Random Feature Approximation for Online Nonlinear Graph Topology Identification
2021 IEEE 31st International Workshop on Machine Learning for Signal Processing (MLSP)(2021)
关键词
Nonlinear topology identification,Causality,Directed graphs,Kernels,Random feature approximation
AI 理解论文
溯源树
样例
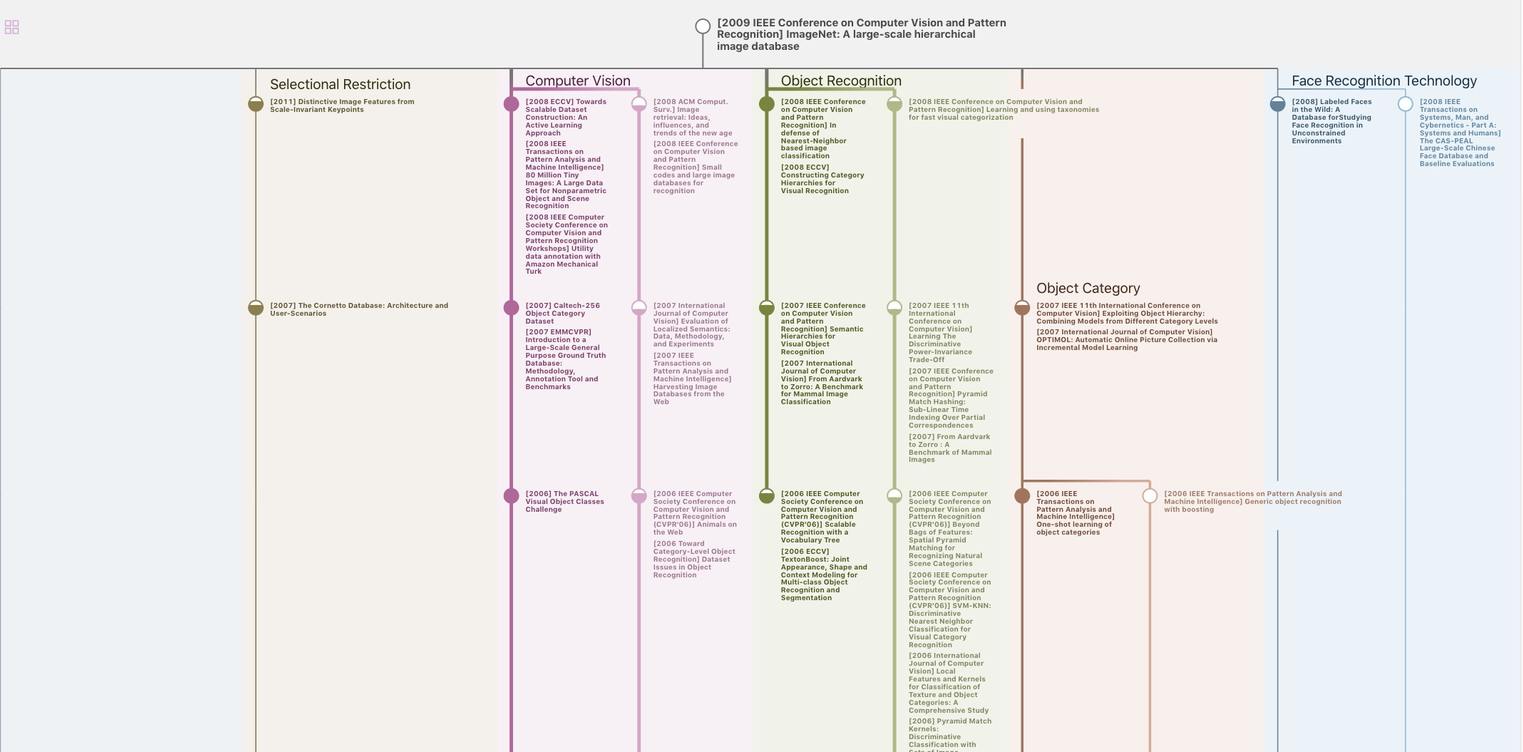
生成溯源树,研究论文发展脉络
Chat Paper
正在生成论文摘要