Improving Signal to Noise Ratios in Ion Mobility Spectrometry and Structures for Lossless Ion Manipulations (SLIM) using a High Dynamic Range Analog-to-Digital Converter
JOURNAL OF THE AMERICAN SOCIETY FOR MASS SPECTROMETRY(2021)
摘要
Signal digitization is a commonly overlooked part of ion mobility-mass spectrometry (IMS-MS) workflows, yet it greatly affects signalto-noise ratio and MS resolution measurements. Here, we report on the integration of a 2 GS/s, 14-bit ADC with structures for lossless ion manipulations (SLIM-IMS-MS) and compare the performance to a commonly used 8-bit ADC. The 14-bit ADC provided a reduction in the digitized noise by a factor of similar to 6, owing largely to the use of smaller bit sizes. The low baseline allowed threshold voltage levels to be set very close to the MCP baseline voltage, allowing for as much signal to be acquired as possible without overloading or excessive digitization of MCP baseline noise. Analyses of Agilent tuning mixture ions and a mixture of heavy labeled phosphopeptides showed that the 14-bit ADC provided a similar to 1.5-2x signal-to-noise (S/N) increase for high intensity ions, such as the Agilent tuning mixture ions and the 2+ and 3+ charge states of many phosphopeptide constituents. However, signal enhancements were as much as 10-fold for low intensity ions, and the 14-bit ADC enabled discernible signal intensities otherwise lost using an 8-bit digitizer. Additionally, the 14-bit ADC required similar to 14-fold fewer mass spectra to be averaged to produce a mass spectrum with a similar S/N as the 8-bit ADC, demonstrating similar to 10x higher measurement throughput. The high resolution, low baseline, and fast speed of the new 14-bit ADC enables high performance digitization of MS, IMS-MS, and SLIM-IMS-MS spectra and provides a much better picture of analyte profiles in complex mixtures.
更多查看译文
关键词
data acquisition, digitization, ion mobility spectrometry (IMS), mass spectrometry (MS), signal-to-noise (S/N), structures for lossless ion manipulations (SLIM), ultrahigh resolution
AI 理解论文
溯源树
样例
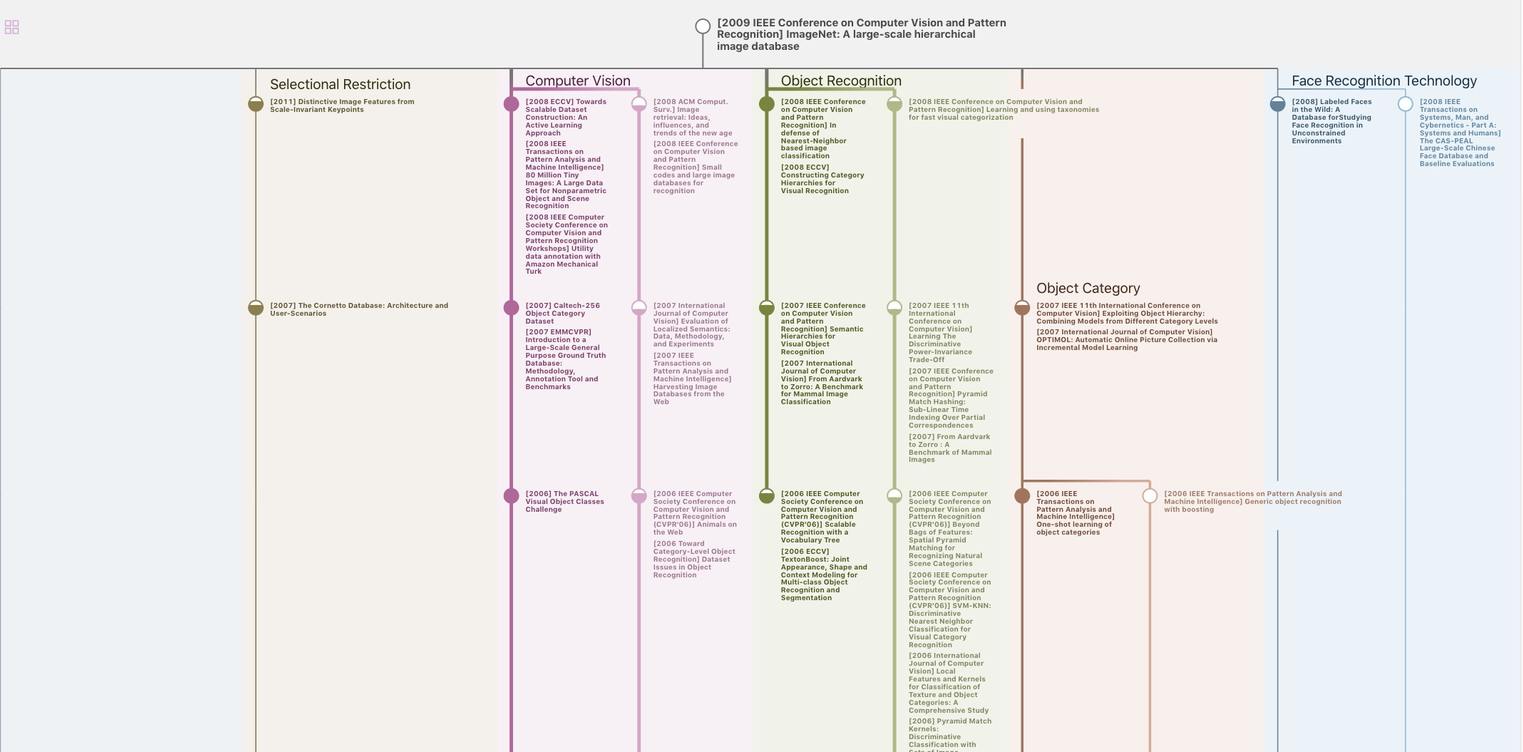
生成溯源树,研究论文发展脉络
Chat Paper
正在生成论文摘要