate it as a Markov decision process over a continuous action space. This allows us to leverage the deep deterministic policy gradient algorithm from reinforcement learning to learn the delay minimizing scheduling policy under two scenarios: 1) an ideal setup where a centralized scheduler performs all per slot scheduling decisions and has full instantaneous knowledge of network state and 2) a centralized scheduler, but where network state feedback and scheduling decisions are limited to once per frame, which is many slots. For the second scenario, we model the scheduler with a recurrent neural network to capture the evolution of the network state over the frame. Detailed system-level simulations show that for the more realistic second scenario, the delay experienced by the 5
th
percentile packets under backpressure based scheduling and max-min scheduling can be up to 230% and 260%, respectively more than that under the proposed scheduler.
更多查看译文
关键词
Integrated access and backhaul (IAB),millimeter wave (mmWave) communication,link scheduling,deep reinforcement learning
AI 理解论文
溯源树
样例
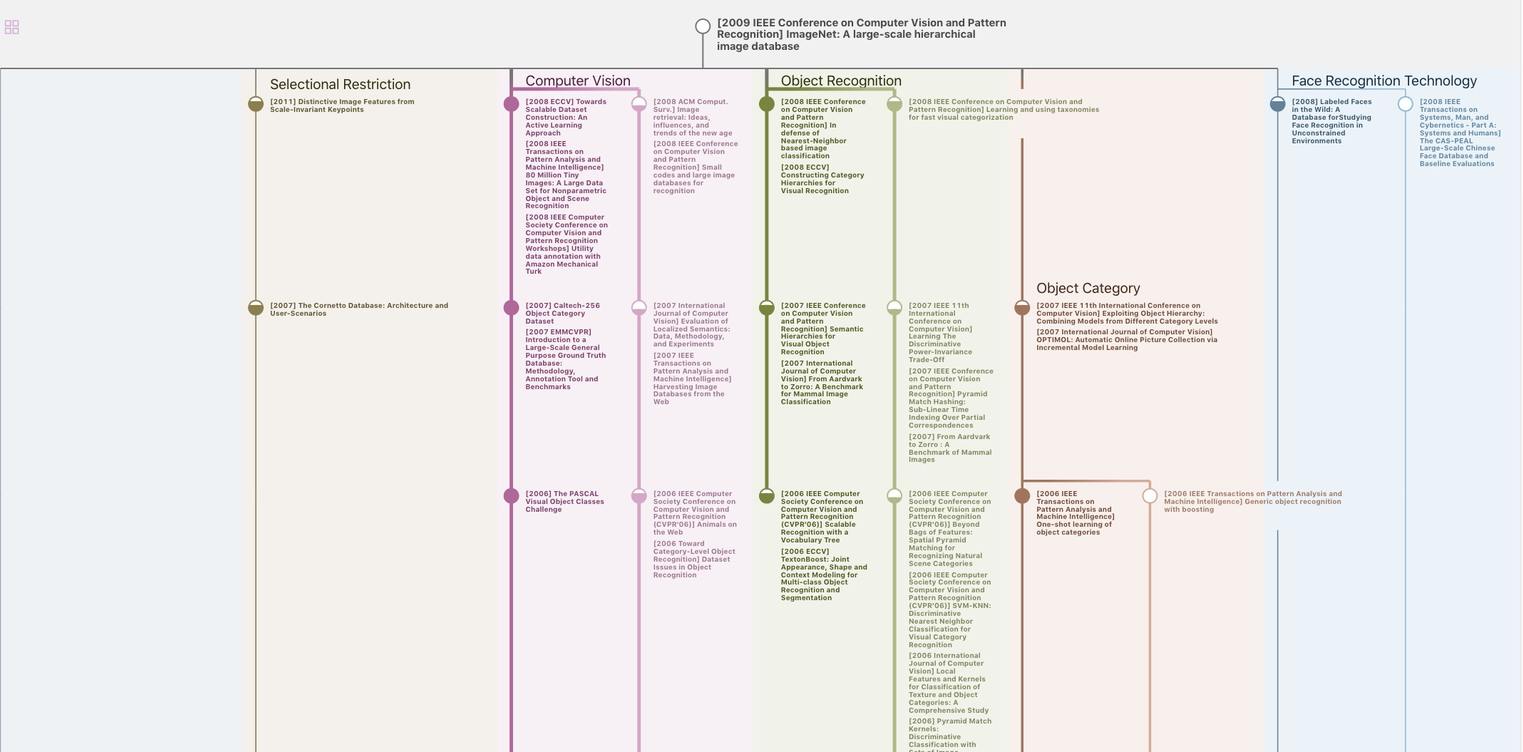
生成溯源树,研究论文发展脉络
Chat Paper
正在生成论文摘要