Improving Pneumonia Localization Via Cross-Attention on Medical Images and Reports.
CoRR(2021)
摘要
Localization and characterization of diseases like pneumonia are primary steps in a clinical pipeline, facilitating detailed clinical diagnosis and subsequent treatment planning. Additionally, such location annotated datasets can provide a pathway for deep learning models to be used for downstream tasks. However, acquiring quality annotations is expensive on human resources and usually requires domain expertise. On the other hand, medical reports contain a plethora of information both about pnuemonia characteristics and its location. In this paper, we propose a novel weakly-supervised attention-driven deep learning model that leverages encoded information in medical reports during training to facilitate better localization. Our model also performs classification of attributes that are associated to pneumonia and extracted from medical reports for supervision. Both the classification and localization are trained in conjunction and once trained, the model can be utilized for both the localization and characterization of pneumonia using only the input image. In this paper, we explore and analyze the model using chest X-ray datasets and demonstrate qualitatively and quantitatively that the introduction of textual information improves pneumonia localization. We showcase quantitative results on two datasets, MIMIC-CXR and Chest X-ray-8, and we also showcase severity characterization on COVID-19 dataset.
更多查看译文
关键词
Pneumonia localization,Cross-attention,Multi-modal system
AI 理解论文
溯源树
样例
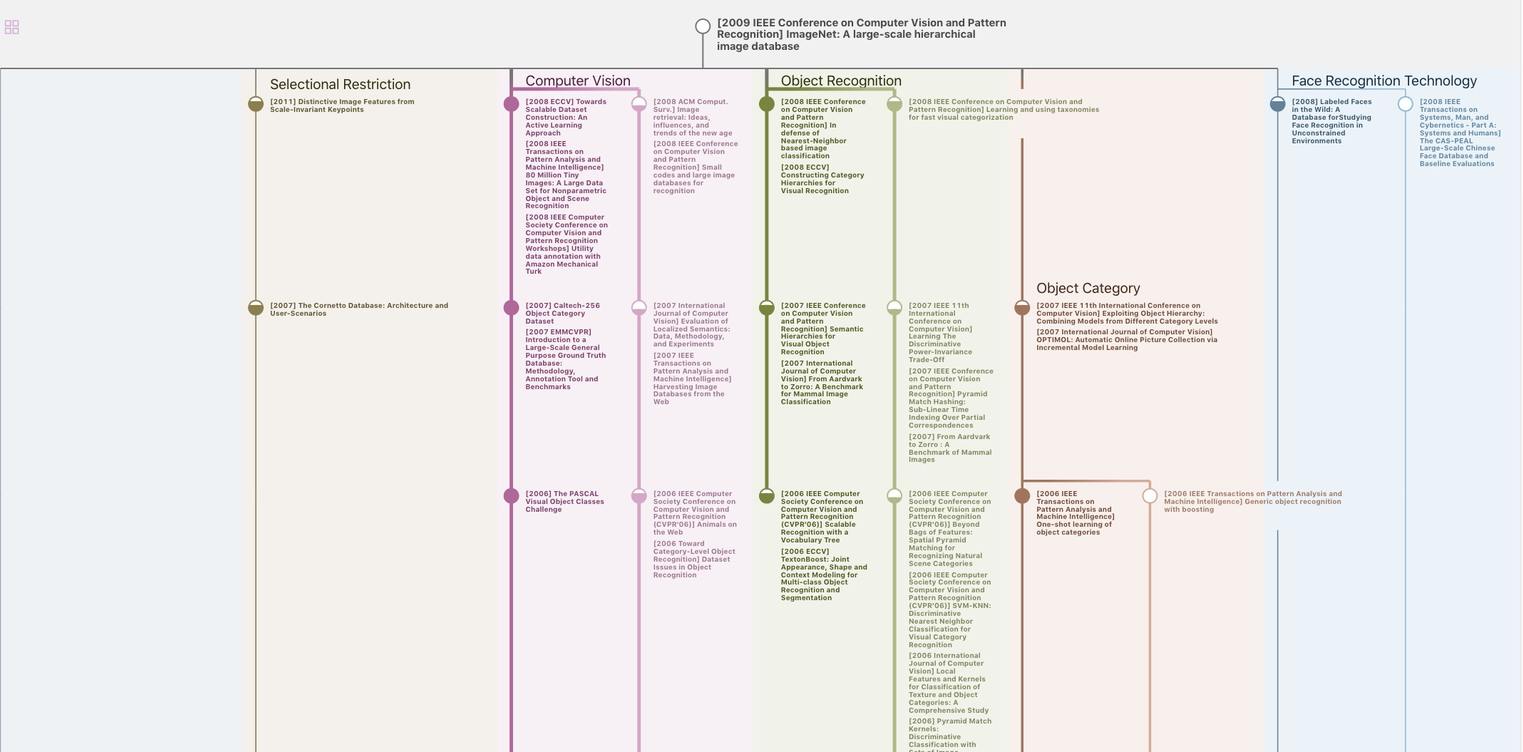
生成溯源树,研究论文发展脉络
Chat Paper
正在生成论文摘要