Acsnet: Action-Context Separation Network For Weakly Supervised Temporal Action Localization
THIRTY-FIFTH AAAI CONFERENCE ON ARTIFICIAL INTELLIGENCE, THIRTY-THIRD CONFERENCE ON INNOVATIVE APPLICATIONS OF ARTIFICIAL INTELLIGENCE AND THE ELEVENTH SYMPOSIUM ON EDUCATIONAL ADVANCES IN ARTIFICIAL INTELLIGENCE(2021)
摘要
The object of Weakly-supervised Temporal Action Localization (WS -TAL) is to localize all action instances in an untrimmed video with only video-level supervision. Due to the lack of frame-level annotations during training, current WS -TAL methods rely on attention mechanisms to localize the foreground snippets or frames that contribute to the video-level classification task. This strategy frequently confuse context with the actual action, in the localization result. Separating action and context is a core problem for precise WS -TAL, but it is very challenging and has been largely ignored in the literature. In this paper, we introduce an Action-Context Separation Network (ACSNet) that explicitly takes into account context for accurate action localization. It consists of two branches (i.e., the Foreground-Background branch and the Action-Context branch). The Foreground-Background branch first distinguishes foreground from background within the entire video while the Action-Context branch further separates the foreground as action and context. We associate video snippets with two latent components (i.e., a positive component and a negative component), and their different combinations can effectively characterize foreground, action and context. Furthermore, we introduce extended labels with auxiliary context categories to facilitate the learning of action-context separation. Experiments on THUMOS14 and ActivityNet v1.2/v1.3 datasets demonstrate the ACSNet outperforms existing state-of-the-art WS-TAL methods by a large margin.
更多查看译文
关键词
action-context localization,weakly supervised temporal
AI 理解论文
溯源树
样例
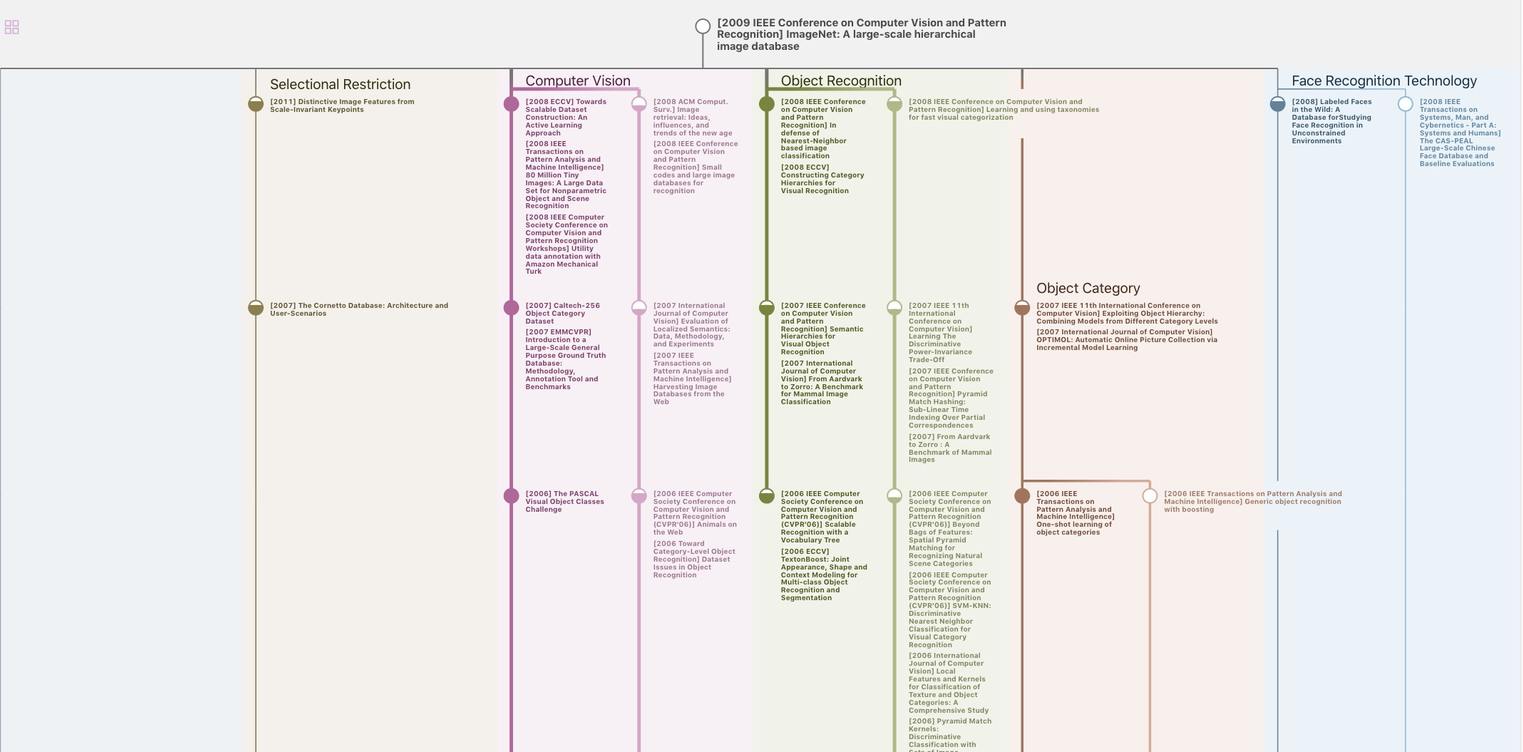
生成溯源树,研究论文发展脉络
Chat Paper
正在生成论文摘要