Learning Differentiable Safety-Critical Control using Control Barrier Functions for Generalization to Novel Environments
2022 EUROPEAN CONTROL CONFERENCE (ECC)(2022)
摘要
Control barrier functions (CBFs) have become a popular tool to enforce safety of a control system. CBFs are commonly utilized in a quadratic program formulation (CBF-QP) as safety-critical constraints. A class K function in CBFs usually needs to be tuned manually in order to balance the trade-off between performance and safety for each environment. However, this process is often heuristic and can become intractable for high relative-degree systems. Moreover, it prevents the CBF-QP from generalizing to different environments in the real world. By embedding the optimization procedure of the exponential control barrier function based quadratic program (ECBF-QP) as a differentiable layer within a deep learning architecture, we propose a differentiable safety-critical control framework that enables generalization to new environments for high relative-degree systems with forward invariance guarantees. Finally, we validate the proposed control design with 2D double and quadruple integrator systems in various environments.
更多查看译文
关键词
control barrier functions,CBFs,control system,quadratic program formulation,safety-critical constraints,k function,relative-degree systems,CBF-QP,exponential control barrier function,differentiable safety-critical control framework,control design
AI 理解论文
溯源树
样例
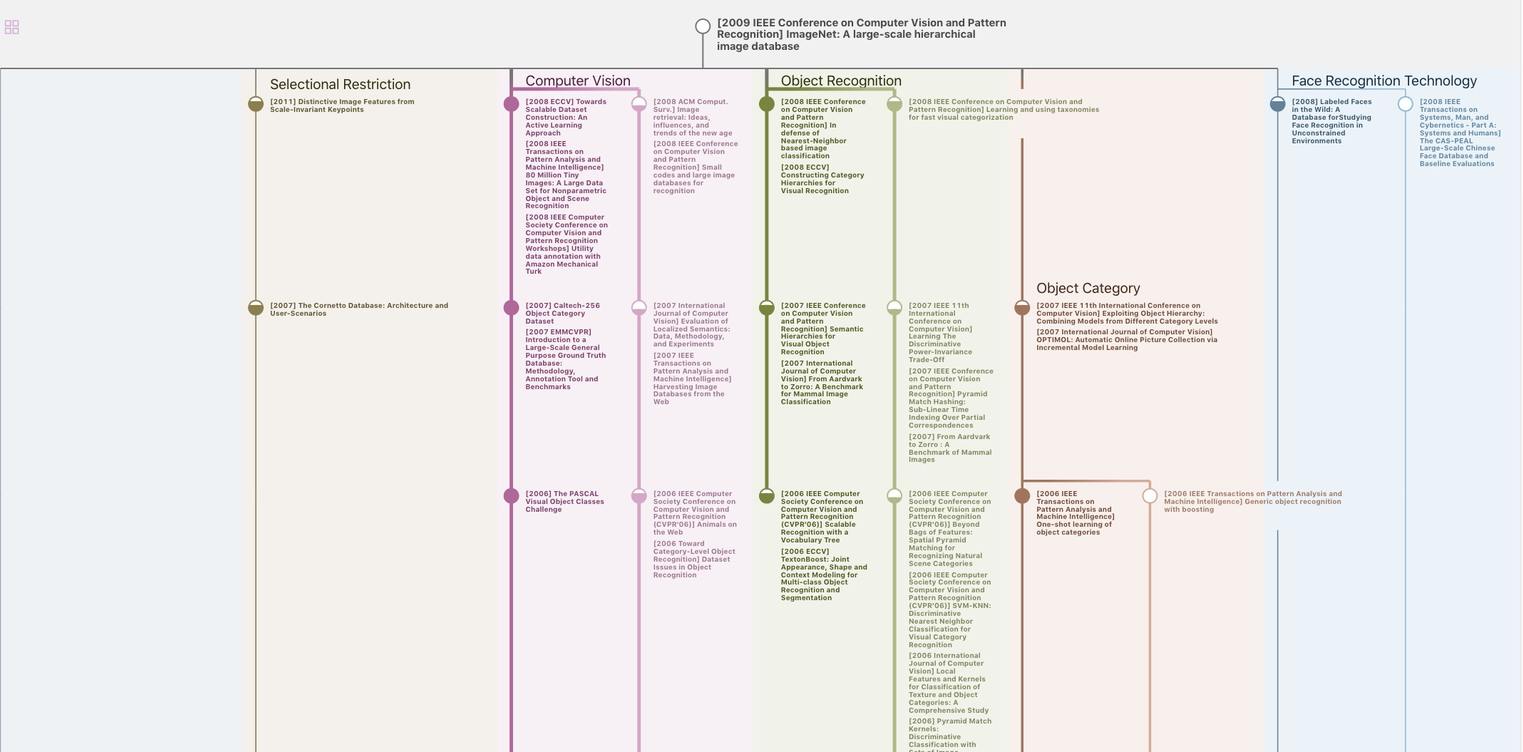
生成溯源树,研究论文发展脉络
Chat Paper
正在生成论文摘要