Reinforcement Learning for Robust Parameterized Locomotion Control of Bipedal Robots
2021 IEEE INTERNATIONAL CONFERENCE ON ROBOTICS AND AUTOMATION (ICRA 2021)(2021)
摘要
Developing robust walking controllers for bipedal robots is a challenging endeavor. Traditional model-based locomotion controllers require simplifying assumptions and careful modelling; any small errors can result in unstable control. To address these challenges for bipedal locomotion, we present a model-free reinforcement learning framework for training robust locomotion policies in simulation, which can then be transferred to a real bipedal Cassie robot. To facilitate sim-toreal transfer, domain randomization is used to encourage the policies to learn behaviors that are robust across variations in system dynamics. The learned policies enable Cassie to perform a set of diverse and dynamic behaviors, while also being more robust than traditional controllers and prior learning-based methods that use residual control. We demonstrate this on versatile walking behaviors such as tracking a target walking velocity, walking height, and turning yaw. (Video(1))
更多查看译文
关键词
careful modelling,unstable control,bipedal locomotion,model-free reinforcement learning framework,robust locomotion policies,bipedal Cassie robot,sim-to-real transfer,learned policies,diverse behaviors,dynamic behaviors,traditional controllers,prior learning-based methods,residual control,versatile walking,target walking velocity,robust parameterized locomotion control,bipedal robots,robust walking controllers,challenging endeavor,traditional model-based locomotion controllers
AI 理解论文
溯源树
样例
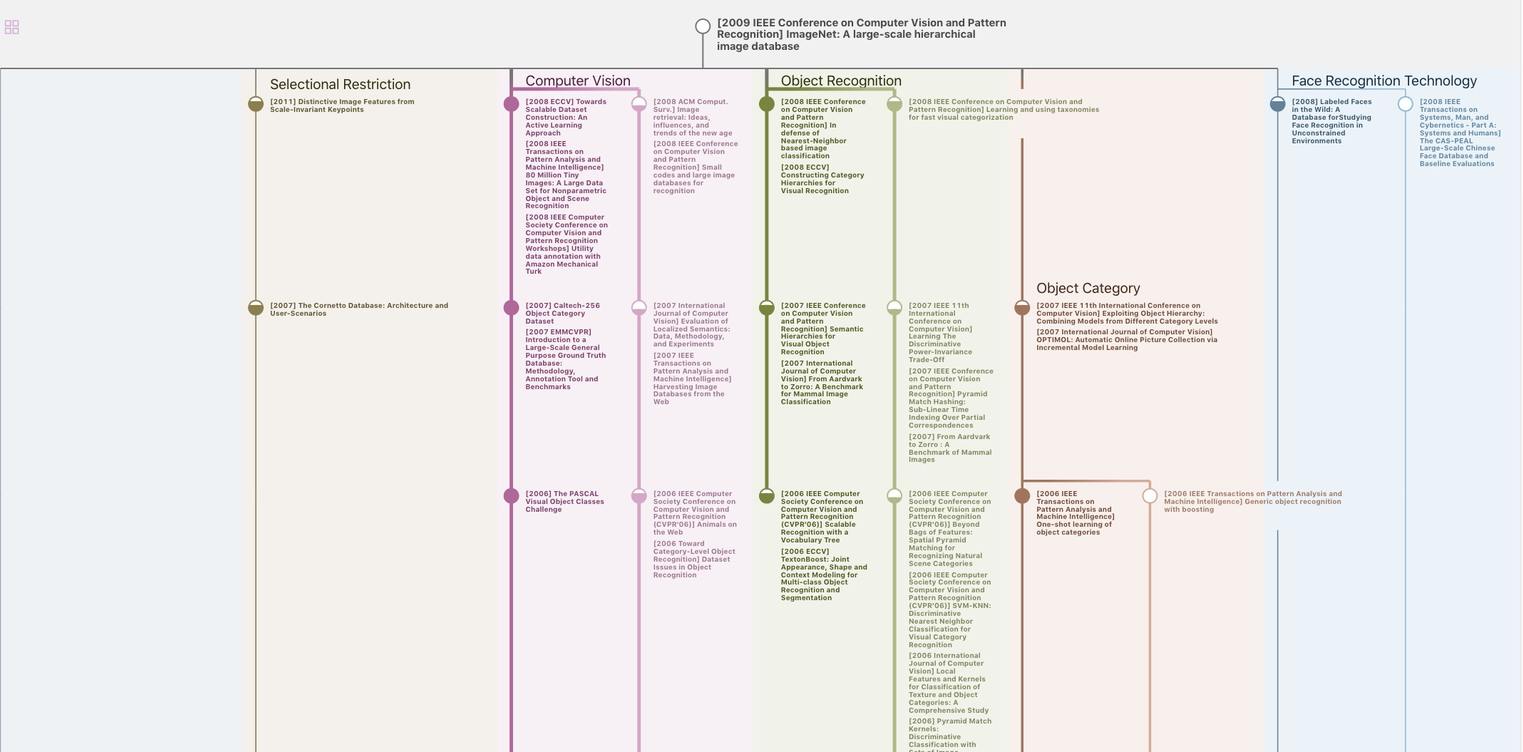
生成溯源树,研究论文发展脉络
Chat Paper
正在生成论文摘要