Regularizing towards Causal Invariance: Linear Models with Proxies
INTERNATIONAL CONFERENCE ON MACHINE LEARNING, VOL 139(2021)
摘要
We propose a method for learning linear models whose predictive performance is robust to causal interventions on unobserved variables, when noisy proxies of those variables are available. Our approach takes the form of a regularization term that trades off between in-distribution performance and robustness to interventions. Under the assumption of a linear structural causal model, we show that a single proxy can be used to create estimators that are prediction optimal under interventions of bounded strength. This strength depends on the magnitude of the measurement noise in the proxy, which is, in general, not identifiable. In the case of two proxy variables, we propose a modified estimator that is prediction optimal under interventions up to a known strength. We further show how to extend these estimators to scenarios where additional information about the "test time" intervention is available during training. We evaluate our theoretical findings in synthetic experiments and using real data of hourly pollution levels across several cities in China.
更多查看译文
关键词
causal invariance,linear models,proxies
AI 理解论文
溯源树
样例
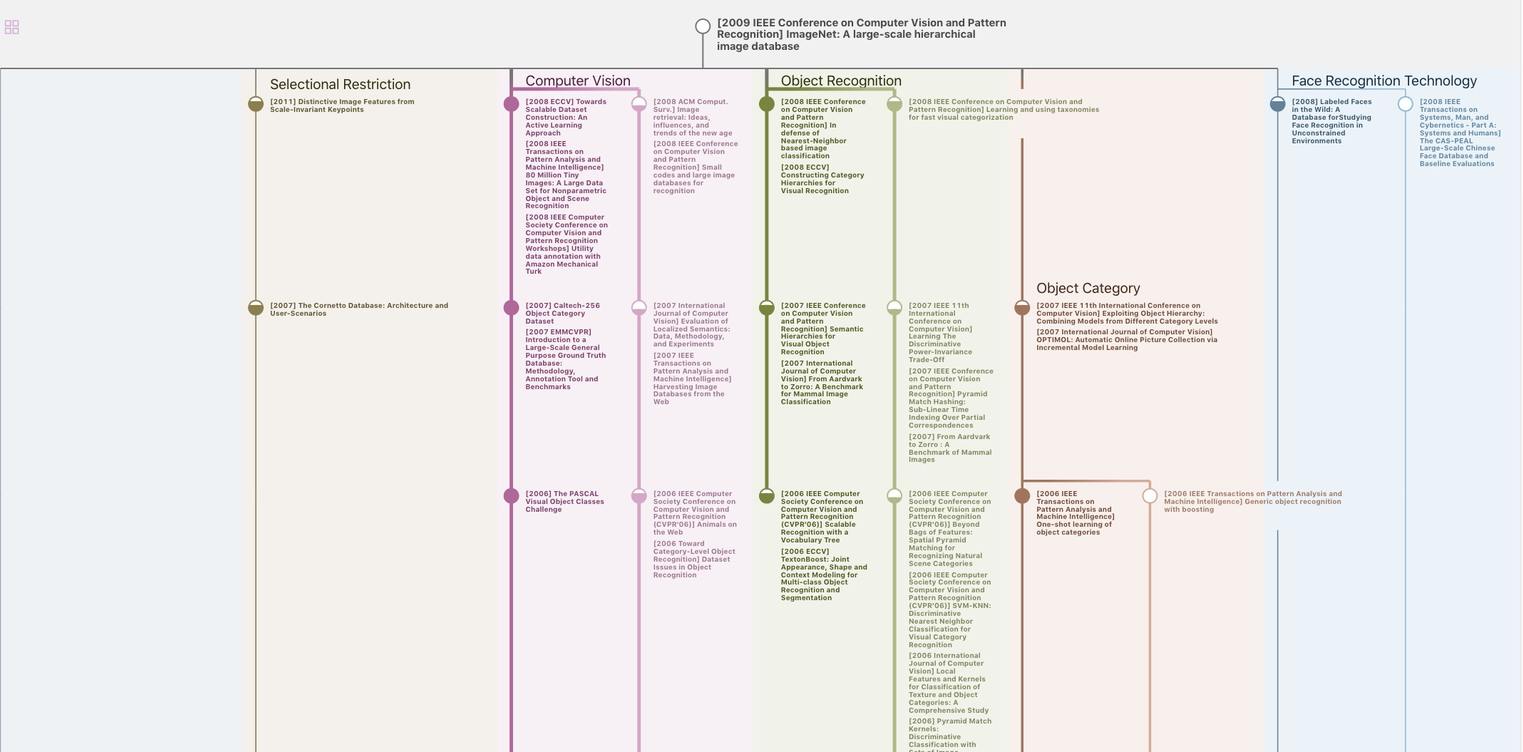
生成溯源树,研究论文发展脉络
Chat Paper
正在生成论文摘要