Coarse-to-Fine Semantic Alignment for Cross-Modal Moment Localization
IEEE TRANSACTIONS ON IMAGE PROCESSING(2021)
摘要
Video moment localization, as an important branch of video content analysis, has attracted extensive attention in recent years. However, it is still in its infancy due to the following challenges: cross-modal semantic alignment and localization efficiency. To address these impediments, we present a cross-modal semantic alignment network. To be specific, we first design a video encoder to generate moment candidates, learn their representations, as well as model their semantic relevance. Meanwhile, we design a query encoder for diverse query intention understanding. Thereafter, we introduce a multi-granularity interaction module to deeply explore the semantic correlation between multi-modalities. Thereby, we can effectively complete target moment localization via sufficient cross-modal semantic understanding. Moreover, we introduce a semantic pruning strategy to reduce cross-modal retrieval overhead, improving localization efficiency. Experimental results on two benchmark datasets have justified the superiority of our model over several state-of-the-art competitors.
更多查看译文
关键词
Semantics, Location awareness, Visualization, Context modeling, Proposals, Task analysis, Correlation, Cross-modal moment localization, coarse-to-fine semantic alignment, hierarchical semantic pruning
AI 理解论文
溯源树
样例
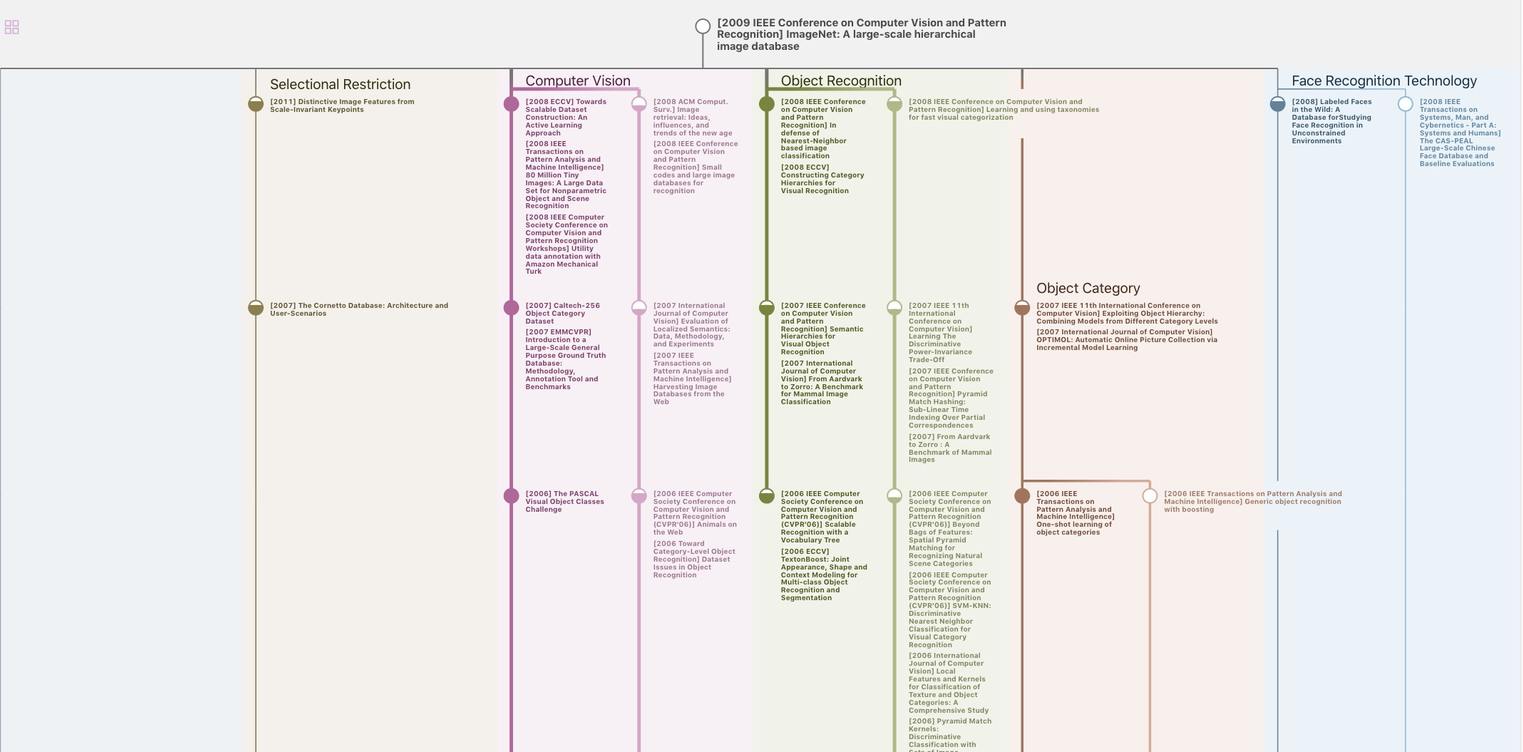
生成溯源树,研究论文发展脉络
Chat Paper
正在生成论文摘要