Deep Vessel Tracking: A Generalized Probabilistic Approach Via Deep Learning
2016 IEEE 13th International Symposium on Biomedical Imaging (ISBI)(2016)
摘要
Analysis of vascular geometry is important in many medical imaging applications, such as retinal, pulmonary, and cardiac investigations. In order to make reliable judgments for clinical usage, accurate and robust segmentation methods are needed. Due to the high complexity of biological vasculature trees, manual identification is often too time-consuming and tedious to be used in practice. To design an automated and computerized method, a major challenge is that the appearance of vasculatures in medical images has great variance across modalities and subjects. Therefore, most existing approaches are specially designed for a particular task, lacking the flexibility to be adapted to other circumstances. In this paper, we present a generic approach for vascular structure identification from medical images, which can be used for multiple purposes robustly. The proposed method uses the state-of-the-art deep convolutional neural network (CNN) to learn the appearance features of the target. A Principal Component Analysis (PCA)-based nearest neighbor search is then utilized to estimate the local structure distribution, which is further incorporated within the generalized probabilistic tracking framework to extract the entire connected tree. Qualitative and quantitative results over retinal fundus data demonstrate that the proposed framework achieves comparable accuracy as compared with state-of-the-art methods, while efficiently producing more information regarding the candidate tree structure.
更多查看译文
关键词
Vascular Structure,Deep Learning,Principal Component Analysis,Nearest Neighbor Search,Generalized Probabilistic Tracking
AI 理解论文
溯源树
样例
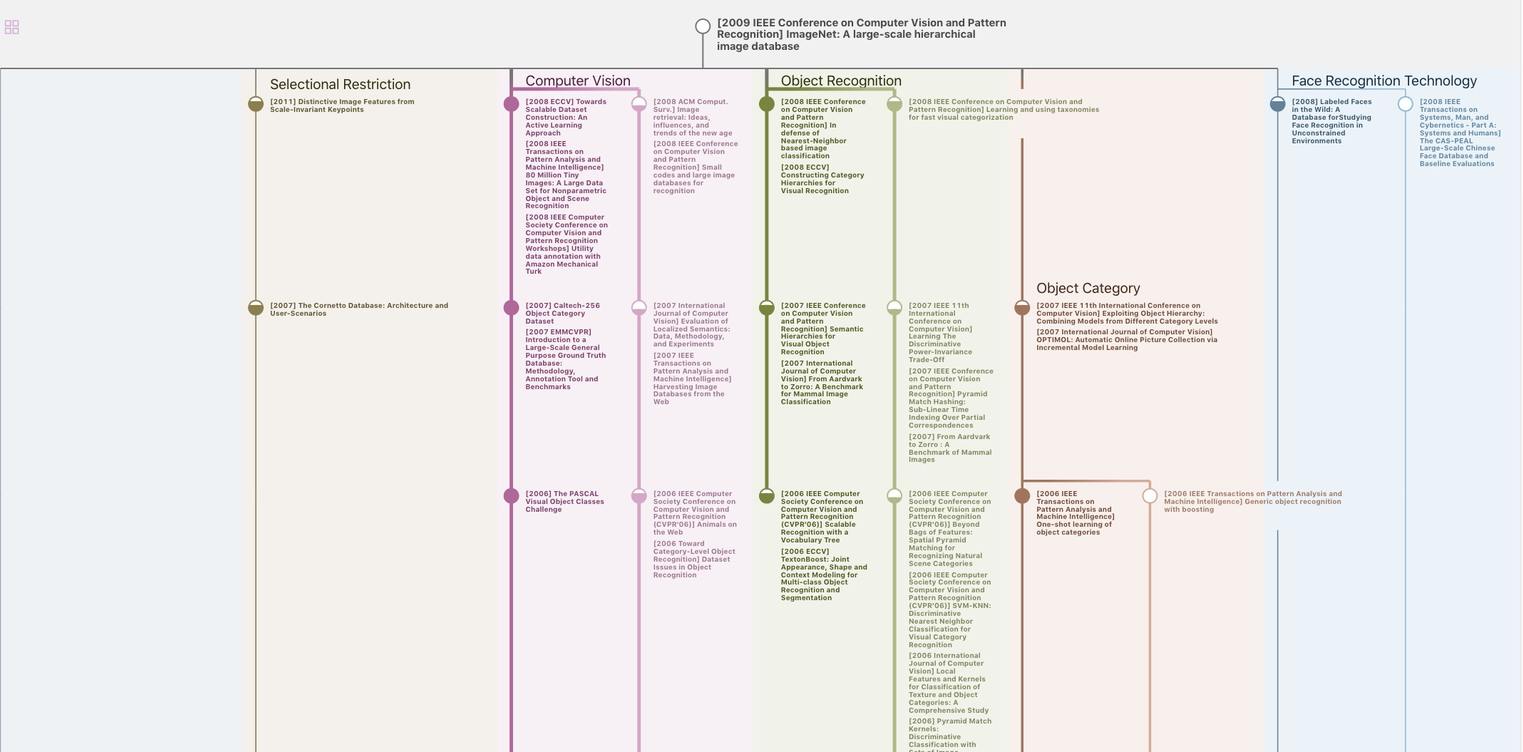
生成溯源树,研究论文发展脉络
Chat Paper
正在生成论文摘要