MSCANet: Adaptive Multi-scale Context Aggregation Network for Congested Crowd Counting.
MMM (2)(2021)
摘要
Crowd counting has achieved significant progress with deep convolutional neural networks. However, most of the existing methods don’t fully utilize spatial context information, and it is difficult for them to count the congested crowd accurately. To this end, we propose a novel Adaptive Multi-scale Context Aggregation Network (MSCANet), in which a Multi-scale Context Aggregation module (MSCA) is designed to adaptively extract and aggregate the contextual information from different scales of the crowd. More specifically, for each input, we first extract multi-scale context features via atrous convolution layers. Then, the multi-scale context features are progressively aggregated via a channel attention to enrich the crowd representations in different scales. Finally, a 1 × 1 convolution layer is applied to regress the crowd density. We perform extensive experiments on three public datasets: ShanghaiTech Part_A, UCF_CC_50 and UCF-QNRF, and the experimental results demonstrate the superiority of our method compared to current the state-of-the-art methods.
更多查看译文
关键词
congested crowd counting,context,multi-scale
AI 理解论文
溯源树
样例
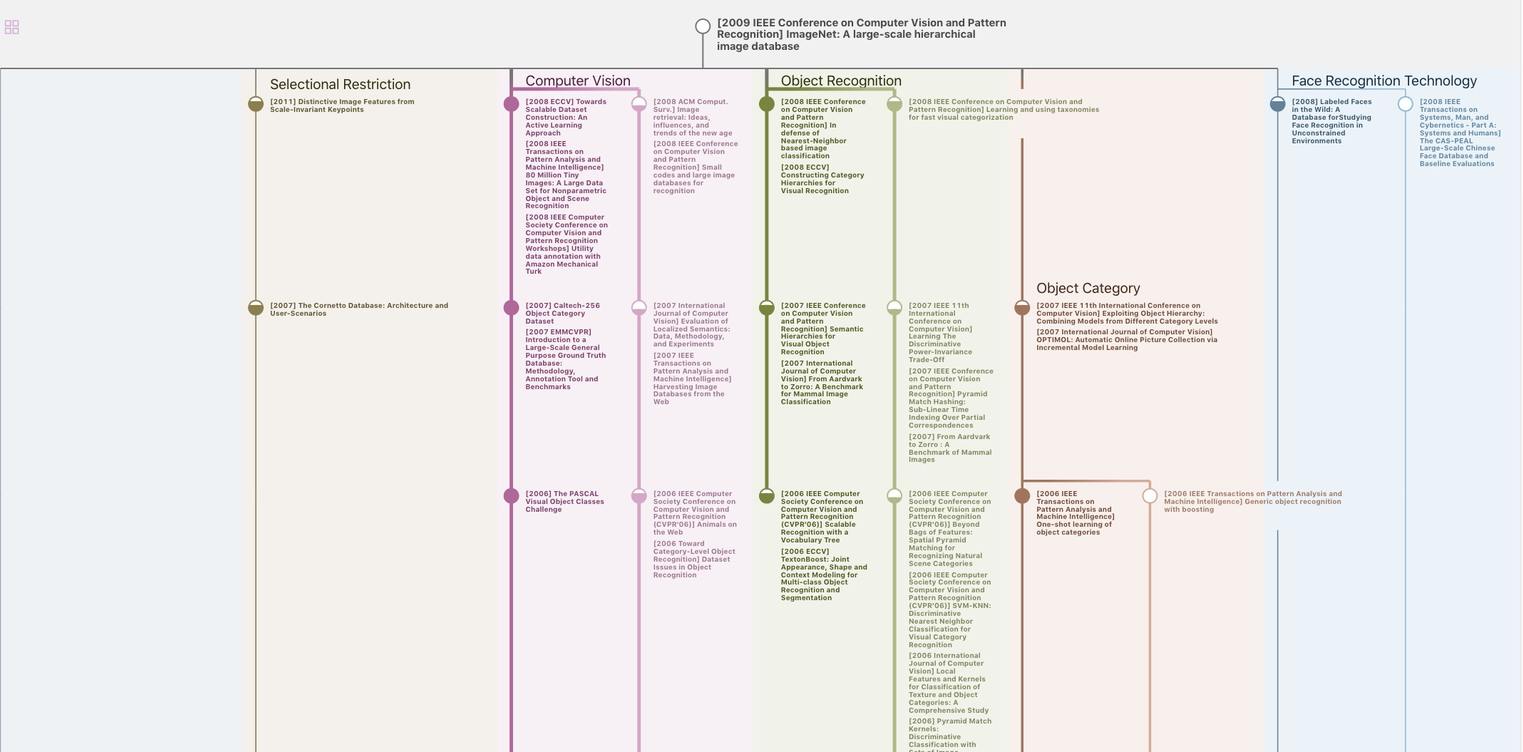
生成溯源树,研究论文发展脉络
Chat Paper
正在生成论文摘要