LSFB: A Low-cost and Scalable Framework for Building Large-Scale Localization Benchmark
2020 IEEE International Symposium on Mixed and Augmented Reality Adjunct (ISMAR-Adjunct)(2020)
摘要
With the rapid development of mobile sensor, network infrastructure and cloud computing, the scale of AR application scenario is expanding from small or medium scale to large-scale environments. Localization in the large-scale environment is a critical demand for the AR applications. Most of the commonly used localization techniques require quite a number of data with groundtruth localization for algorithm benchmarking or model training. The existed groundtruth collection methods can only be used in the outdoors, or require quite expensive equipments or special deployments in the environment, thus are not scalable to large-scale environments or to massively produce a large amount of groundtruth data. In this work, we propose LSFB, a novel low-cost and scalable frame-work to build localization benchmark in large-scale environments with groundtruth poses. The key is to build an accurate HD map of the environment. For each visual-inertial sequence captured in it, the groundtruth poses are obtained by joint optimization taking both the HD map and visual-inertial constraints. The experiments demonstrate the obtained groundtruth poses are accurate enough for AR applications. We use the proposed method to collect a dataset of both mobile phones and AR glass exploring in large-scale environments, and will release the dataset as a new localization benchmark for AR.
更多查看译文
关键词
Human-centered computing,Human Computer Interaction,Interaction Paradigms,Mixed / Augmented Reality,Computing Methodologies,Artificial Intelligence,Computer Vision,Tracking
AI 理解论文
溯源树
样例
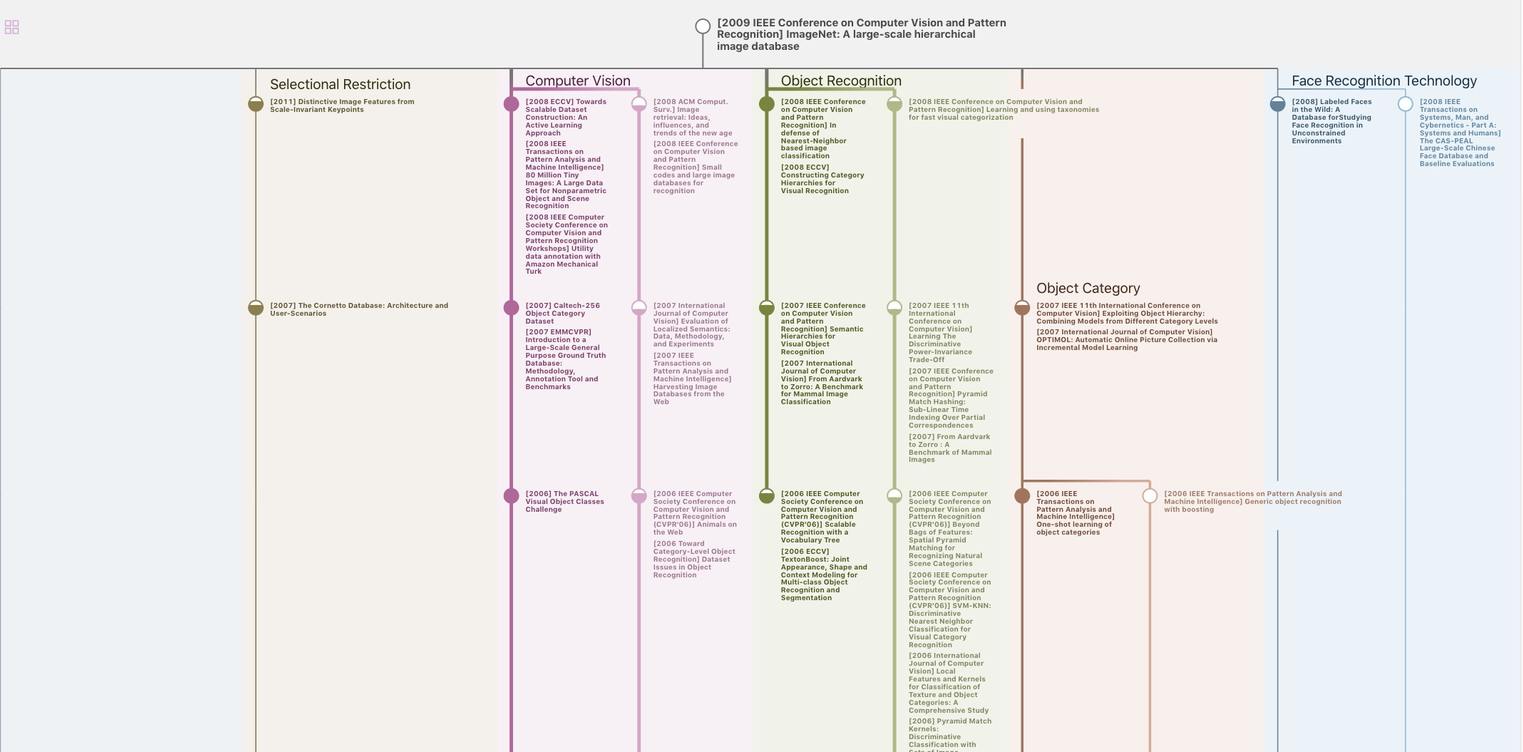
生成溯源树,研究论文发展脉络
Chat Paper
正在生成论文摘要