Near-Memory Processing in Action: Accelerating Personalized Recommendation With AxDIMM
IEEE Micro(2022)
Abstract
Near-memory processing (NMP) is a prospective paradigm enabling memory-centric computing. By moving the compute capability next to the main memory (DRAM modules), it can fundamentally address the CPU-memory bandwidth bottleneck and thus effectively improve the performance of memory-constrained workloads. Using the personalized recommendation system as a driving example, we developed a scalable, practical DIMM-based NMP solution tailor-designed for accelerating the inference serving. Our solution is demonstrated on a versatile FPGA-enabled NMP platform called AxDIMM that allows rapid prototyping and evaluation of NMP’s performance potential on real hardware under a realistic system setting using industry-representative recommendation framework. We experimentally validated the performance of a two-ranked AxDIMM prototype, which achieves up to 1.89× speedup in latency and 31.6% memory energy saving for embedding operations. For end-to-end recommendation inference serving, AxDIMM improves the throughput up to 1.5× and latency-bounded throughput up to 1.77×, respectively.
MoreTranslated text
Key words
personalized recommendation,axdimm,near-memory
AI Read Science
Must-Reading Tree
Example
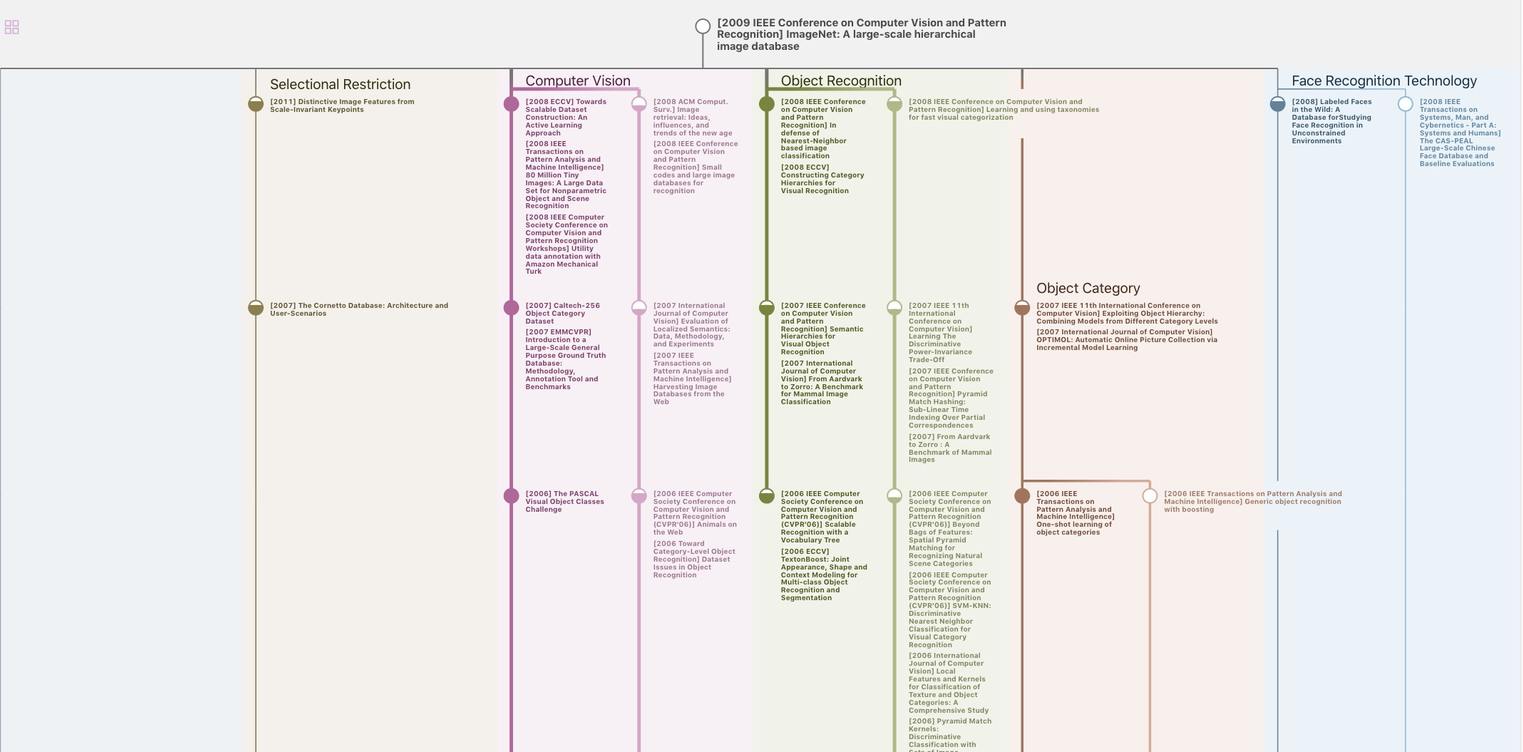
Generate MRT to find the research sequence of this paper
Chat Paper
Summary is being generated by the instructions you defined