Identifying Driver Interactions via Conditional Behavior Prediction
2021 IEEE INTERNATIONAL CONFERENCE ON ROBOTICS AND AUTOMATION (ICRA 2021)(2021)
摘要
Interactive driving scenarios, such as lane changes. merges and unprotected turns, are some of the most challenging situations for autonomous driving. Planning in interactive scenarios requires accurately modeling the reactions of other agents to different future actions of the ego agent. We develop end-to-end models for conditional behavior prediction (CBP) that take as an input a query future trajectory for an ego agent, and predict distributions over future trajectories for other agents conditioned on the query. Leveraging such a model, we develop a general-purpose agent interactivity score derived from probabilistic first principles. The interactivity score allows us to find interesting interactive scenarios for training and evaluating behavior prediction models. We further demonstrate that the proposed score is effective for agent prioritization under computational budget constraints.
更多查看译文
关键词
driver interactions,conditional behavior prediction
AI 理解论文
溯源树
样例
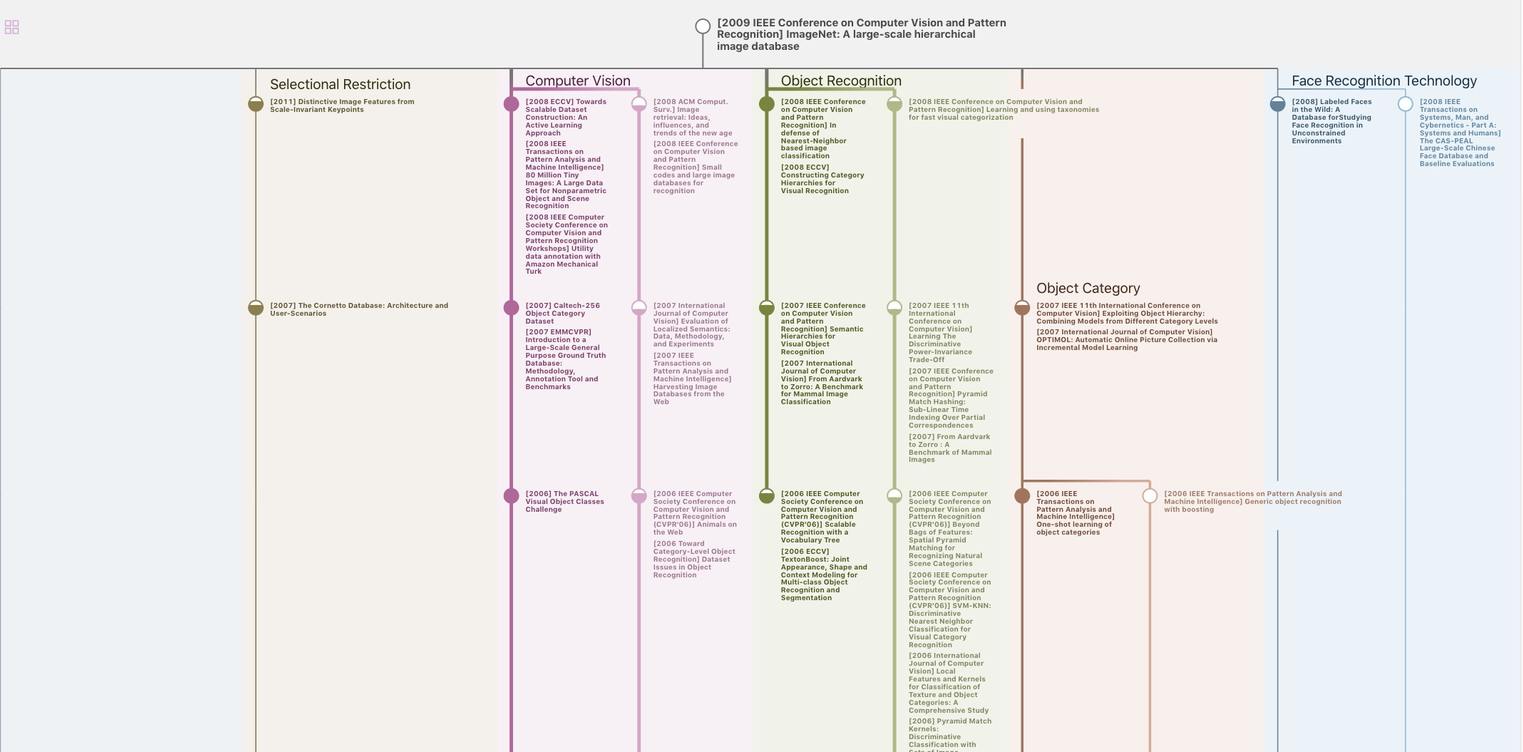
生成溯源树,研究论文发展脉络
Chat Paper
正在生成论文摘要