Scenario Aware Speech Recognition: Advancements for Apollo Fearless Steps & CHiME-4 Corpora
2021 IEEE Automatic Speech Recognition and Understanding Workshop (ASRU)(2021)
摘要
In this study, we propose to investigate triplet loss for the purpose of an alternative feature representation for ASR. We consider a general non-semantic speech representation, which is trained with a self-supervised criteria based on triplet loss called TRILL, for acoustic modeling to represent the acoustic characteristics of each audio. This strategy is then applied to the CHiME-4 corpus and CRSS-UTDallas Fearless Steps Corpus, with emphasis on the 100-hour challenge corpus which consists of 5 selected NASA Apollo-11 channels. An analysis of the extracted embeddings provides the foundation needed to characterize training utterances into distinct groups based on acoustic distinguishing properties. Moreover, we also demonstrate that triplet-loss based embedding performs better than i-Vector in acoustic modeling, confirming that the triplet loss is more effective than a speaker feature. With additional techniques such as pronunciation and silence probability modeling, plus multi-style training, we achieve a +5.42% and +3.18% relative WER improvement for the development and evaluation sets of the Fearless Steps Corpus. To explore generalization, we further test the same technique on the 1 channel track of CHiME-4 and observe a +11.90% relative WER improvement for real test data.
更多查看译文
关键词
speech recognition,scenario aware,speech representation
AI 理解论文
溯源树
样例
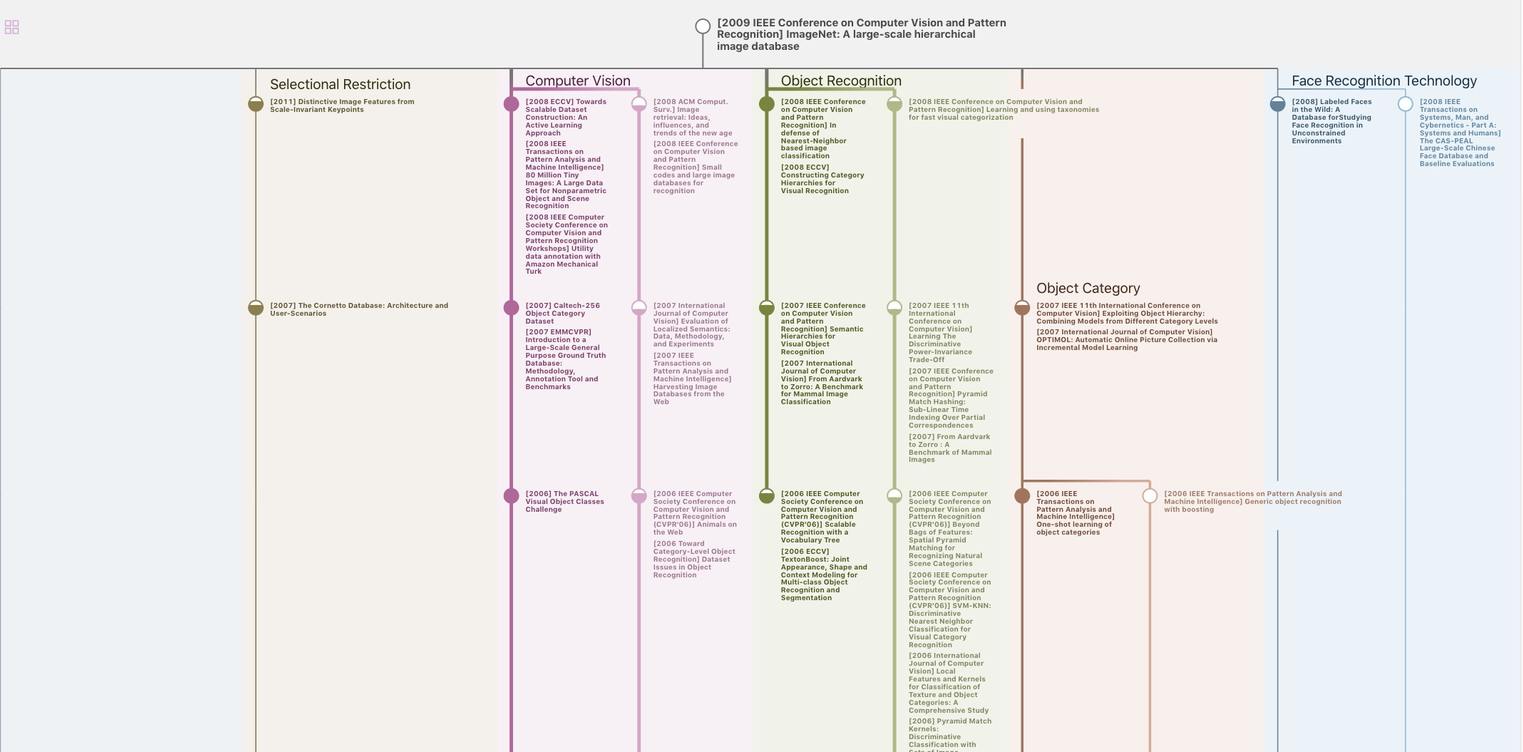
生成溯源树,研究论文发展脉络
Chat Paper
正在生成论文摘要