Thousand to One - Semantic Prior Modeling for Conceptual Coding.
ICME(2021)
摘要
Conceptual coding has been an emerging research topic recently, which encodes natural images into disentangled conceptual representations for compression. However, the compression performance of the existing methods is still sub-optimal due to the lack of comprehensive consideration of rate constraint and reconstruction quality. To this end, we propose a novel end-to-end semantic prior modeling-based conceptual coding scheme towards extremely low bitrate image compression, which leverages semantic-wise deep representations as a unified prior for entropy estimation and texture synthesis. Specifically, we employ semantic segmentation maps as structural guidance for extracting deep semantic prior, which provides fine-grained texture distribution modeling for better detail construction and higher flexibility in subsequent high-level vision tasks. Moreover, a cross-channel entropy model is proposed to further exploit the inter-channel correlation of the spatially independent semantic prior, leading to more accurate entropy estimation for rate-constrained training. The proposed scheme achieves an ultra-high 1000x compression ratio, while still enjoying high visual reconstruction quality and versatility towards visual processing and analysis tasks.
更多查看译文
关键词
semantic prior,modeling
AI 理解论文
溯源树
样例
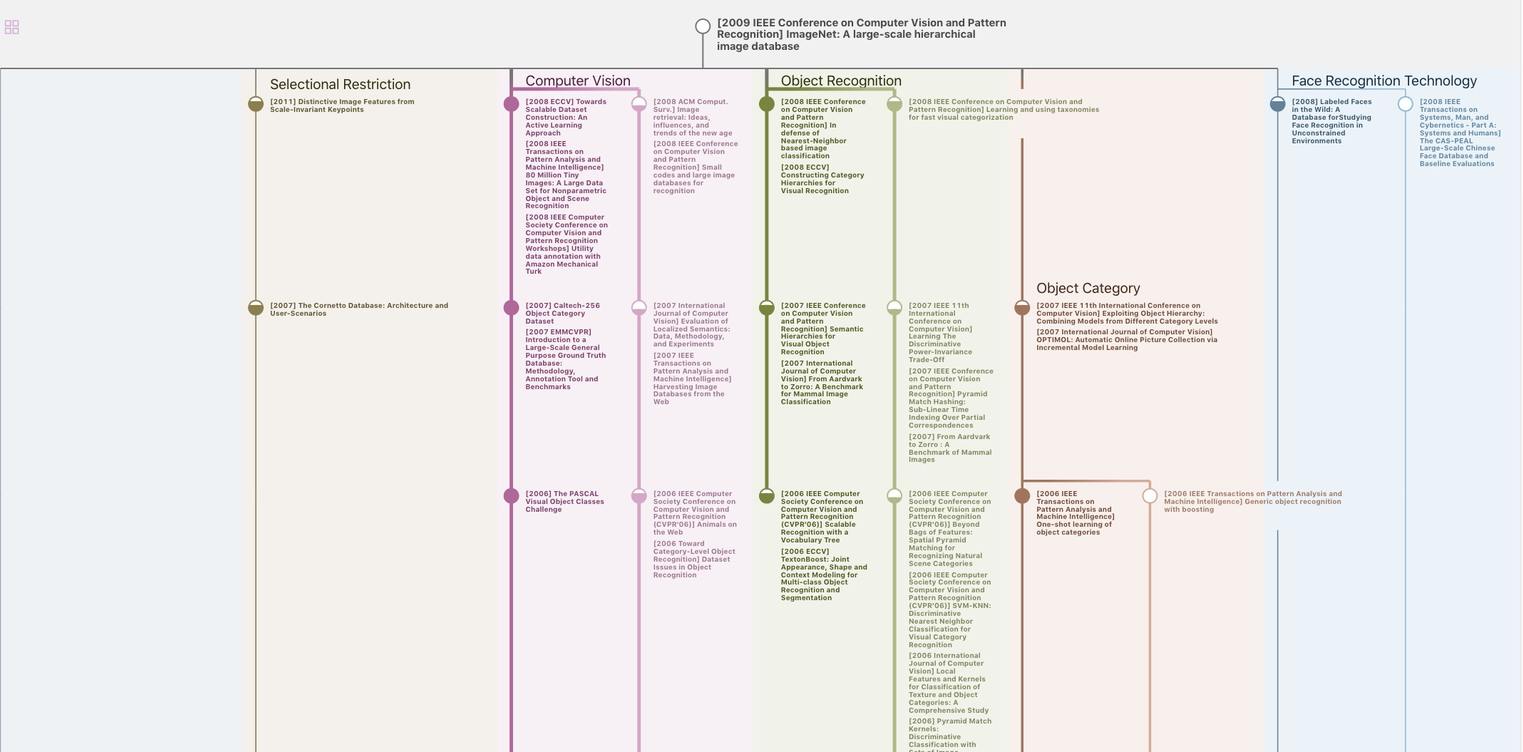
生成溯源树,研究论文发展脉络
Chat Paper
正在生成论文摘要