Progressive Stage-wise Learning for Unsupervised Feature Representation Enhancement
2021 IEEE/CVF CONFERENCE ON COMPUTER VISION AND PATTERN RECOGNITION, CVPR 2021(2021)
摘要
Unsupervised learning methods have recently shown their competitiveness against supervised training. Typically, these methods use a single objective to train the en-tire network. But one distinct advantage of unsupervised over supervised learning is that the former possesses more variety and freedom in designing the objective. In this work, we explore new dimensions of unsupervised learning by proposing the Progressive Stage-wise Learning (PSL) framework. For a given unsupervised task, we design multi-level tasks and define different learning stages for the deep network. Early learning stages are forced to focus on low-level tasks while late stages are guided to extract deeper information through harder tasks. We discover that by progressive stage-wise learning, unsupervised feature representation can be effectively enhanced. Our extensive experiments show that PSL consistently improves results for the leading unsupervised learning methods.
更多查看译文
关键词
unsupervised feature representation,enhancement,stage-wise
AI 理解论文
溯源树
样例
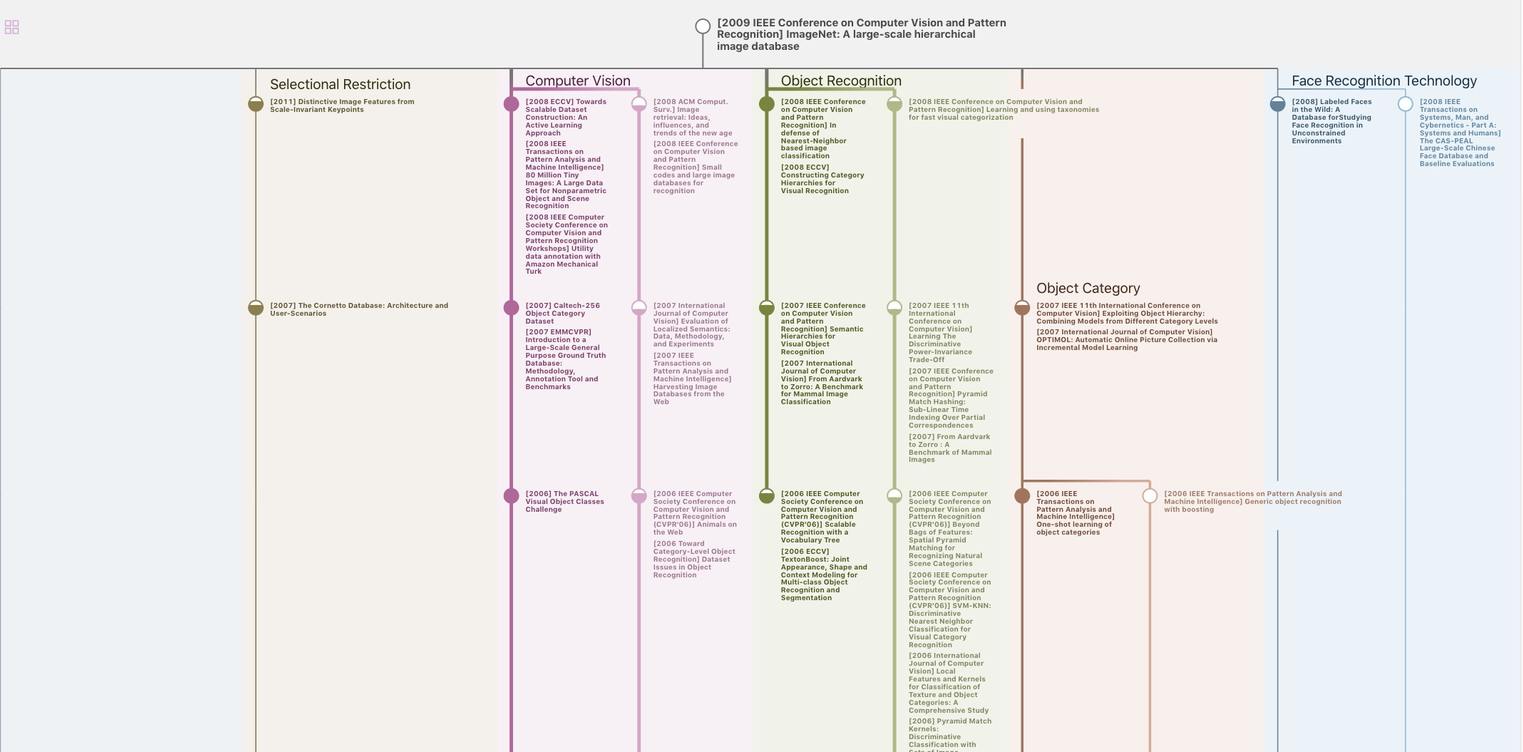
生成溯源树,研究论文发展脉络
Chat Paper
正在生成论文摘要