Learning-Based Network Boolean Tomography for Identifying Congested Links with Correlations.
GLOBECOM(2020)
摘要
The accurate identification of congested links is crucial for network performance monitoring. Network boolean tomography uses end-to-end path measurements to identify congested links, and appears as a significant alternative when direct link monitoring is not available. However, most of existing tomographic methods assume no correlations between links, i.e., the congestion of one link is assumed to be independent from the congestion of any others, hindering their applications in practice because links could become correlated during a joint optimization procedure of many network operations like traffic routing and balancing. In this paper, we study practical network boolean tomography without such an assumption. We elaborate on the ill-posed nature of network boolean tomography to highlight the significance of integrating link correlations, and model the congested link identification from end-to-end congestion observations of paths as a problem of Maximum A-Posteriori (MAP) estimation. To avoid the explicit acquisition of any priori knowledge of link correlations, we then propose a learning-based algorithm with Long Short-term Memory (LSTM), a special recurrent neural network that is good at learning statistical dependencies of sequence elements from historical data. Numerical results over real network topologies validate our learning-based network boolean tomography.
更多查看译文
关键词
Network Performance Monitoring, Network Tomography, Congested Link Identification, Correlation
AI 理解论文
溯源树
样例
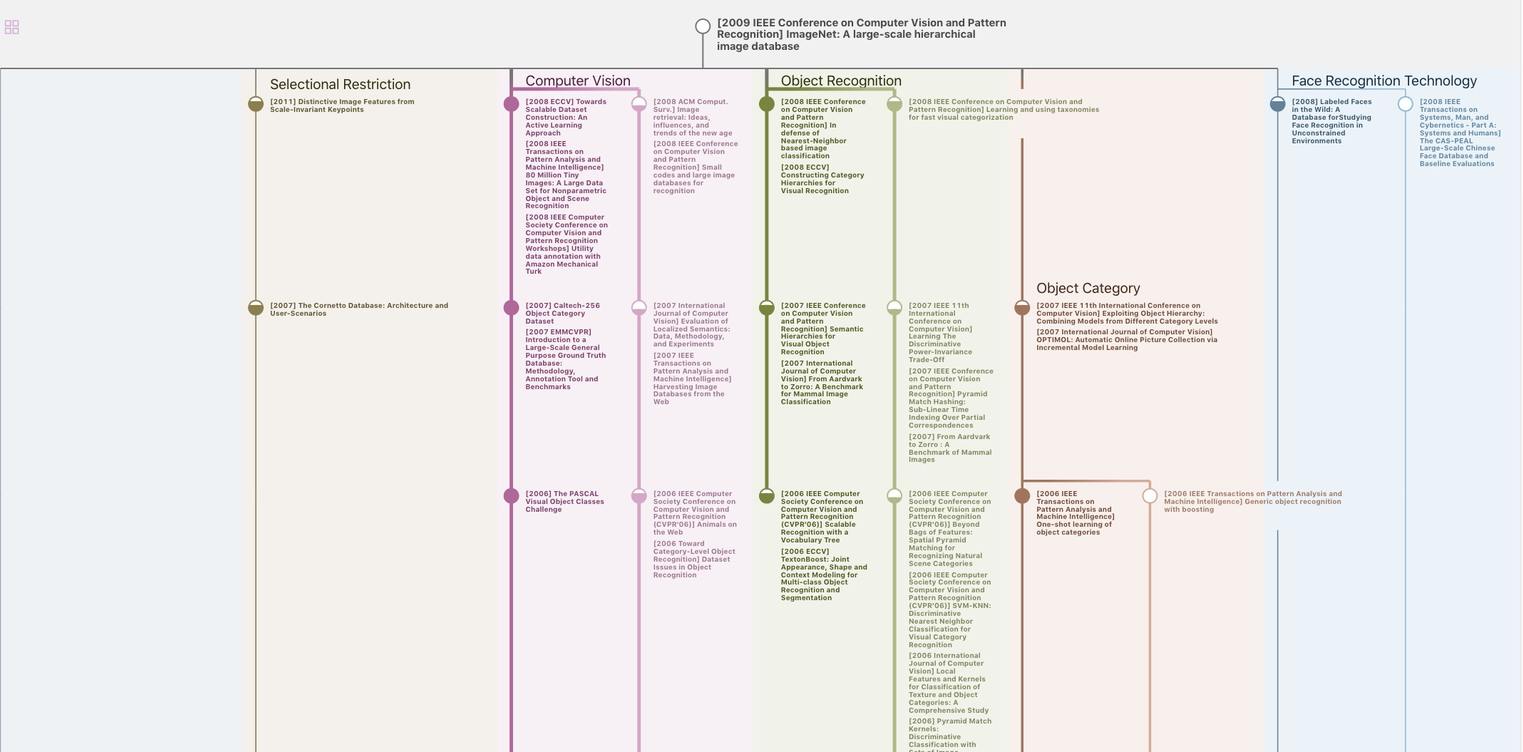
生成溯源树,研究论文发展脉络
Chat Paper
正在生成论文摘要