Enhancing Characteristic Gene Selection and Tumor Classification by the Robust Laplacian Supervised Discriminative Sparse PCA
JOURNAL OF CHEMICAL INFORMATION AND MODELING(2022)
摘要
Characteristic gene selection and tumor classification of gene expression data play major roles in genomic research. Due to the characteristics of a small sample size and high dimensionality of gene expression data, it is a common practice to perform dimensionality reduction prior to the use of machine learning-based methods to analyze the expression data. In this context, classical principal component analysis (PCA) and its improved versions have been widely used. Recently, methods based on supervised discriminative sparse PCA have been developed to improve the performance of data dimensionality reduction. However, such methods still have limitations: most of them have not taken into consideration the improvement of robustness to outliers and noise, label information, sparsity, as well as capturing intrinsic geometrical structures in one objective function. To address this drawback, in this study, we propose a novel PCA-based method, known as the robust Laplacian supervised discriminative sparse PCA, termed RLSDSPCA, which enforces the L2,1 norm on the error function and incorporates the graph Laplacian into supervised discriminative sparse PCA. To evaluate the efficacy of the proposed RLSDSPCA, we applied it to the problems of characteristic gene selection and tumor classification problems using gene expression data. The results demonstrate that the proposed RLSDSPCA method, when used in combination with other related methods, can effectively identify new pathogenic genes associated with diseases. In addition, RLSDSPCA has also achieved the best performance compared with the state-of-the-art methods on tumor classification in terms of major performance metrics. The codes and data sets used in the study are freely available at http://csbio.njust.edu.cn/bioinf/rlsdspca/.
更多查看译文
AI 理解论文
溯源树
样例
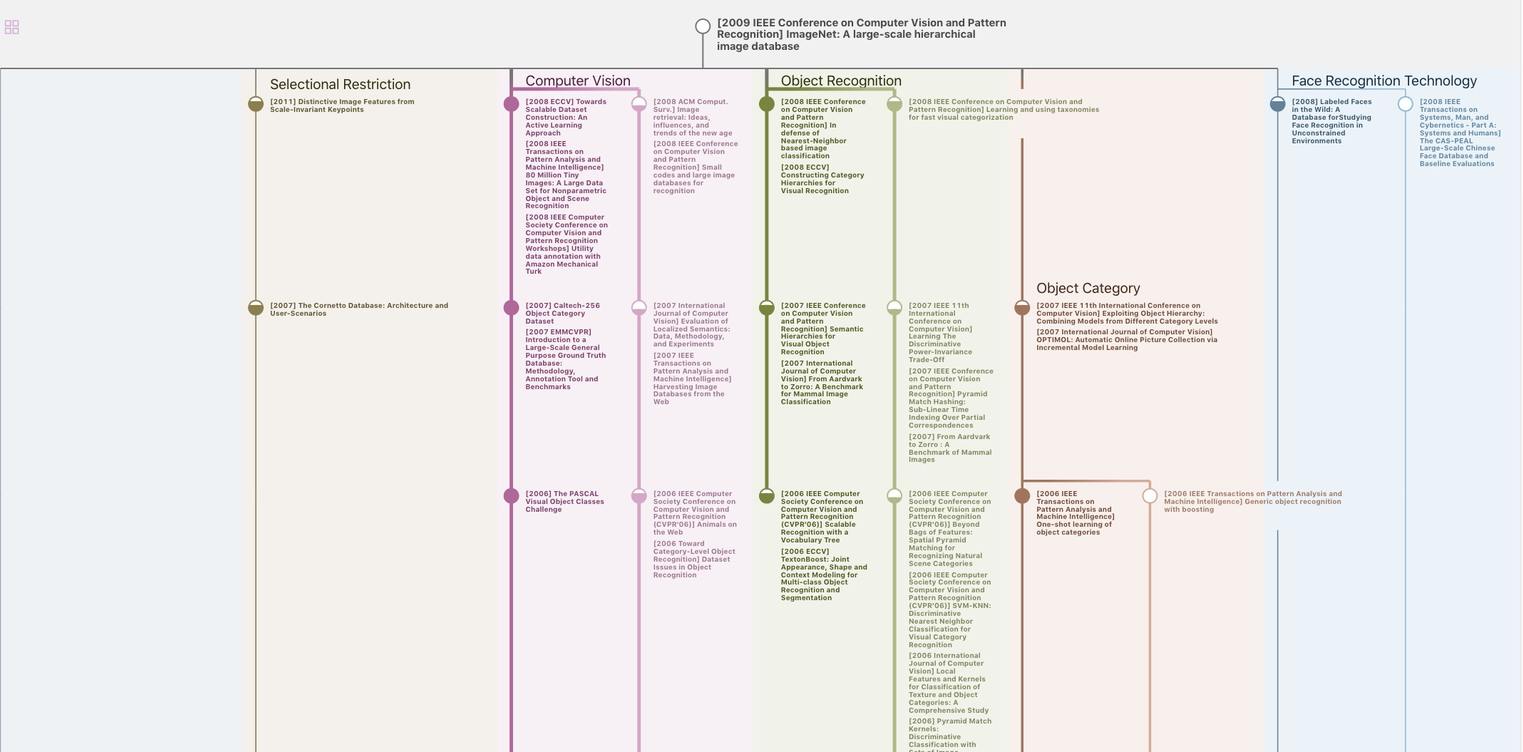
生成溯源树,研究论文发展脉络
Chat Paper
正在生成论文摘要