Back to the Feature: Learning Robust Camera Localization from Pixels to Pose
2021 IEEE/CVF CONFERENCE ON COMPUTER VISION AND PATTERN RECOGNITION, CVPR 2021(2021)
摘要
Camera pose estimation in known scenes is a 3D geometry task recently tackled by multiple learning algorithms. Many regress precise geometric quantities, like poses or 3D points, from an input image. This either fails to generalize to new viewpoints or ties the model parameters to a specific scene. In this paper, we go Back to the Feature: we argue that deep networks should focus on learning robust and invariant visual features, while the geometric estimation should be left to principled algorithms. We introduce PixLoc, a scene-agnostic neural network that estimates an accurate 6-DoF pose from an image and a 3D model. Our approach is based on the direct alignment of multiscale deep features, casting camera localization as metric learning. PixLoc learns strong data priors by end-to-end training from pixels to pose and exhibits exceptional generalization to new scenes by separating model parameters and scene geometry. The system can localize in large environments given coarse pose priors but also improve the accuracy of sparse feature matching by jointly refining keypoints and poses with little overhead. The code will be publicly available at github.com/cvg/pixloc.
更多查看译文
关键词
learning robust camera localization,pixels,feature
AI 理解论文
溯源树
样例
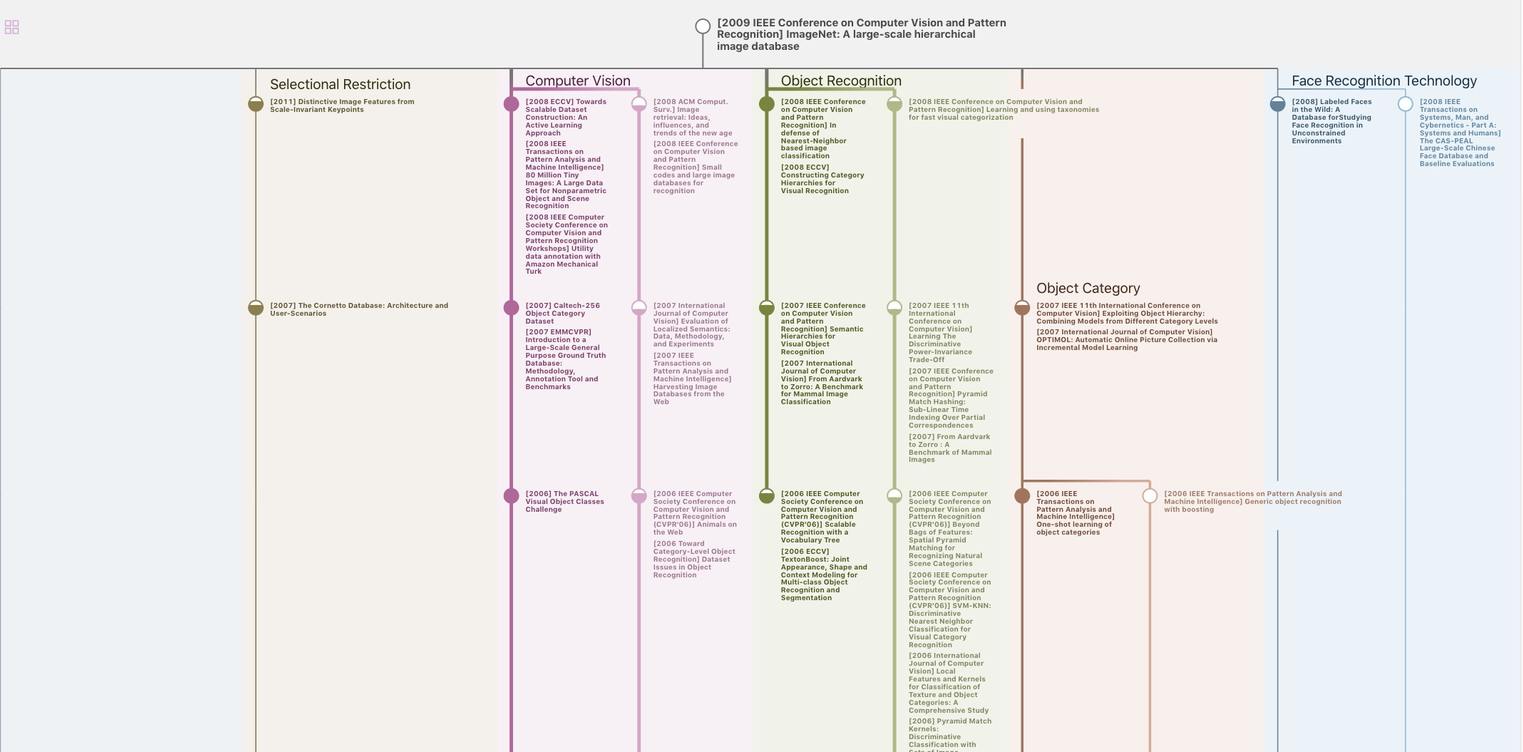
生成溯源树,研究论文发展脉络
Chat Paper
正在生成论文摘要