Image-Based Joint State Estimation Pipeline for Sensorless Manipulators
IEEE/RJS International Conference on Intelligent RObots and Systems (IROS)(2021)CCF C
Abstract
Motion planning is a largely solved problem for robot arms with joint state feedback, but remains an area of research for sensorless manipulators such as toy robot arms and heavy equipment such as excavators and cranes. A promising approach to this problem is deep learning, which employs a pre-trained convolutional neural network to identify manipulator links and estimate joint states from a monocular camera video feed. Whereas manual labeling of training image sets is tedious and non-transferable, a simulation environment can automatically generate labeled training image sets of any size. The issue is the gap between simulated and real-world images. This paper solves this problem by implementing a Generative Adversarial Network. The complete joint state estimation pipeline is implemented and tested in hardware experiments to validate our proposed approach.
MoreTranslated text
Key words
joint state feedback,sensorless manipulators,toy robot arms,heavy equipment,excavators,cranes,deep learning,pre-trained convolutional neural network,manipulator links,monocular camera video feed,manual labeling,simulation environment,labeled training image sets,real-world images,generative adversarial network,motion planning,joint state estimation pipeline
求助PDF
上传PDF
View via Publisher
AI Read Science
AI Summary
AI Summary is the key point extracted automatically understanding the full text of the paper, including the background, methods, results, conclusions, icons and other key content, so that you can get the outline of the paper at a glance.
Example
Background
Key content
Introduction
Methods
Results
Related work
Fund
Key content
- Pretraining has recently greatly promoted the development of natural language processing (NLP)
- We show that M6 outperforms the baselines in multimodal downstream tasks, and the large M6 with 10 parameters can reach a better performance
- We propose a method called M6 that is able to process information of multiple modalities and perform both single-modal and cross-modal understanding and generation
- The model is scaled to large model with 10 billion parameters with sophisticated deployment, and the 10 -parameter M6-large is the largest pretrained model in Chinese
- Experimental results show that our proposed M6 outperforms the baseline in a number of downstream tasks concerning both single modality and multiple modalities We will continue the pretraining of extremely large models by increasing data to explore the limit of its performance
Upload PDF to Generate Summary
Must-Reading Tree
Example
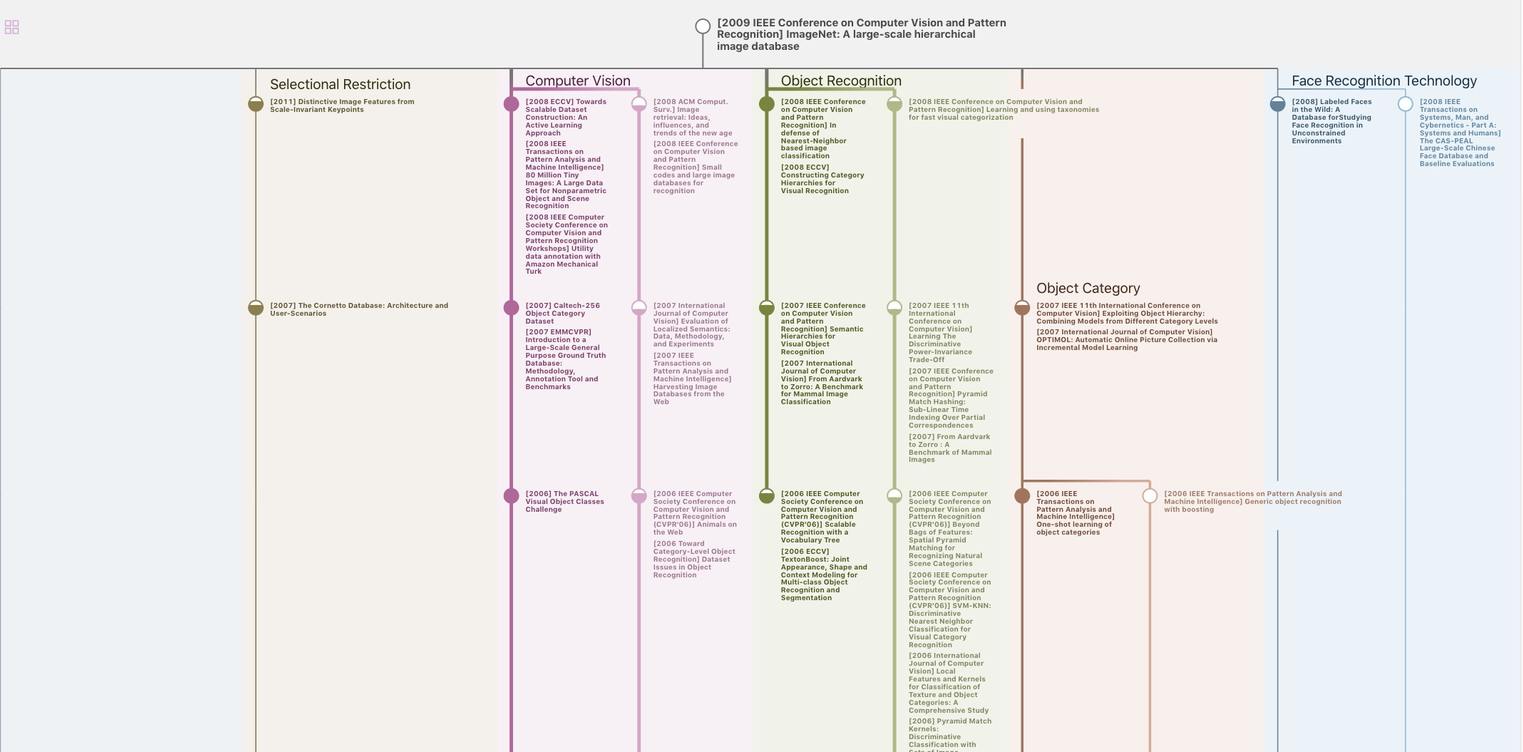
Generate MRT to find the research sequence of this paper
Related Papers
2014
被引用2939 | 浏览
2017
被引用455 | 浏览
2018
被引用55 | 浏览
2020
被引用152 | 浏览
Data Disclaimer
The page data are from open Internet sources, cooperative publishers and automatic analysis results through AI technology. We do not make any commitments and guarantees for the validity, accuracy, correctness, reliability, completeness and timeliness of the page data. If you have any questions, please contact us by email: report@aminer.cn
Chat Paper
GPU is busy, summary generation fails
Rerequest