CPR: Classifier-Projection Regularization for Continual Learning
ICLR(2020)
摘要
We propose a general, yet simple patch that can be applied to existing
regularization-based continual learning methods called classifier-projection
regularization (CPR). Inspired by both recent results on neural networks with
wide local minima and information theory, CPR adds an additional regularization
term that maximizes the entropy of a classifier's output probability. We
demonstrate that this additional term can be interpreted as a projection of the
conditional probability given by a classifier's output to the uniform
distribution. By applying the Pythagorean theorem for KL divergence, we then
prove that this projection may (in theory) improve the performance of continual
learning methods. In our extensive experimental results, we apply CPR to
several state-of-the-art regularization-based continual learning methods and
benchmark performance on popular image recognition datasets. Our results
demonstrate that CPR indeed promotes a wide local minima and significantly
improves both accuracy and plasticity while simultaneously mitigating the
catastrophic forgetting of baseline continual learning methods. The codes and
scripts for this work are available at https://github.com/csm9493/CPR_CL.
更多查看译文
关键词
continual learning,regularization,classifier-projection
AI 理解论文
溯源树
样例
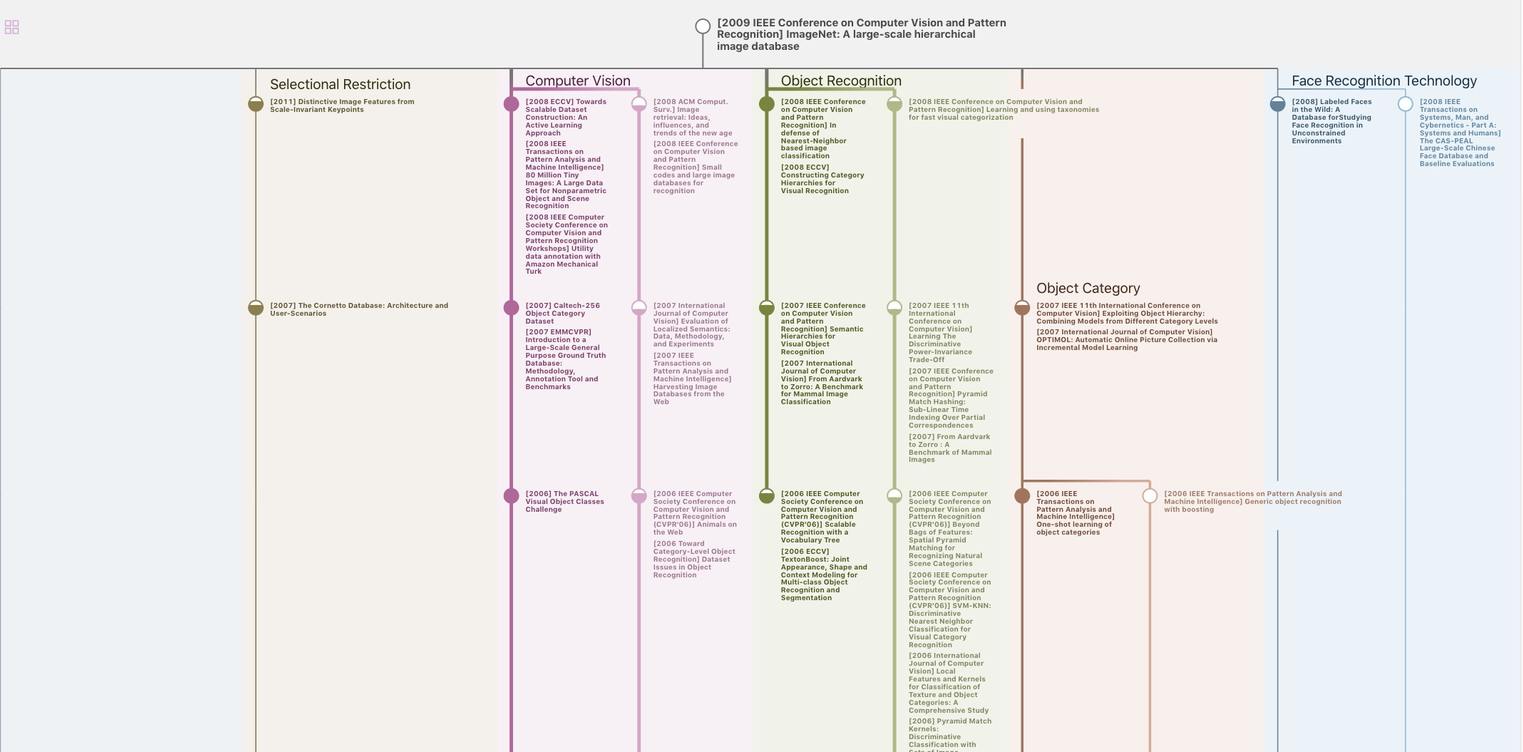
生成溯源树,研究论文发展脉络
Chat Paper
正在生成论文摘要