3D Human Action Representation Learning via Cross-View Consistency Pursuit
2021 IEEE/CVF CONFERENCE ON COMPUTER VISION AND PATTERN RECOGNITION, CVPR 2021(2021)
摘要
In this work, we propose a Cross-view Contrastive Learning framework for unsupervised 3D skeleton-based action Representation (CrosSCLR), by leveraging multiview complementary supervision signal. CrosSCLR consists of both single-view contrastive learning (Skeleton-CLR) and cross-view consistent knowledge mining (CVC-KM) modules, integrated in a collaborative learning manner. It is noted that CVC-KM works in such a way that high-confidence positive/negative samples and their distributions are exchanged among views according to their embedding similarity, ensuring cross-view consistency in terms of contrastive context, i.e., similar distributions. Extensive experiments show that CrosSCLR achieves remarkable action recognition results on NTU-60 and NTU-120 dmasets under unsupervised settings, with observed higherquality action representations. Our code is available at https://github.com/Linguoli/CrosSCLR.
更多查看译文
关键词
single-view contrastive learning,Skeleton-CLR,cross-view consistent knowledge mining modules,CVC-KM,collaborative learning manner,contrastive context,action recognition,observed higher-quality action representations,3D human action representation learning,cross-view consistency pursuit,cross-view contrastive learning framework,unsupervised 3D skeleton-based action representation,multiview complementary supervision signal
AI 理解论文
溯源树
样例
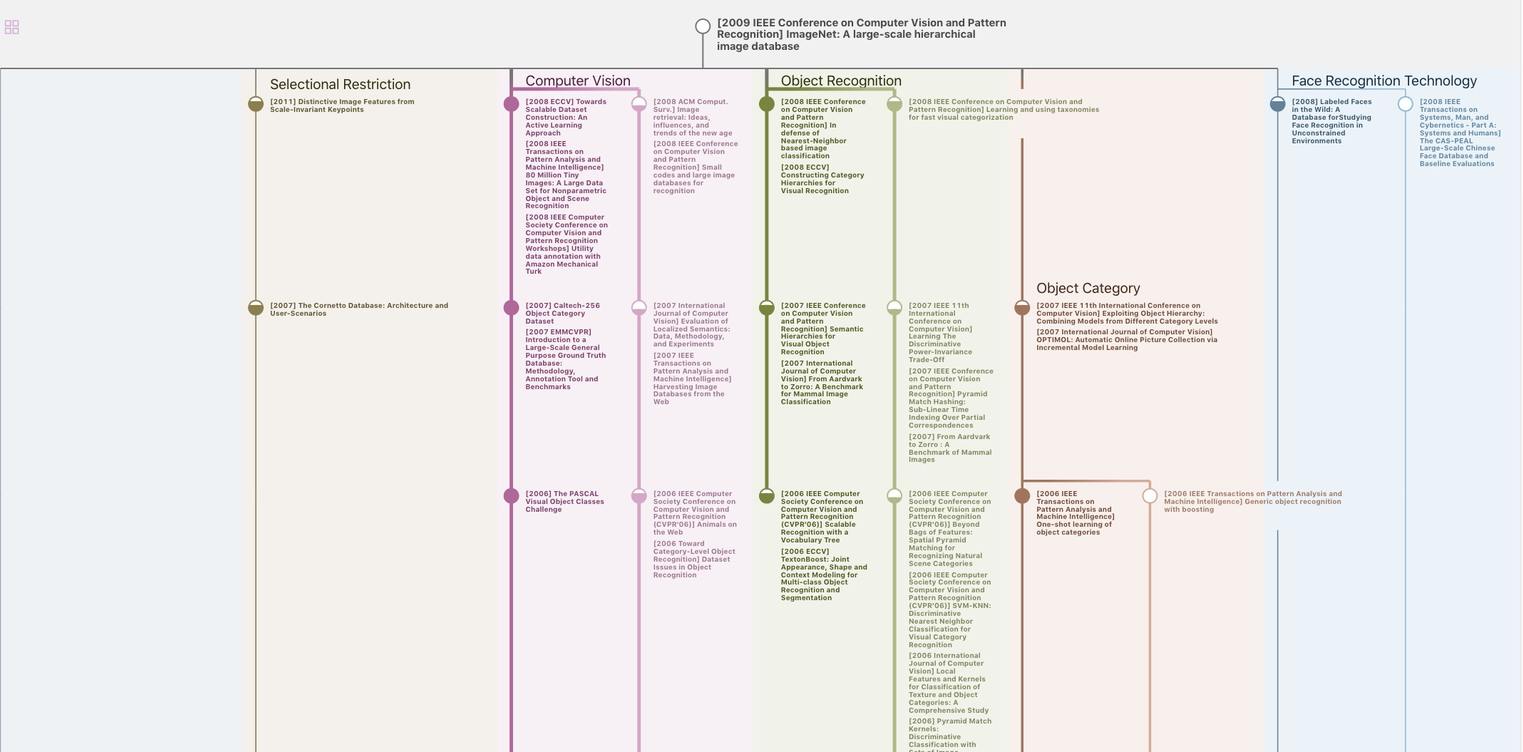
生成溯源树,研究论文发展脉络
Chat Paper
正在生成论文摘要