Actionable Models: Unsupervised Offline Reinforcement Learning Of Robotic Skills
INTERNATIONAL CONFERENCE ON MACHINE LEARNING, VOL 139(2021)
摘要
We consider the problem of learning useful robotic skills from previously collected offline data without access to manually specified rewards or additional online exploration, a setting that is becoming increasingly important for scaling robot learning by reusing past robotic data. In particular, we propose the objective of learning a functional understanding of the environment by learning to reach any goal state in a given dataset. We employ goal-conditioned Q-learning with hindsight relabeling and develop several techniques that enable training in a particularly challenging offline setting. We find that our method can operate on high-dimensional camera images and learn a variety of skills on real robots that generalize to previously unseen scenes and objects. We also show that our method can learn to reach long-horizon goals across multiple episodes through goal chaining, and learn rich representations that can help with downstream tasks through pre-training or auxiliary objectives. The videos of our experiments can be found at https : / / actionable-models.github.io
更多查看译文
关键词
unsupervised offline reinforcement learning,robotic,skills,models
AI 理解论文
溯源树
样例
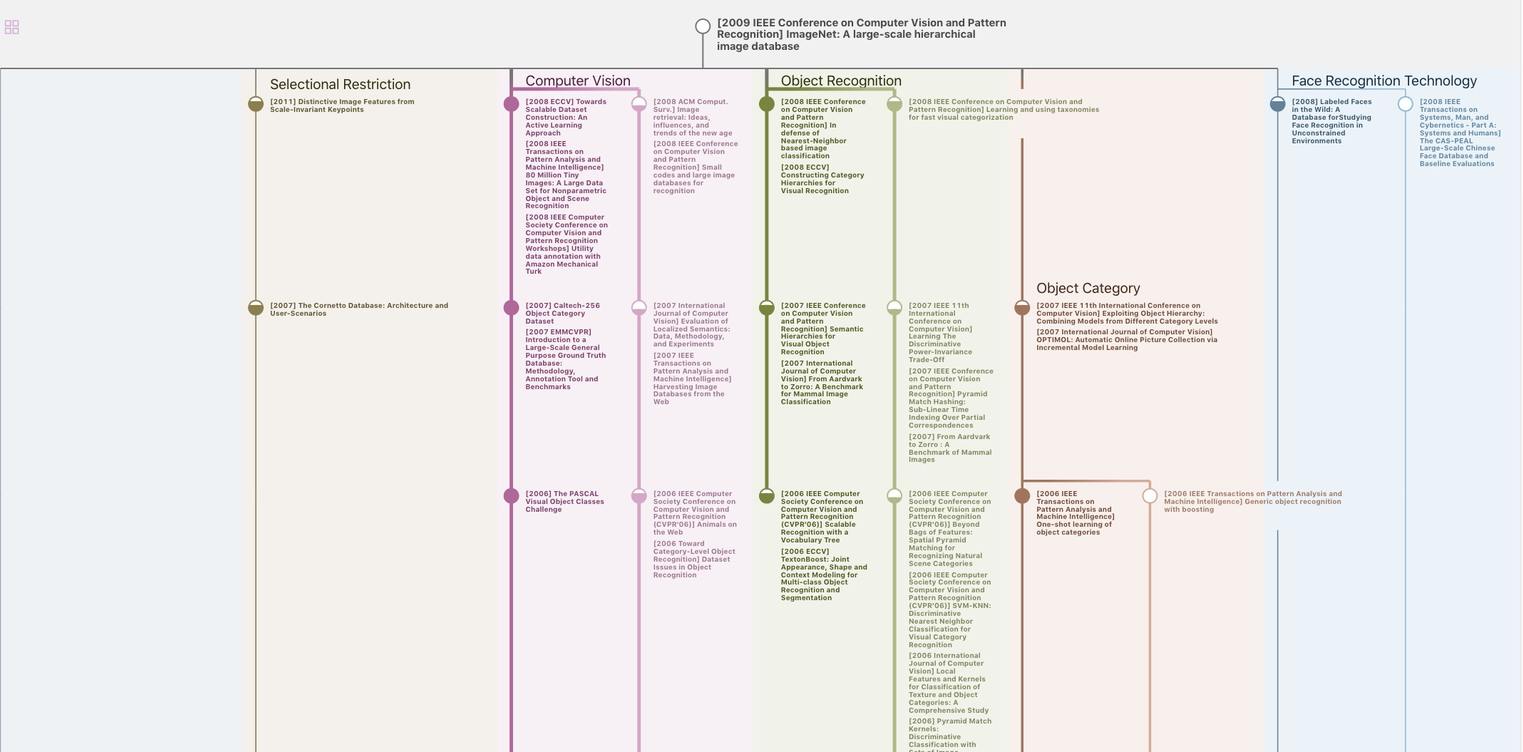
生成溯源树,研究论文发展脉络
Chat Paper
正在生成论文摘要