A Binary-Activation, Multi-Level Weight RNN and Training Algorithm for ADC-/DAC-Free and Noise-Resilient Processing-in-Memory Inference With eNVM
user-5e9d449e4c775e765d44d7c9(2023)
摘要
We propose a new algorithm for training neural networks with binary activations and multi-level weights, which enables efficient processing-in-memory circuits with embedded nonvolatile memories (eNVM). Binary activations obviate costly DACs and ADCs. Multi-level weights leverage multi-level eNVM cells. Compared to existing algorithms, our method not only works for feed-forward networks (e.g., fully-connected and convolutional), but also achieves higher accuracy and noise resilience for recurrent networks. In particular, we present an RNN-based trigger-word detection PIM accelerator, with detailed hardware noise models and circuit co-design techniques, and validate our algorithm's high inference accuracy and robustness against a variety of real hardware non-idealities.
更多查看译文
关键词
Embedded non-volatile memory,in-memory-computing,processing-in-memory,recurrent neural networks,trigger word detection
AI 理解论文
溯源树
样例
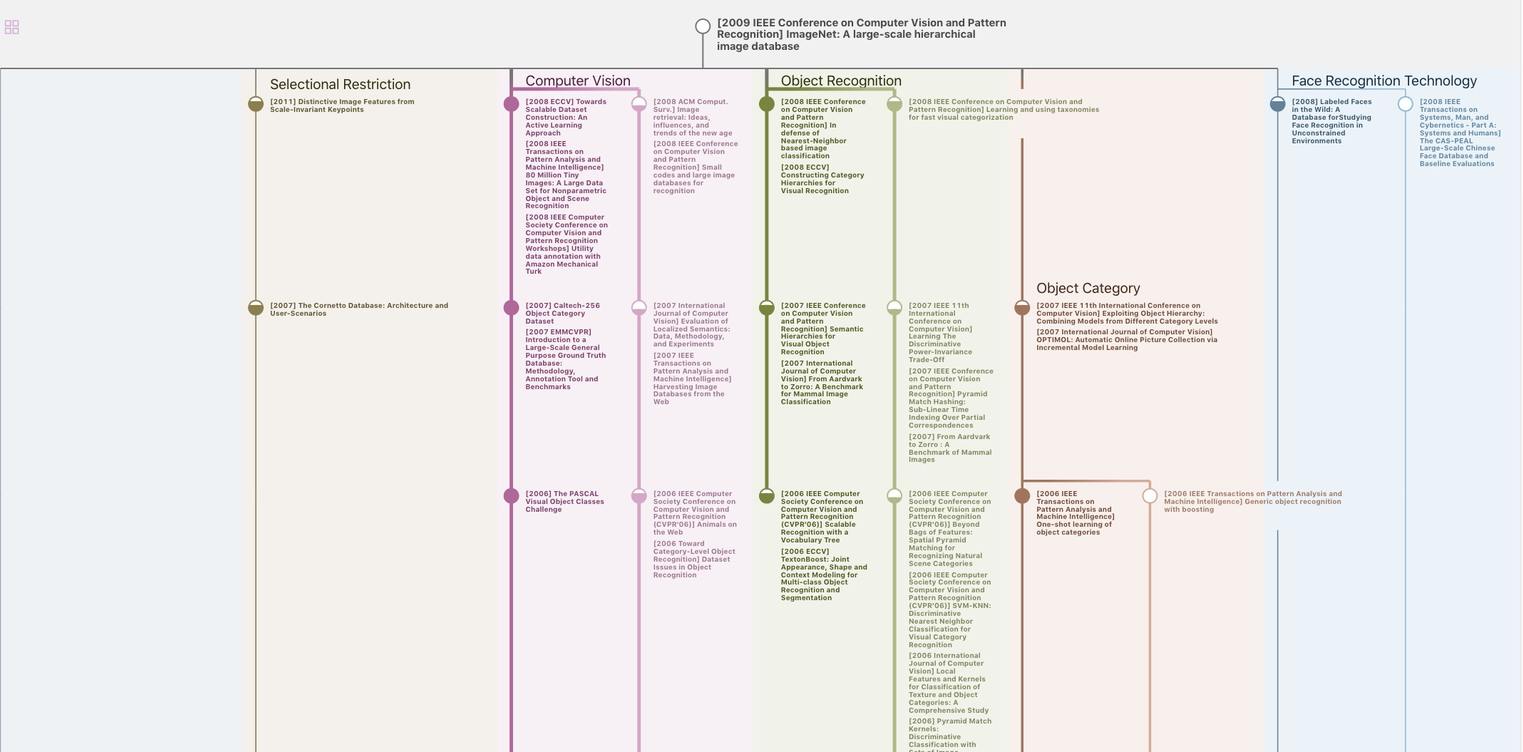
生成溯源树,研究论文发展脉络
Chat Paper
正在生成论文摘要