Fast Reconstruction for Large Disk Enclosures Based on RAID2.0
50TH INTERNATIONAL CONFERENCE ON PARALLEL PROCESSING(2021)
关键词
RAID,RAID2.0,Disk Failure,Reconstruction
AI 理解论文
溯源树
样例
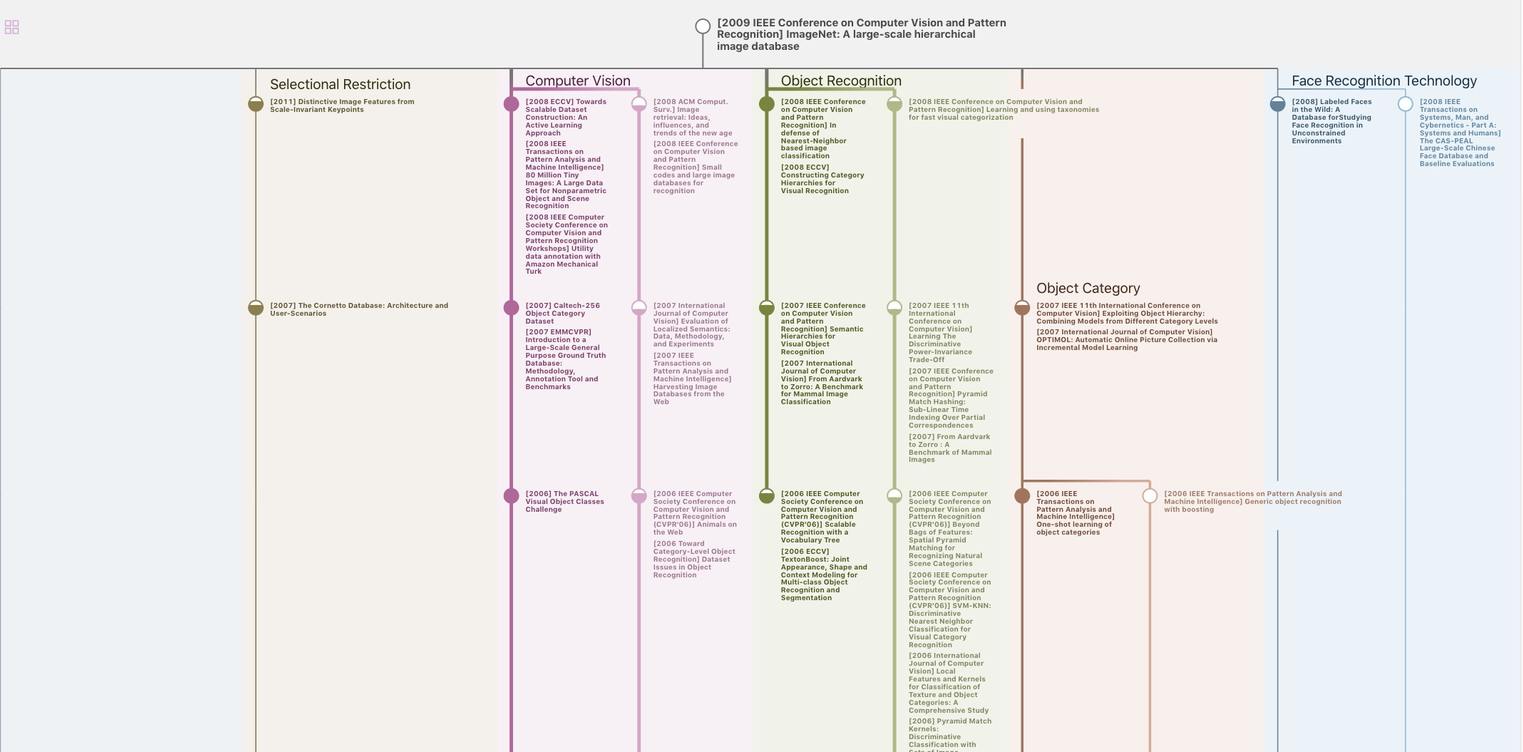
生成溯源树,研究论文发展脉络
Chat Paper
正在生成论文摘要
50TH INTERNATIONAL CONFERENCE ON PARALLEL PROCESSING(2021)