Mis-spoke or mis-lead: Achieving Robustness in Multi-Agent Communicative Reinforcement Learning.
International Joint Conference on Autonomous Agents and Multi-agent Systems(2022)
摘要
Recent studies in multi-agent communicative reinforcement learning (MACRL) demonstrate that multi-agent coordination can be significantly improved when communication between agents is allowed. Meanwhile, advances in adversarial machine learning (ML) have shown that ML and reinforcement learning (RL) models are vulnerable to a variety of attacks that significantly degrade the performance of learned behaviours. However, despite the obvious and growing importance, the combination of adversarial ML and MACRL remains largely uninvestigated. In this paper, we make the first step towards conducting message attacks on MACRL methods. In our formulation, one agent in the cooperating group is taken over by an adversary and can send malicious messages to disrupt a deployed MACRL-based coordinated strategy during the deployment phase. We further our study by developing a defence method via message reconstruction. Finally, we address the resulting arms race, i.e., we consider the ability of the malicious agent to adapt to the changing and improving defensive communicative policies of the benign agents. Specifically, we model the adversarial MACRL problem as a two-player zero-sum game and then utilize Policy-Space Response Oracle to achieve communication robustness. Empirically, we demonstrate that MACRL methods are vulnerable to message attacks while our defence method the game-theoretic framework can effectively improve the robustness of MACRL.
更多查看译文
关键词
reinforcement,robustness,learning,mis-spoke,mis-lead,multi-agent
AI 理解论文
溯源树
样例
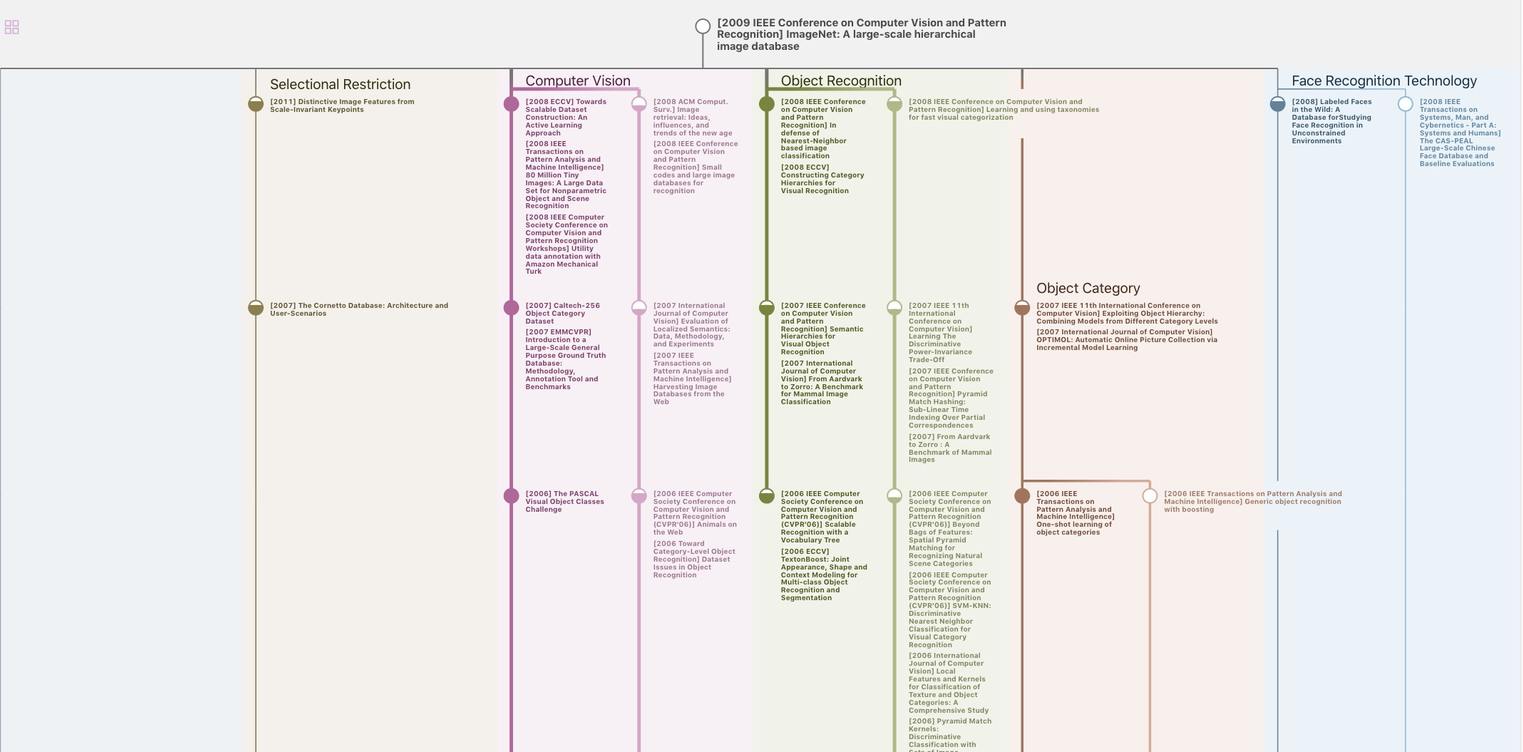
生成溯源树,研究论文发展脉络
Chat Paper
正在生成论文摘要