Weakly Supervised Correspondence Learning
2022 International Conference on Robotics and Automation (ICRA)(2022)
摘要
Correspondence learning is a fundamental problem in robotics, which aims to learn a mapping between state, action pairs of agents of different dynamics or embodiments. However, current correspondence learning methods either leverage strictly paired data-which are often difficult to collect-or learn in an unsupervised fashion from unpaired data using regularization techniques such as cycle-consistency-which suffer from severe misalignment issues. We propose a weakly supervised correspondence learning approach that trades off between strong supervision over strictly paired data and unsupervised learning with a regularizer over unpaired data. Our idea is to leverage two types of weak supervision: i) temporal ordering of states and actions to reduce the compounding error, and ii) paired abstractions, instead of paired data, to alleviate the misalignment problem and learn a more accurate correspondence. The two types of weak supervision are easy to access in real-world applications, which simultaneously reduces the high cost of annotating strictly paired data and improves the quality of the learned correspondence. We show the videos of the experiments on our website.
更多查看译文
关键词
learning
AI 理解论文
溯源树
样例
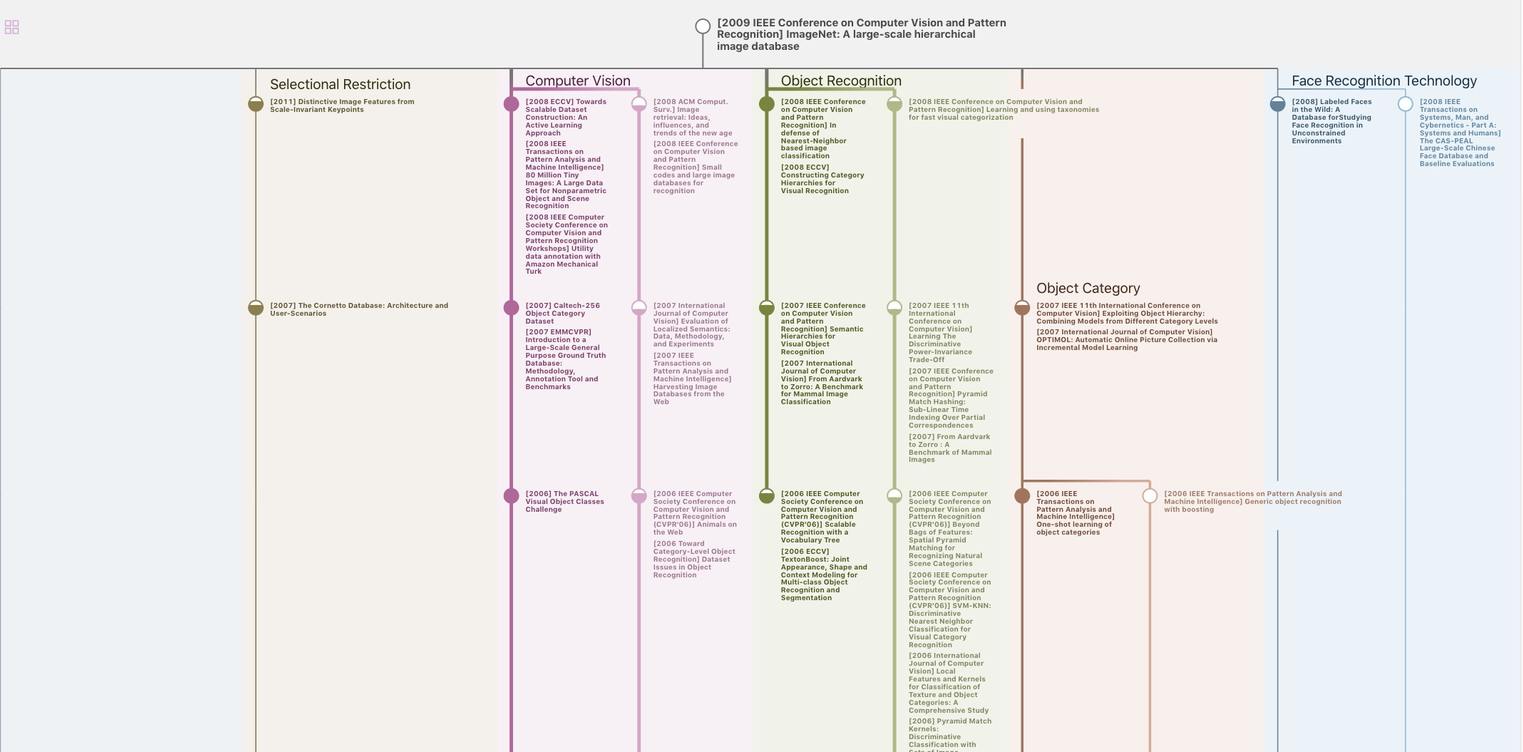
生成溯源树,研究论文发展脉络
Chat Paper
正在生成论文摘要