Learning Pneumatic Non-Prehensile Manipulation With a Mobile Blower
IEEE ROBOTICS AND AUTOMATION LETTERS(2022)
摘要
We investigate pneumatic non-prehensile manipulation (i.e., blowing) as a means of efficiently moving scattered objects into a target receptacle. Due to the chaotic nature of aerodynamic forces, a blowing controller must i) continually adapt to unexpected changes from its actions, ii) maintain fine-grained control, since the slightest misstep can result in large unintended consequences (e.g., scatter objects already in a pile), and iii) infer long-range plans (e.g., move the robot to strategic blowing locations). We tackle these challenges in the context of deep reinforcement learning, introducing a multi-frequency version of the spatial action maps framework. This allows for efficient learning of vision-based policies that effectively combine high-level planning and low-level closed-loop control for dynamic mobile manipulation. Experiments show that our system learns efficient behaviors for the task, demonstrating in particular that blowing achieves better downstream performance than pushing, and that our policies improve performance over baselines. Moreover, we show that our system naturally encourages emergent specialization between the different subpolicies spanning low-level fine-grained control and high-level planning. On a real mobile robot equipped with a miniature air blower, we show that our simulation-trained policies transfer well to a real environment and can generalize to novel objects.
更多查看译文
关键词
Deep learning methods, reinforcement learning, mobile manipulation
AI 理解论文
溯源树
样例
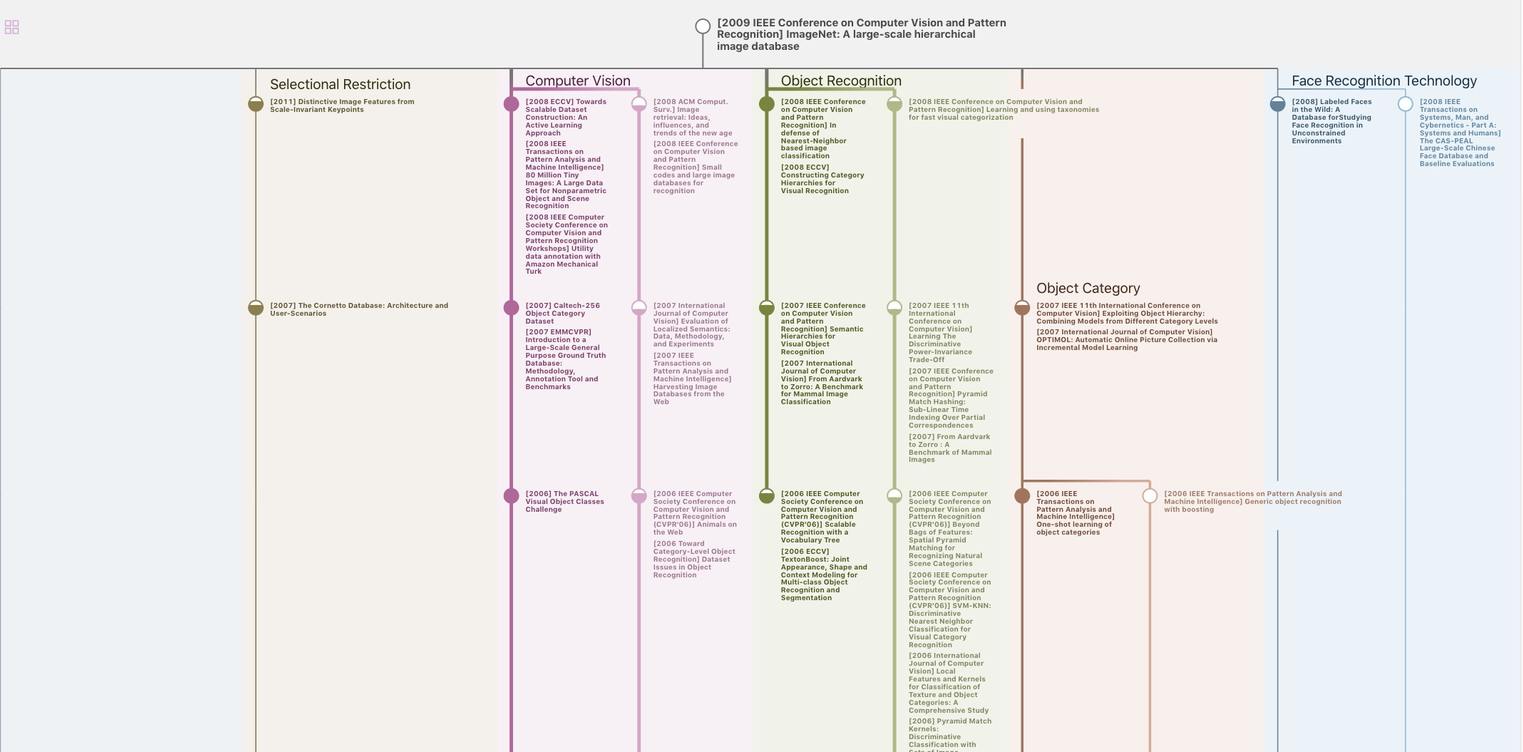
生成溯源树,研究论文发展脉络
Chat Paper
正在生成论文摘要