Enhancing Context-Based Meta-Reinforcement Learning Algorithms Via An Efficient Task Encoder (Student Abstract)
THIRTY-FIFTH AAAI CONFERENCE ON ARTIFICIAL INTELLIGENCE, THIRTY-THIRD CONFERENCE ON INNOVATIVE APPLICATIONS OF ARTIFICIAL INTELLIGENCE AND THE ELEVENTH SYMPOSIUM ON EDUCATIONAL ADVANCES IN ARTIFICIAL INTELLIGENCE(2021)
摘要
Meta-Reinforcement Learning (meta-RL) algorithms enable agents to adapt to new tasks from small amounts of exploration, based on the experience of similar tasks. Recent studies have pointed out that a good representation of a task is key to the success of off-policy context-based meta-RL. Inspired by contrastive methods in unsupervised representation learning, we propose a new method to learn the task representation based on the mutual information between transition tuples in a trajectory and the task embedding. We also propose a new estimation for task similarity based on Q-function, which can be used to form a constraint on the distribution of the encoded task variables, making the task encoder encode the task variables more effective on new tasks. Experiments on meta-RL tasks show that the newly proposed method outperforms existing meta-RL algorithms.
更多查看译文
AI 理解论文
溯源树
样例
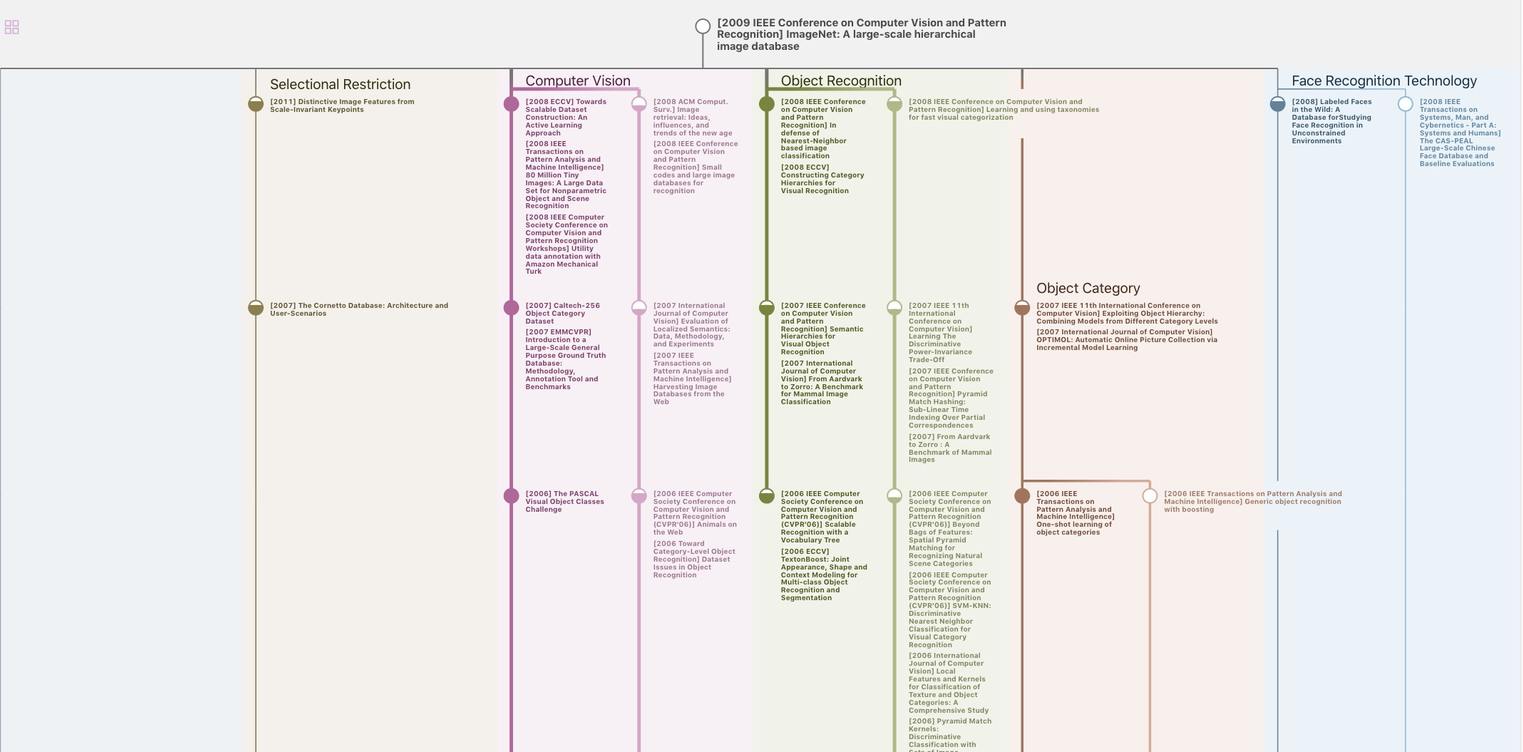
生成溯源树,研究论文发展脉络
Chat Paper
正在生成论文摘要