Unsupervised anomaly detection via dual transformation‐aware embeddings
IET Image Processing(2022)
摘要
Unsupervised anomaly detection refers to the discovery of unconventional images that are globally or locally different from the training set. Recently, reconstruction-based anomaly detection methods have made great progress. However, most of the existing methods take reconstructing the original image as the goal of latent feature learning. Due to lack of effective semantic guidance, latent features have intrinsic characteristics which retain redundant details of spatial structure. Such information is too general and cause over-expression problem. To solve this problem, in this paper, dual transformation-aware embeddings are coined which aims to achieve a stable model to learn high-level latent features in a self-supervised manner. To be more specific, the authors try to extract transformation-detectable feature embeddings for both structure and content views which explore the regular pattern under different transformations in normal situations. In addition, the relationship between the original feature and the transformed feature is established. Based on such relationship, the latent feature of generated image to predict transformation parameter is extracted. Then, a transformation-consistency regularization is proposed to constrain decoder to generate high-quality image with high-level consistency and achieve a more stable model. Experiments on MVTec-AD and CIFAR10 datasets prove the effectiveness and robustness of the proposed method.
更多查看译文
AI 理解论文
溯源树
样例
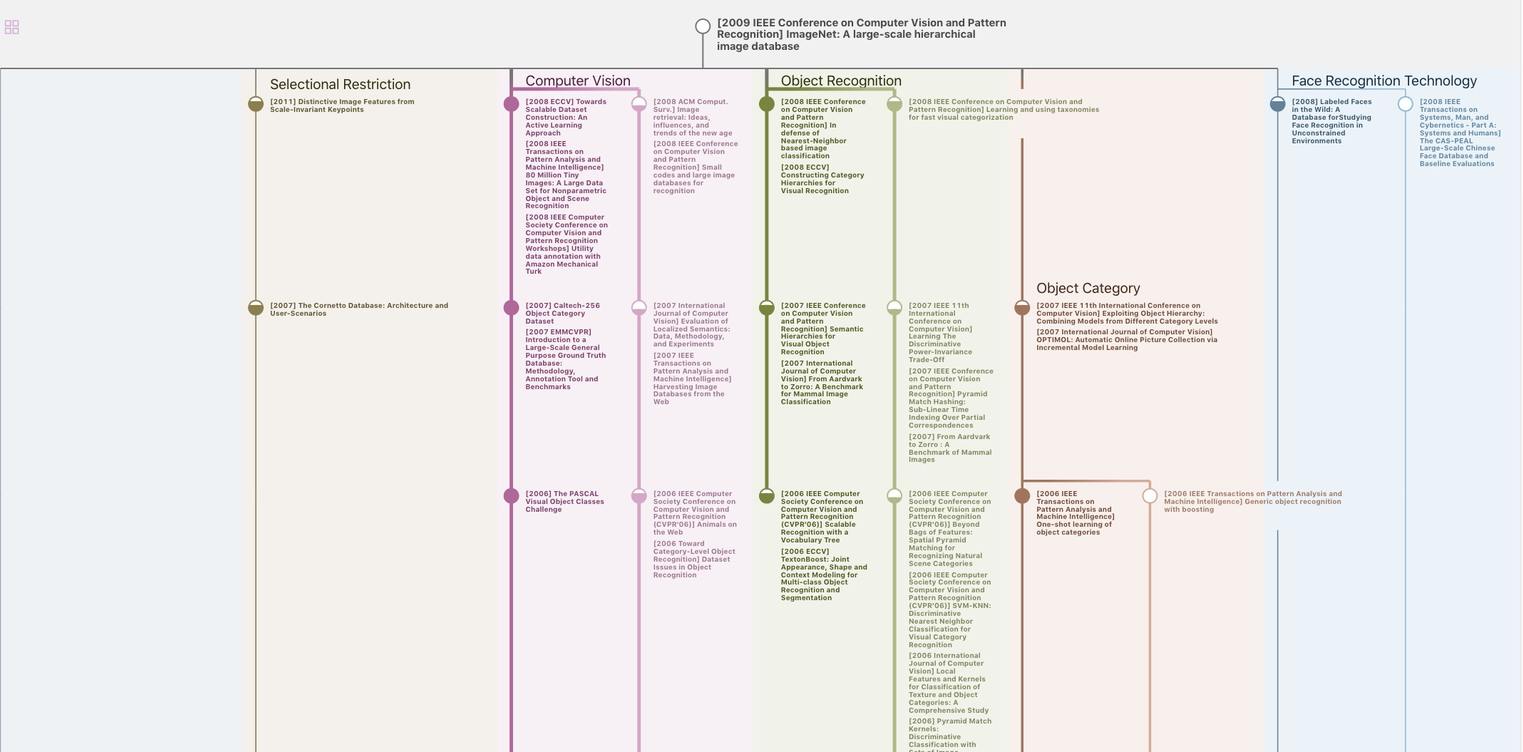
生成溯源树,研究论文发展脉络
Chat Paper
正在生成论文摘要