Improving Model Predictive Path Integral using Covariance Steering
ArXiv(2021)
摘要
This paper presents a novel control approach for autonomous systems operating under uncertainty. We combine Model Predictive Path Integral (MPPI) control with Covariance Steering (CS) theory to obtain a robust controller for general nonlinear systems. The proposed Covariance-Controlled Model Predictive Path Integral (CC-MPPI) controller addresses the performance degradation observed in some MPPI implementations owing to unexpected disturbances and uncertainties. Namely, in cases where the environment changes too fast or the simulated dynamics during the MPPI rollouts do not capture the noise and uncertainty in the actual dynamics, the baseline MPPI implementation may lead to divergence. The proposed CC-MPPI controller avoids divergence by controlling the dispersion of the rollout trajectories at the end of the prediction horizon. Furthermore, the CC-MPPI has adjustable trajectory sampling distributions that can be changed according to the environment to achieve efficient sampling. Numerical examples using a ground vehicle navigating in challenging environments demonstrate the proposed approach.
更多查看译文
关键词
model predictive path integral,covariance steering
AI 理解论文
溯源树
样例
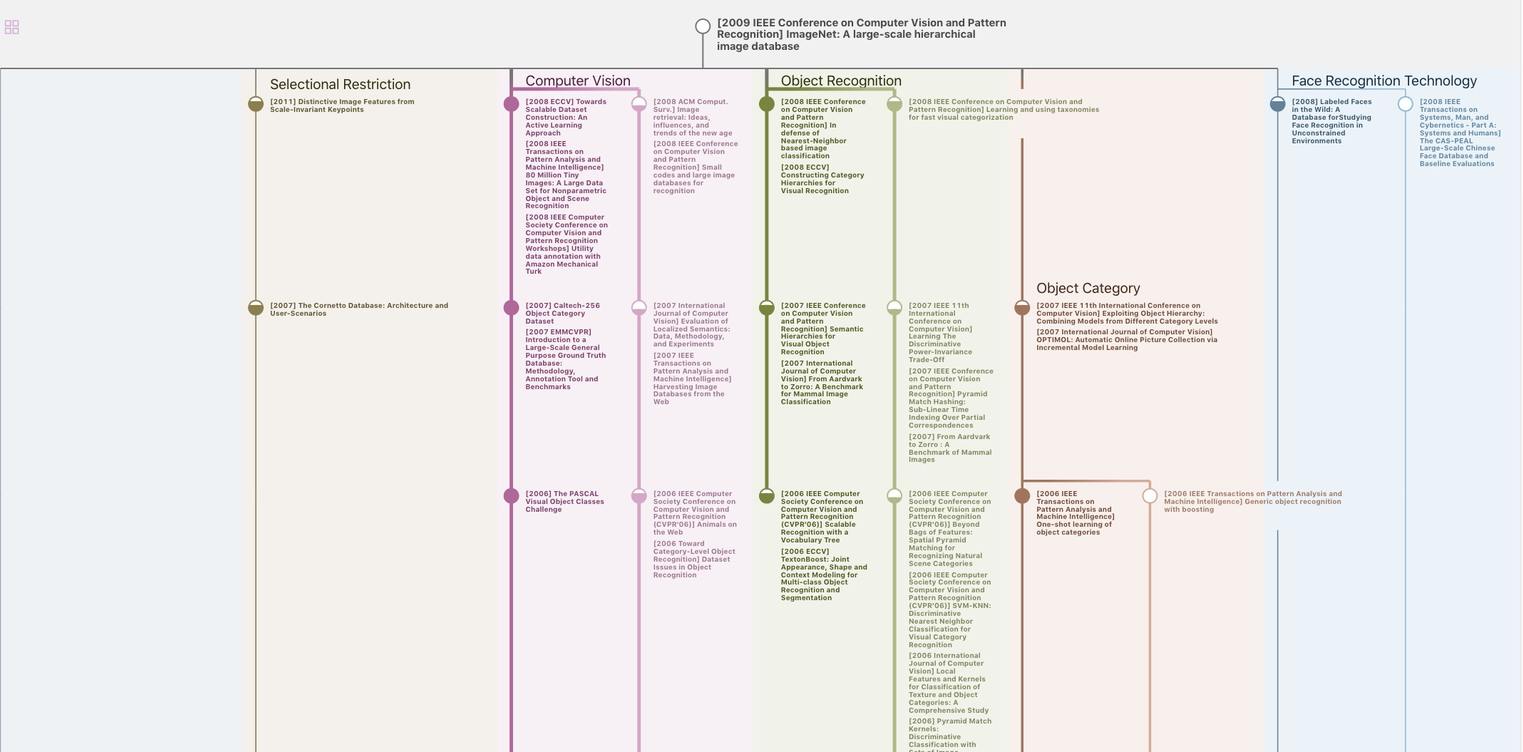
生成溯源树,研究论文发展脉络
Chat Paper
正在生成论文摘要