Natural Criteria for Comparison of Pedestrian Flow Forecasting Models.
IROS(2020)
摘要
Models of human behaviour, such as pedestrian flows, are beneficial for safe and efficient operation of mobile robots. We present a new methodology for benchmarking of pedestrian flow models based on the afforded safety of robot navigation in human-populated environments. While previous evaluations of pedestrian flow models focused on their predictive capabilities, we assess their ability to support safe path planning and scheduling. Using real-world datasets gathered continuously over several weeks, we benchmark state-of-the-art pedestrian flow models, including both time-averaged and time-sensitive models. In the evaluation, we use the learned models to plan robot trajectories and then observe the number of times when the robot gets too close to humans, using a predefined social distance threshold. The experiments show that while traditional evaluation criteria based on model fidelity differ only marginally, the introduced criteria vary significantly depending on the model used, providing a natural interpretation of the expected safety of the system. For the time-averaged flow models, the number of encounters increases linearly with the percentage operating time of the robot, as might be reasonably expected. By contrast, for the time-sensitive models, the number of encounters grows sublinearly with the percentage operating time, by planning to avoid congested areas and times.
更多查看译文
关键词
time-sensitive models,robot trajectories,time-averaged flow models,pedestrian flow forecasting models,human behaviour,safe operation,mobile robots,robot navigation,human-populated environments,safe path planning,scheduling,learned models
AI 理解论文
溯源树
样例
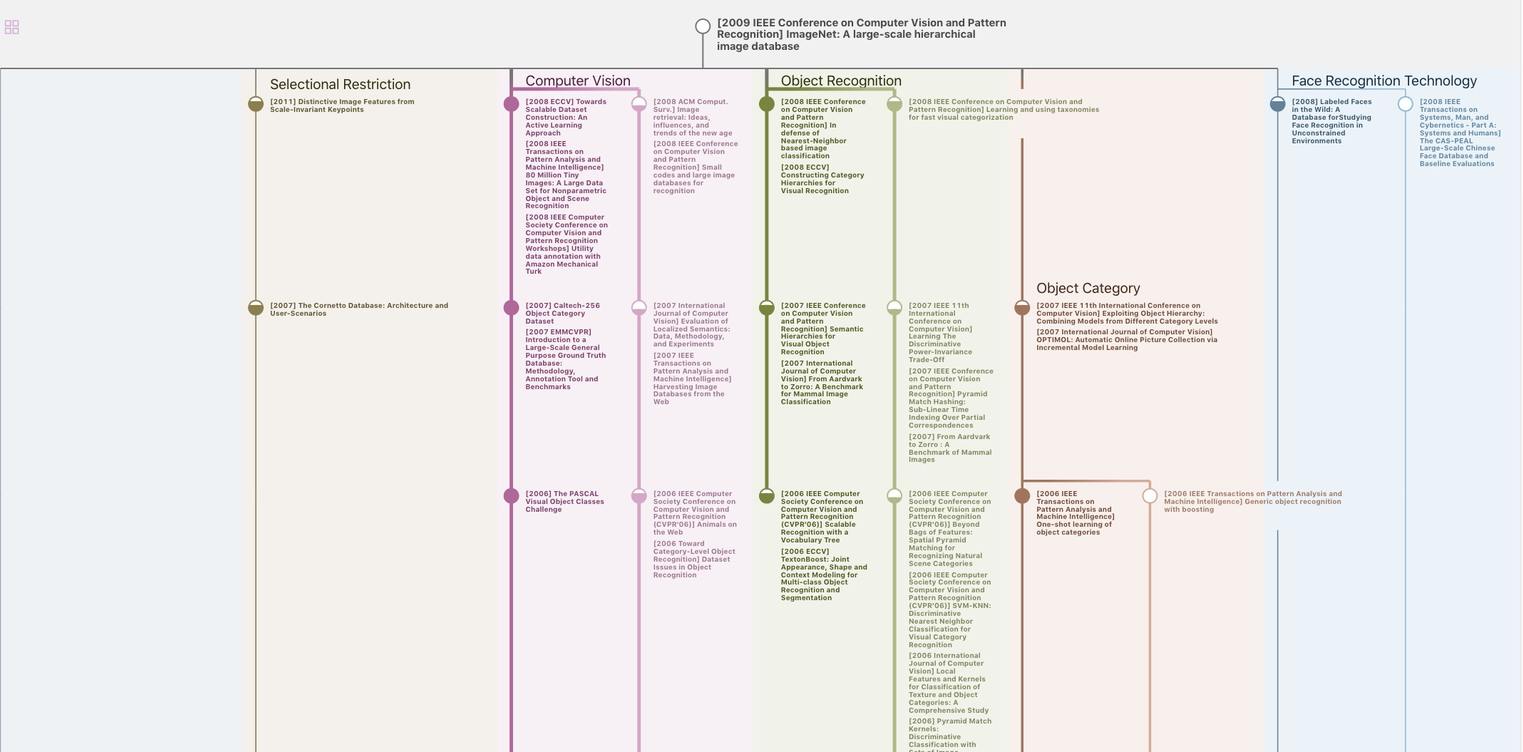
生成溯源树,研究论文发展脉络
Chat Paper
正在生成论文摘要