LiftPool: Lifting-based Graph Pooling for Hierarchical Graph Representation Learning
arxiv(2022)
摘要
Graph pooling has been increasingly considered for graph neural networks (GNNs) to facilitate hierarchical graph representation learning. Existing graph pooling methods commonly consist of two stages, i.e., selecting the top-ranked nodes and removing the rest nodes to construct a coarsened graph representation. However, local structural information of the removed nodes would be inevitably dropped in these methods, due to the inherent coupling of nodes (location) and their features (signals). In this paper, we propose an enhanced three-stage method via lifting, named LiftPool, to improve hierarchical graph representation by maximally preserving the local structural information in graph pooling. LiftPool introduces an additional stage of graph lifting before graph coarsening to preserve the local information of the removed nodes and decouple the processes of node removing and feature reduction. Specifically, for each node to be removed, its local information is obtained by subtracting the global information aggregated from its neighboring preserved nodes. Subsequently, this local information is aligned and propagated to the preserved nodes to alleviate information loss in graph coarsening. Furthermore, we demonstrate that the proposed LiftPool is localized and permutation-invariant. The proposed graph lifting structure is general to be integrated with existing downsampling-based graph pooling methods. Evaluations on benchmark graph datasets show that LiftPool substantially outperforms the state-of-the-art graph pooling methods in the task of graph classification.
更多查看译文
关键词
graph pooling,hierarchical graph,representation,lifting-based
AI 理解论文
溯源树
样例
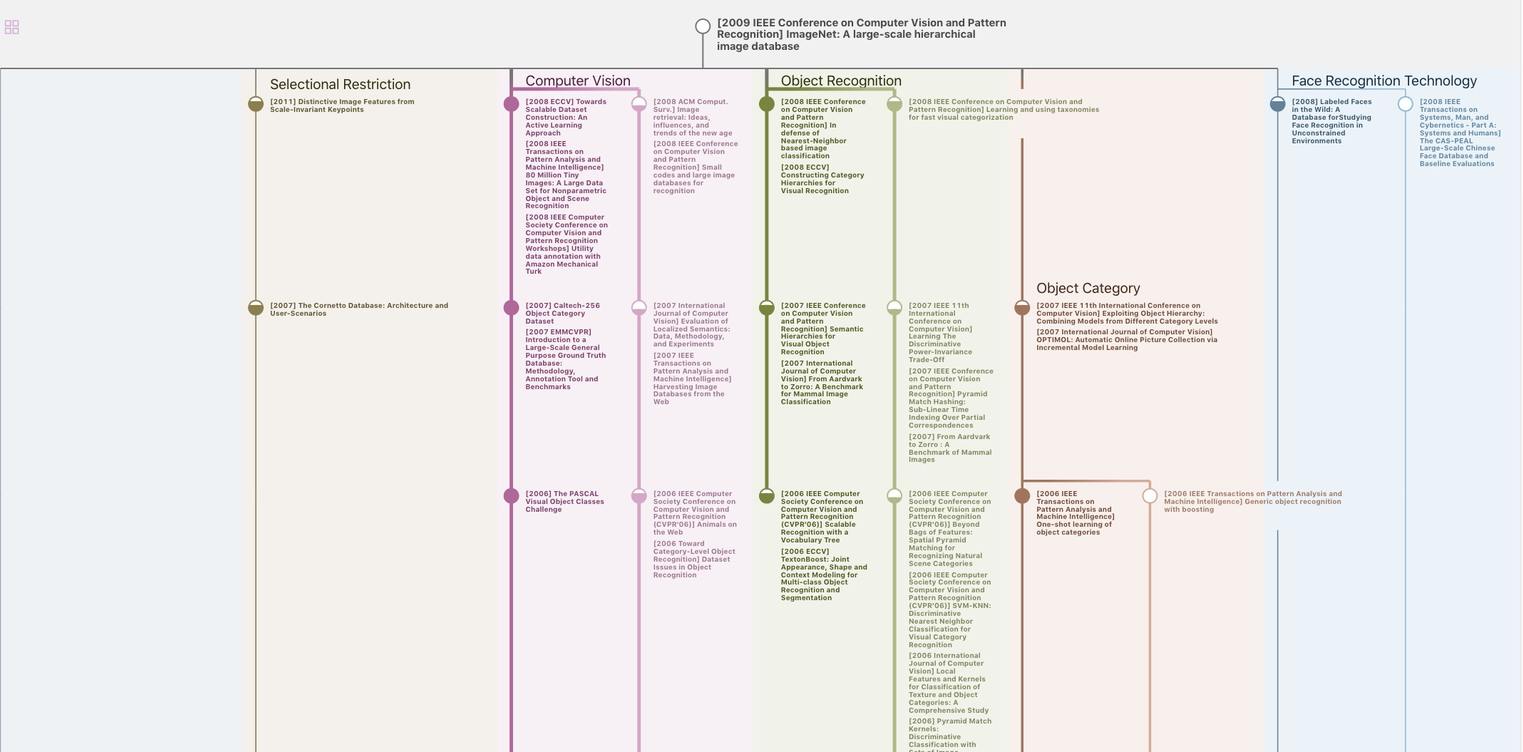
生成溯源树,研究论文发展脉络
Chat Paper
正在生成论文摘要