Data-Driven Safety-Critical Control: Synthesizing Control Barrier Functions With Koopman Operators
IEEE Control Systems Letters(2021)
摘要
Control barrier functions (CBFs) are a powerful tool to guarantee safety of autonomous systems, yet they rely on the computation of control invariant sets, which is notoriously difficult. A backup strategy employs an implicit control invariant set computed by forward integrating the system dynamics. However, this integration is prohibitively expensive for high dimensional systems, and inaccurate in the presence of unmodelled dynamics. We propose to learn discrete-time Koopman operators of the closed-loop dynamics under a backup strategy. This approach replaces forward integration by a simple matrix multiplication, which can mostly be computed offline. We also derive an error bound on the unmodeled dynamics in order to robustify the CBF controller. Our approach extends to multi-agent systems, and we demonstrate the method on collision avoidance for wheeled robots and quadrotors.
更多查看译文
关键词
Robotics,computational methods,supervisory control
AI 理解论文
溯源树
样例
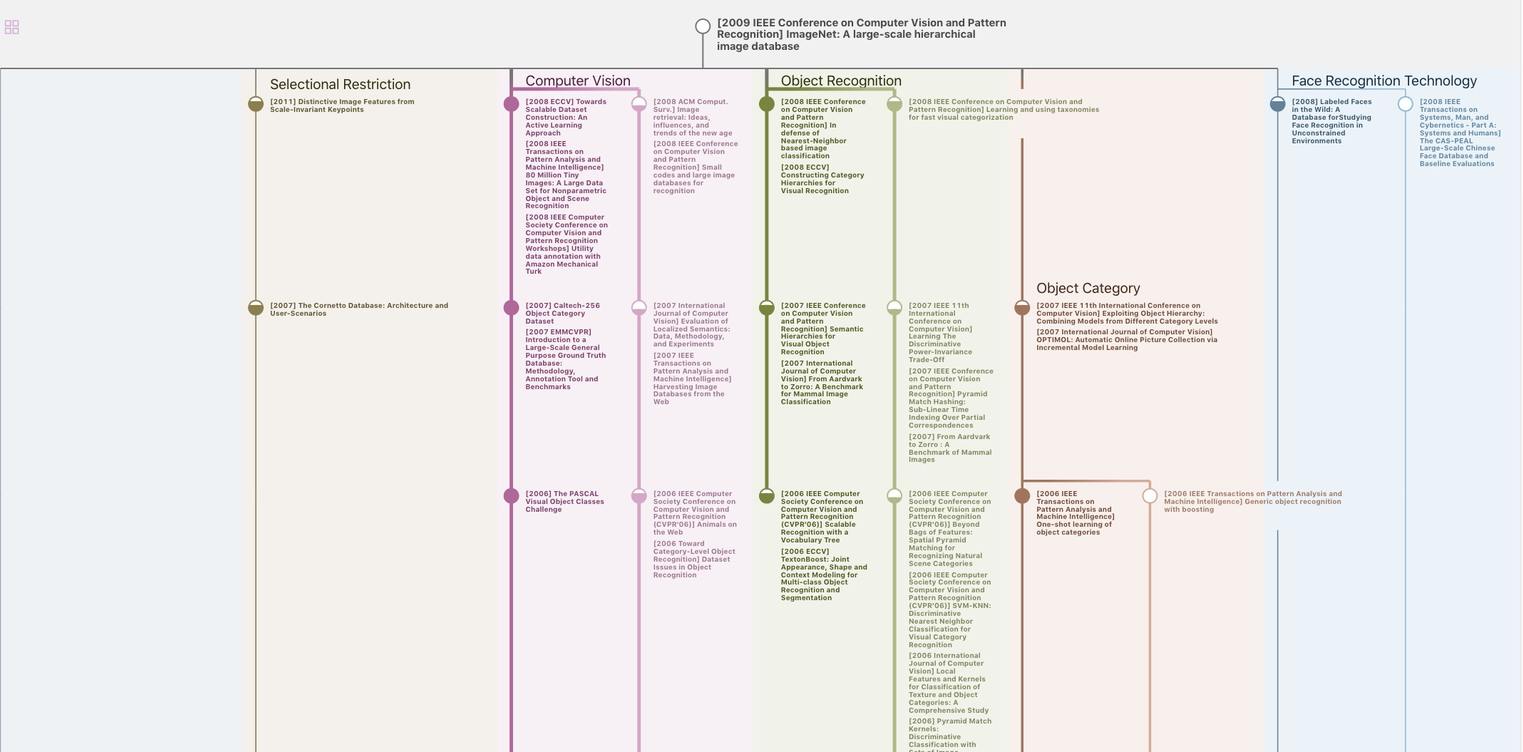
生成溯源树,研究论文发展脉络
Chat Paper
正在生成论文摘要