Large-Scale ASR Domain Adaptation Using Self- and Semi-Supervised Learning
arxiv(2022)
摘要
Self- and semi-supervised learning methods have been actively investigated to reduce labeled training data or enhance model performance. However, these approaches mostly focus on in-domain performance for public datasets. In this study, we utilize the combination of self- and semi-supervised learning methods to solve unseen domain adaptation problems in a large-scale production setting for online ASR model. This approach demonstrates that using the source domain data with a small fraction of the target domain data (3%) can recover the performance gap compared to a full data baseline: 13.5% relative WER improvement for target domain data.
更多查看译文
关键词
speech recognition,domain adaptation,self-supervised learning,semi-supervised learning,RNN-T
AI 理解论文
溯源树
样例
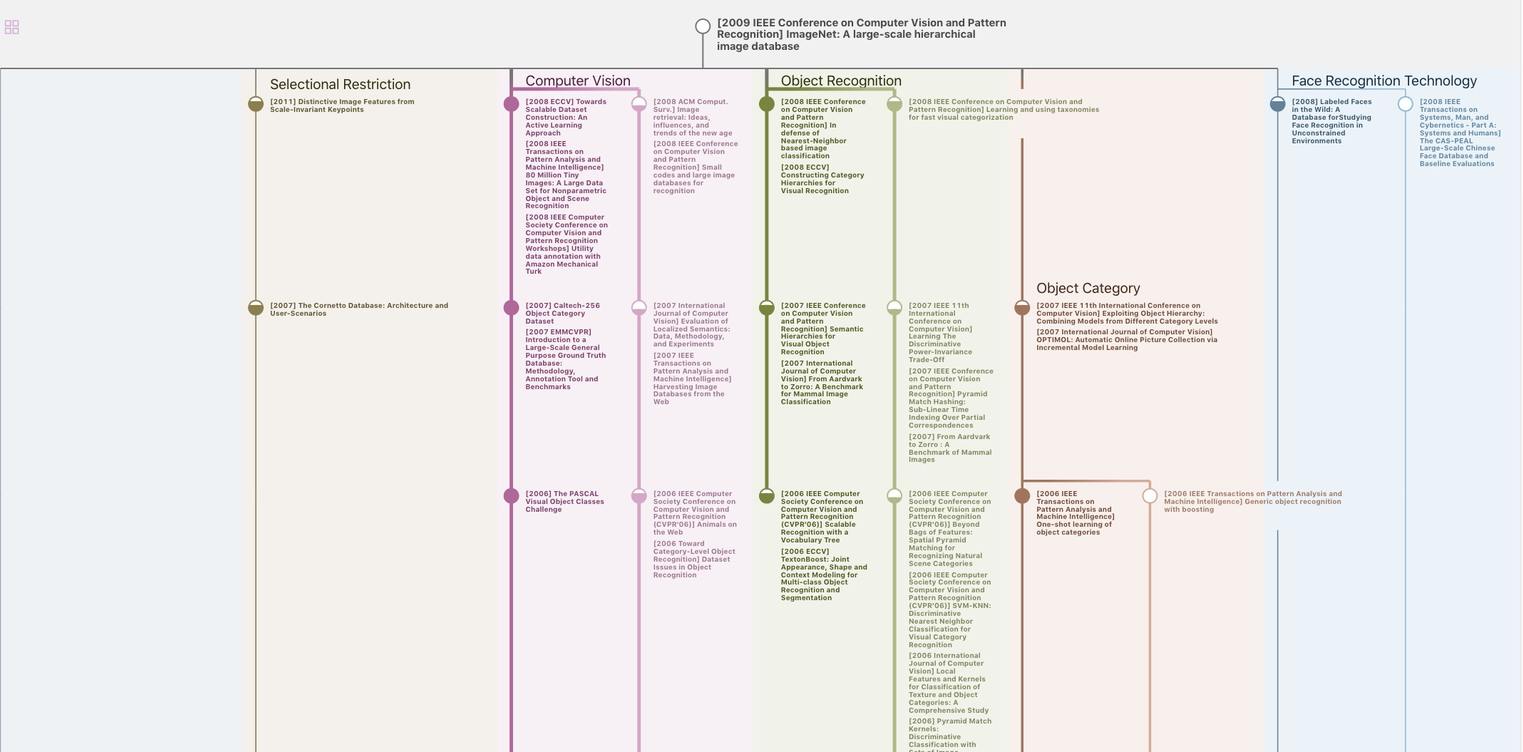
生成溯源树,研究论文发展脉络
Chat Paper
正在生成论文摘要