Accelerated Training Via Device Similarity in Federated Learning
PROCEEDINGS OF THE 4TH INTERNATIONAL WORKSHOP ON EDGE SYSTEMS, ANALYTICS AND NETWORKING (EDGESYS'21)(2021)
Abstract
Federated Learning is a privacy-preserving, machine learning technique that generates a globally shared model with in-situ model training on distributed devices. These systems are often comprised of millions of user devices and only a subset of available devices can be used for training in each epoch. Designing a device selection strategy is challenging, given that devices are highly heterogeneous in both their system resources and training data. This heterogeneity makes device selection very crucial for timely model convergence and sufficient model accuracy. Existing approaches have addressed system heterogeneity for device selection but have largely ignored the data heterogeneity. In this work, we analyze the impact of data heterogeneity on device selection, model convergence, model accuracy, and fault tolerance in a federated learning setting. Based on our analysis, we propose that clustering devices with similar data distributions followed by selecting the devices with the best processing capacity from each cluster can significantly improve the model convergence without compromising model accuracy. This clustering also guides us in designing policies for fault tolerance in the system. We propose three methods for identifying groups of devices with similar data distributions. We also identify and discuss rich trade-offs between privacy, bandwidth consumption, and computation overhead for each of these proposed methods. Our preliminary experiments show that the proposed methods can provide a 46% - 58% reduction in training time compared to existing approaches in reaching the same accuracy.
MoreTranslated text
PDF
View via Publisher
AI Read Science
Must-Reading Tree
Example
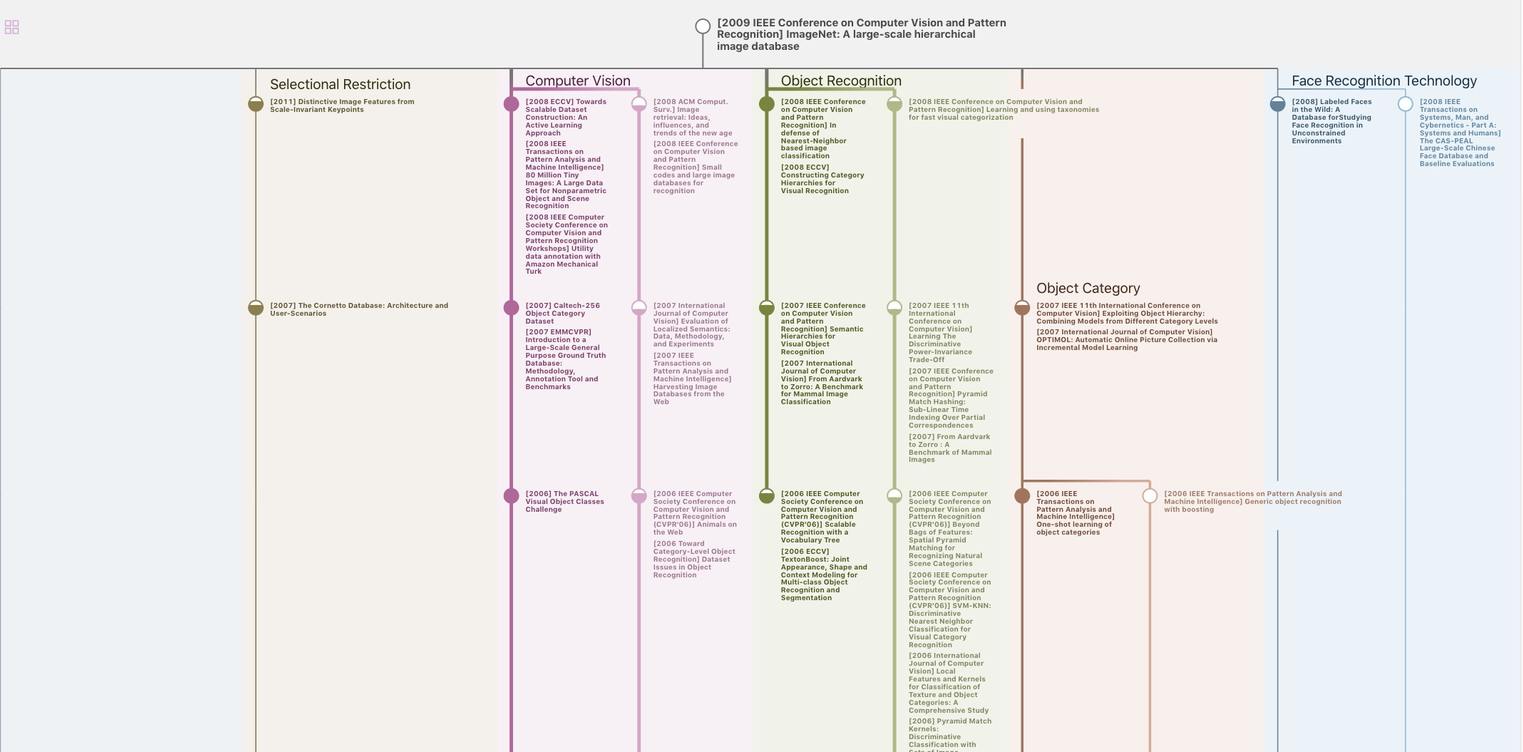
Generate MRT to find the research sequence of this paper
Related Papers
2018
被引用1731 | 浏览
2018
被引用568 | 浏览
2020
被引用373 | 浏览
2020
被引用135 | 浏览
2020
被引用2 | 浏览
Data Disclaimer
The page data are from open Internet sources, cooperative publishers and automatic analysis results through AI technology. We do not make any commitments and guarantees for the validity, accuracy, correctness, reliability, completeness and timeliness of the page data. If you have any questions, please contact us by email: report@aminer.cn
Chat Paper
去 AI 文献库 对话