Deep Learning for Moving Blockage Prediction Using Real Mmwave Measurements
arXiv (Cornell University)(2021)
摘要
Millimeter wave (mmWave) communication is a key component of 5G systems and beyond. Such systems provide high bandwidth and high data rate but are sensitive to blockages. A sudden blockage in the line of sight (LOS) link leads to abrupt disconnection. Thus addressing blockage problems is essential for enhancing the reliability and latency of mmWave communication networks. In this paper, we propose a novel solution that relies only on in-band mmWave wireless measurements to proactively predict future dynamic line-of-sight (LOS) link blockages. The proposed solution utilizes deep neural networks and special patterns of received signal power, which we call pre-blockage wireless signatures, to infer future blockages. Specifically, the machine learning models attempt to predict: (i) Whether a blockage will occur in the next few seconds? (ii) At what time instance will this blockage occur? To evaluate our proposed approach, we build a mmWave communication setup with moving blockage in an indoor scenario and collect received power sequences. Simulation results on a real dataset show that blockage occurrence can be predicted with more than 85% accuracy, and the exact time instance of blockage occurrence can be obtained with less than 2 time instances (1.66s) error for prediction interval of 10 time instances (8.8s). This demonstrates the potential of the proposed solution for dynamic blockage prediction and proactive hand-off.
更多查看译文
关键词
Millimeter wave,machine learning,blockage prediction,handover
AI 理解论文
溯源树
样例
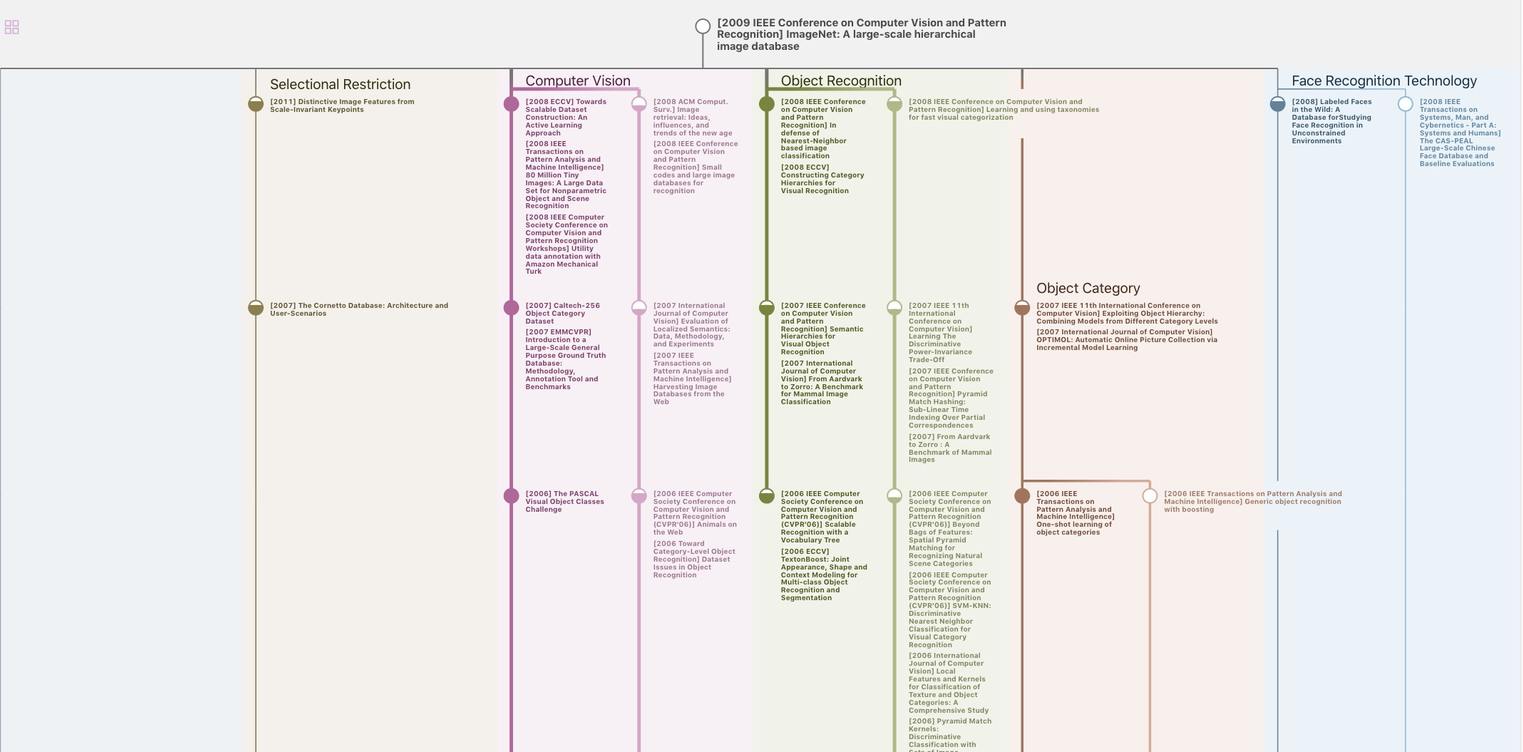
生成溯源树,研究论文发展脉络
Chat Paper
正在生成论文摘要