Semantics-Aware Privacy Risk Assessment Using Self-Learning Weight Assignment for Mobile Apps
Periodicals(2021)
摘要
AbstractMost of the existing mobile application (app) vetting mechanisms only estimate risks at a coarse-grained level by analyzing app syntax but not semantics. We propose a semantics-aware privacy risk assessment framework (SPRisk), which considers the sensitivity discrepancy of privacy-related factors at semantic level. Our framework can provide qualitative (i.e., risk level) and quantitative (i.e., risk score) assessment results, both of which help users make decisions to install an app or not. Furthermore, to find the reasonable weight distribution of each factor automatically, we exploit a self-learning weight assignment method, which is based on fuzzy clustering and knowledge dependency theory. We implement a prototype system and evaluate the effectiveness of SPRisk with 192,445 normal apps and 7,111 malicious apps. A measurement study further reveals some interesting findings, such as the privacy risk distribution of Google Play Store, the diversity of official and unofficial marketplaces, which provide insights into understanding the seriousness of privacy threat in the Android ecosystem.
更多查看译文
关键词
Android, semantics-aware, self-learning weight assignment, privacy risk assessment
AI 理解论文
溯源树
样例
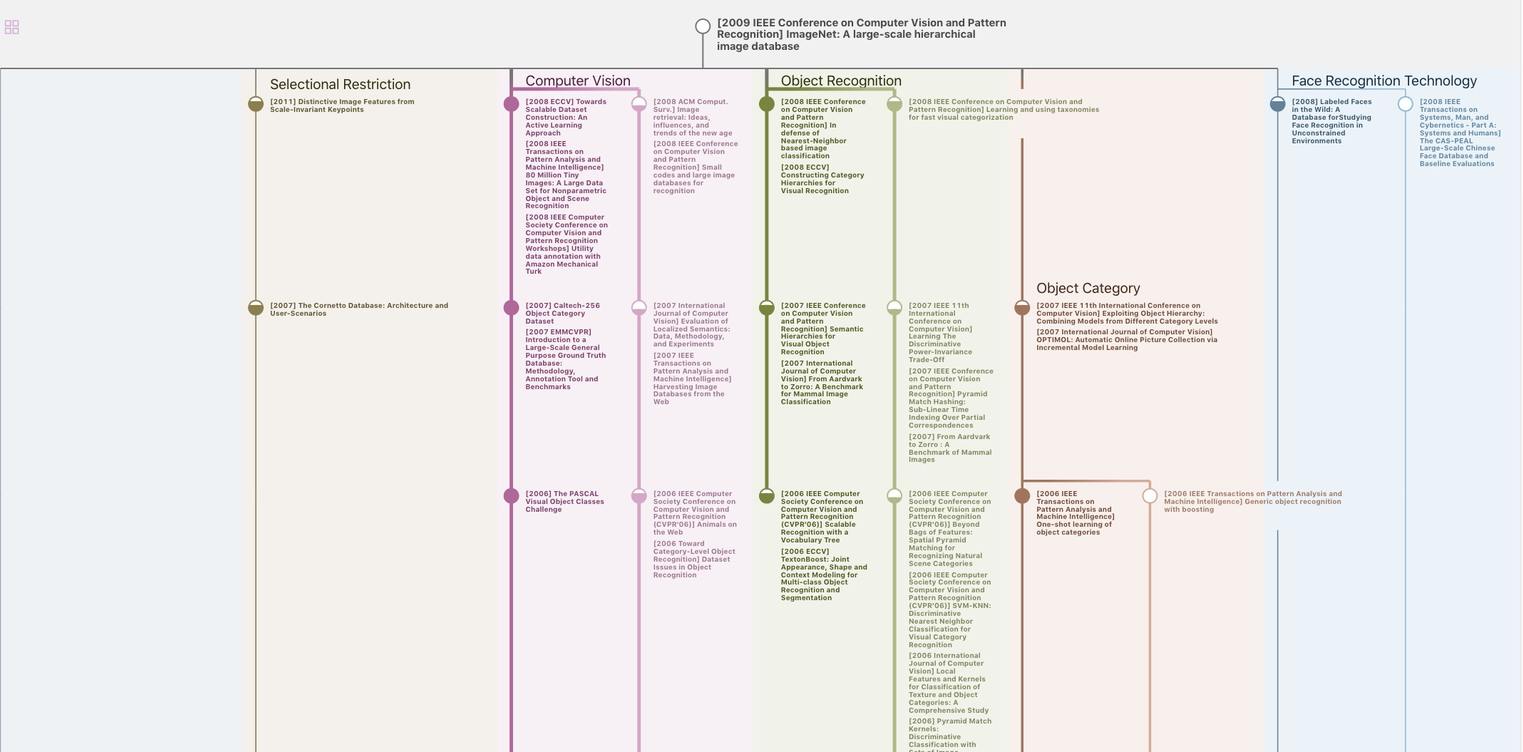
生成溯源树,研究论文发展脉络
Chat Paper
正在生成论文摘要