HDPL: a hybrid descriptor for points and lines based on graph neural networks
INDUSTRIAL ROBOT-THE INTERNATIONAL JOURNAL OF ROBOTICS RESEARCH AND APPLICATION(2021)
摘要
Purpose - This paper aims to design a novel feature descriptor to improve the performance of feature matching in challenge scenes, such as low texture and wide-baseline scenes. Common descriptors are not suitable for low texture scenes and other challenging scenes mainly owing to encoding only one kind of features. The proposed feature descriptor considers multiple features and their locations, which is more expressive. Design/methodology/approach - A graph neural network-based descriptors enhancement algorithm for feature matching is proposed. In this paper, point and line features are the primary concerns. In the graph, commonly used descriptors for points and lines constitute the nodes and the edges are determined by the geometric relationship between points and lines. After the graph convolution designed for incomplete join graph, enhanced descriptors are obtained. Findings - Experiments are carried out in indoor, outdoor and low texture scenes. The experiments investigate the real-time performance, rotation invariance, scale invariance, viewpoint invariance and noise sensitivity of the descriptors in three types of scenes. The results show that the enhanced descriptors are robust to scene changes and can be used in wide-baseline matching. Originality/value - A graph structure is designed to represent multiple features in an image. In the process of building graph structure, the geometric relation between multiple features is used to establish the edges. Furthermore, a novel hybrid descriptor for points and lines is obtained using graph convolutional neural network. This enhanced descriptor has the advantages of both point features and line features in feature matching.
更多查看译文
关键词
Multiple features, Hybrid descriptors, Graph neural networks, Feature matching, Challenging scenes
AI 理解论文
溯源树
样例
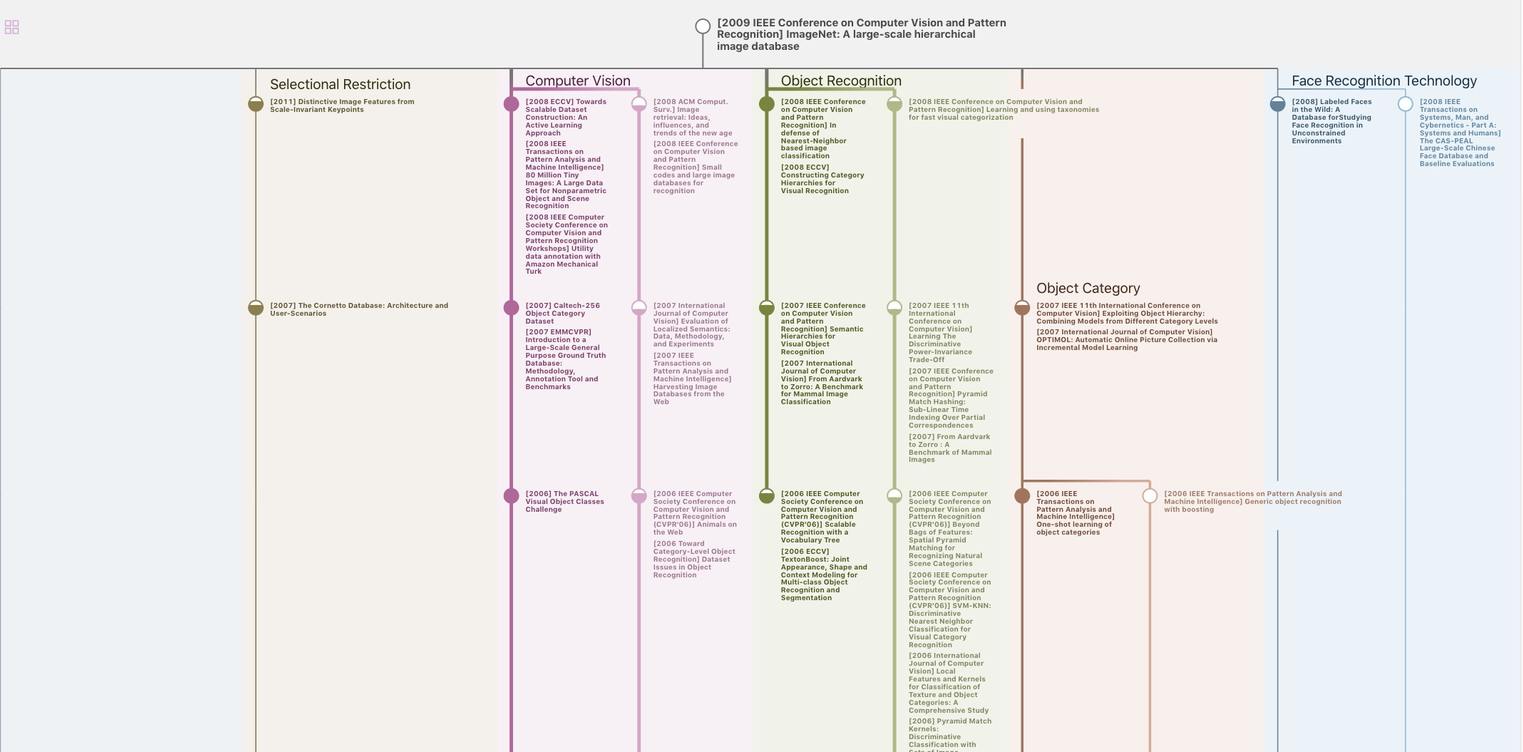
生成溯源树,研究论文发展脉络
Chat Paper
正在生成论文摘要