RHUPS: Mining Recent High Utility Patterns with Sliding Window-based Arrival Time Control over Data Streams
ACM Transactions on Intelligent Systems and Technology(2021)
关键词
Recent high utility pattern,stream database,sliding window,evolutionary time-fading factor
AI 理解论文
溯源树
样例
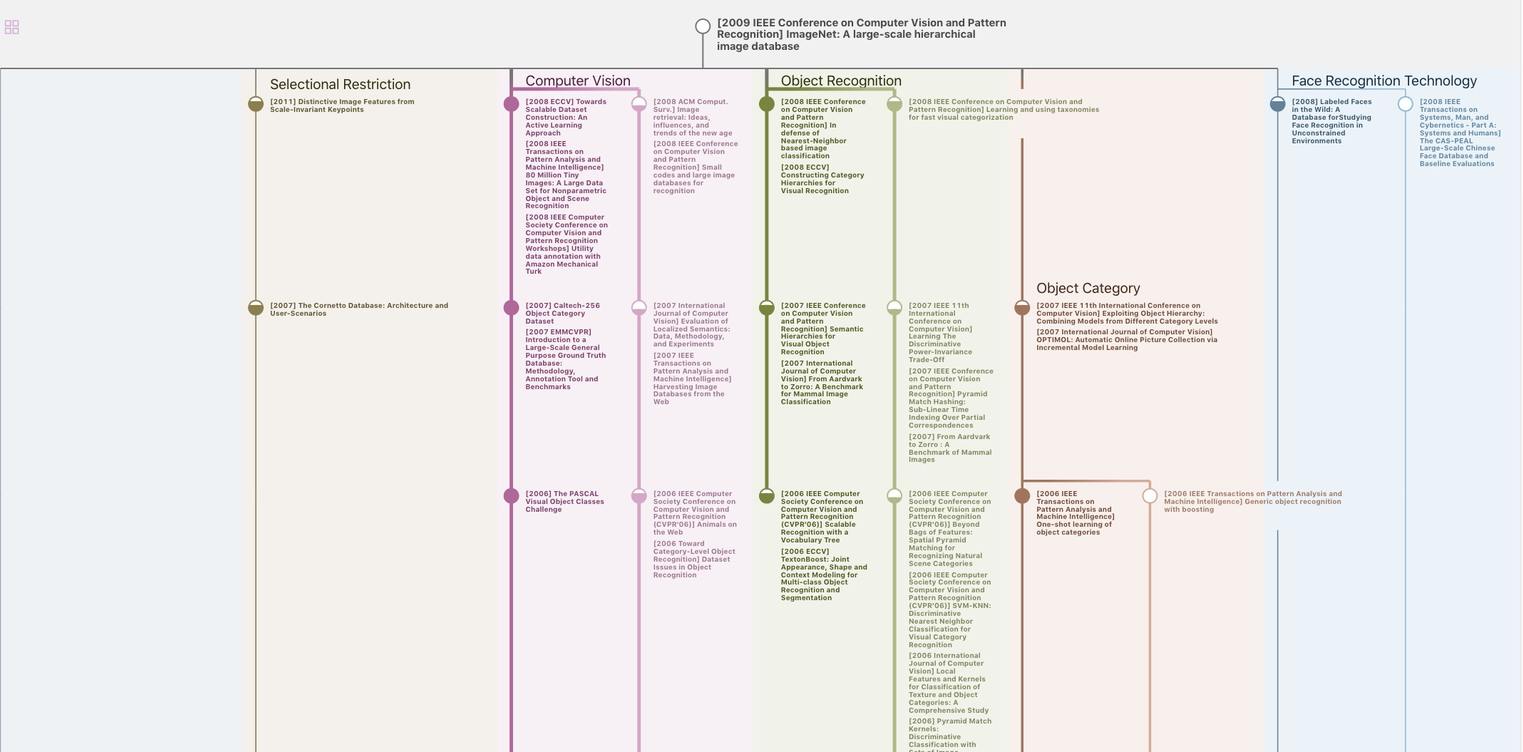
生成溯源树,研究论文发展脉络
Chat Paper
正在生成论文摘要